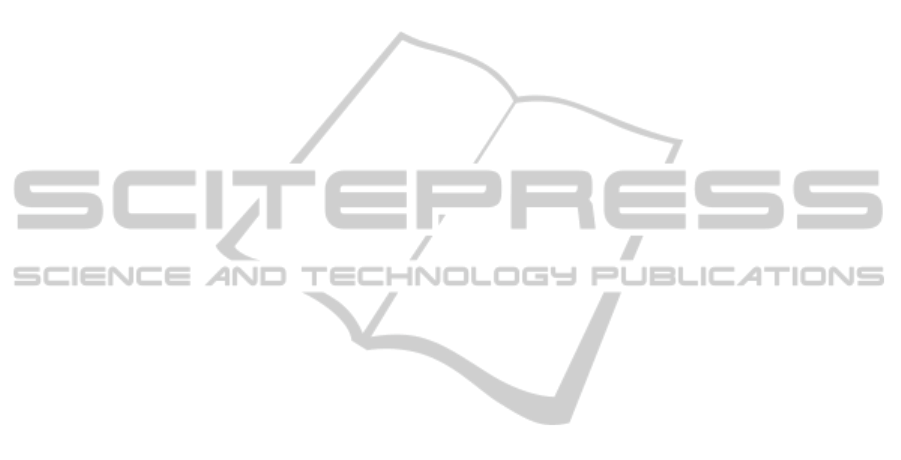
and La Ni
˜
na events. Besides, the tri-plots experiments
tell us what kind of features we probably should have
them included for the classification or for typhoon
databases. Until now, the world typhoon databases
just store the low level features of typhoons. We be-
lieve that adding the high level winds or the consid-
eration of topographical effect should be included in
typhoon databases. On the other hand, the Markov
chain-based model gives us better performance than
the tri-plots. However, the different probability dis-
tributions should be considered in the future, that is,
Markov chain-based model still has more potential to
do the following classification researches. Finally, ei-
ther tri-plots or different Markov chain models, can
be extended to other traditional Meteorological data
analysis. Because the quantity of the Meteorologi-
cal is very large; for example, the space resolution
of NCEP reanalysis data is about 144×73 in hori-
zontal; the 17 layers in vertical for one specific fea-
ture and the time resolution is about 4 times in one
day. So, when we extend our methods to analyze the
annual events, we need to modify our current algo-
rithm. First, we need an alternative method to solve
the eigenproblem in Isomap (solving large and sparse
matrix), and we need more efficiency box-counting
data structure in tri-plots.
REFERENCES
Belussi, A. and Faloutsos., C. (1995). Estimating the se-
lectivity of spatial queries using the correlation fractal
dimension. pages 299–310. In the 21-th conference of
Very Large Data Bases.
Bishop, C. M. (2006). Pattern recognition and machine
learning. Springer press.
Camargo, S, J., Robertson, A. W., Gaffney, S. J., and Smyth,
P. (2007a). Cluster analysis of typhoon tracks. Part
I: general properties. Journal of Climate, 20:3635–
3653.
Camargo, S, J., Robertson, A. W., Gaffney, S. J., and Smyth,
P. (2007b). Cluster analysis of typhoon tracks. Part
II: large circulation and ENSO. Journal of Climate,
20:3654–3676.
Chan, J. C. L. and Gray., W. M. (1982). Tropical cy-
clone movement and surrounding flow relationships.
Monthly Weather Review, 100:1354–1374.
Emanuel, K. (2005). Divine wind: the history and science
of hurricanes. Oxford university press.
Gaffney, S. and Smyth., P. (1999). Trajectory clustering
with mixtures of regression models. pages 63–72. In
the International Conference on Knowledge Discov-
ery and Data Mining.
Harr, P. A. and Elsberry, R. L. (1991). Tropical cy-
clone track characteristics as a function of large-
scale circulation anomalies. Monthly Weather Review,
119:1448–1468.
Hsieh, W. (2009). Machine learning methods in the envi-
ronmental sciences. Cambridge university press.
Jordan, M. I. An introduction to probabilistic graphic mod-
els. In preparation.
Lee, J.-G., Han, J., and Whang., K.-Y. (2007). Trajectory
clustering: A partition-and-group framework. pages
593–640. International Conference on Management of
Data.
Lee, Y.-J. and Mangasarian., O. L. (2001). SSVM:
A smooth support vector machine for classification.
Comput. Optim. Appl., 20(1):5–22.
Lin, H. (2009). Trajectory based on behavior analysis for
verification and recognition. Master’s thesis, National
Taiwan University of Science and Technology, master
thesis, Taipei.
Miller, J., Weichman, P. B., and Cross, M. C. (1992). Statis-
tical mechanics, eulers equation, and jupiters red spot.
Physics Review, A45:2328–2359.
Risi, C. (2004). Statistical synthesis of tropical cyclone
tracks in a risk evaluation perspective. Technical re-
port, Massachusetts Institute of Technology, intern-
ship report, Cambridge.
Strogatz, S. H. (2001). Nonlinear dynamics and chaos: with
applications to Physics, Biology, Chemistry, and engi-
neering. Westview press.
Tenebaum, J. B., d. Silva, V., and Langford., J. C. (2000). A
global geometric framework for nonlinear dimension-
ality reduction. Science, 290:2319–2323.
Traina, A., Traina, C., Papadimitriou, S., and Faloutsos, C.
(2001). Tri-plots: Scalable tools for multi dimensional
data. pages 184–193. In the International Conference
on Knowledge Discovery and Data Mining.
Vlachos, M., Gunopulos, D., and Kollios, G. (2002). Dis-
covering similar multidimensional trajectories. pages
673–684. In the International Conference on Data En-
gineering.
Wang, B., Rui, H., and Chan, J. C. L. (2002). How strong
ENSO events affect tropical activity over the western
north pacific. Journal of Climate, 15:1643–1658.
Webster, P. J., Holland, G. J., Curry, J. A., and Chang., H.-
R. (2005). Changes in tropical cyclone number, dura-
tion, and intensity in a warming environment. Science,
309:1844–1846.
World Meteorological Organization (2009). El Ni
˜
no/La
Ni
˜
na update. Technical report, World Meteorological
Organization.
THE TYPHOON TRACK CLASSIFICATION USING TRI-PLOTS AND MARKOV CHAIN
369