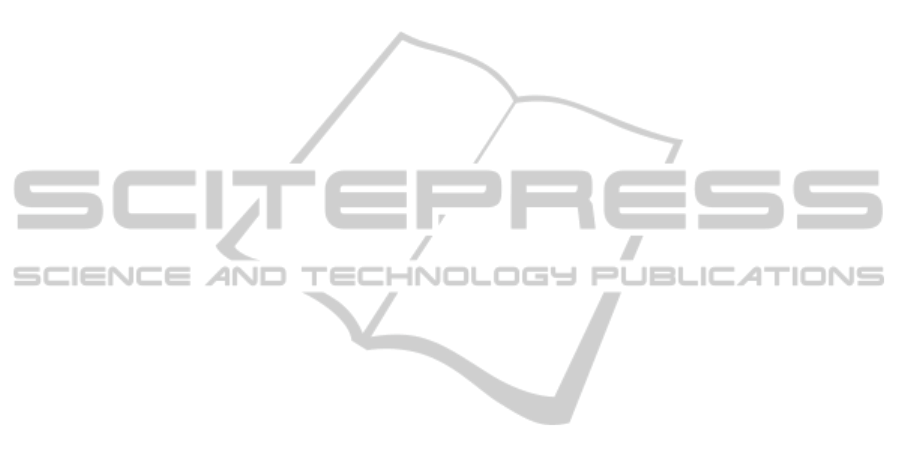
1. Both M
A
and M
B
belong to one 4ft-Discoverer
4 f tD
T
.
2. M
A
belongs to 4ft-Discoverer 4 ftD
T
A
and M
B
be-
longs to 4ft-Discoverer 4 f tD
T
B
where T
A
6= T
B
.
There is a research effort to solve problem of compar-
ison of M
A
and M
B
for both possibilities. However
its description is out of the scope of this paper.
6 CONCLUSIONS
Logic of discovery was introduced in (H
´
ajek and
Havr
´
anek, 1978) and modified in (Rauch, 2010). The
modification resulted into a system 4ft-Discoverer
4 f tD
T
which is a framework for mining associa-
tion rules and application of domain knowledge in
the mining process. We have briefly introduced the
4ft-Discoverer 4 f tD
T
and then we have shown that it
can be enhanced for needs of the SEWEBAR project
which aims to disseminating results of data mining in
the form of analytical reports answering reasonable
analytical questions.
We have identified several research problems re-
lated to this enhancement and outlined possibilities
of their solution. Further work concerns solution of
these problems.
ACKNOWLEDGEMENTS
This paper was prepared with the support of Institu-
tional funds for support of a long-term development
of science and research at the Faculty of Informat-
ics and Statistics of The University of Economics,
Prague.
REFERENCES
Agrawal, R., Imielinski, T., and Swami, A. (1993). Min-
ing association rules between sets of items in large
databases. In 19 ACM SIGMOD Conf. on the Man-
agement of Data, Washington,DC.
H
´
ajek, P. and Havr
´
anek, T. (1978). Mechanizing Hypothesis
Formation (Mathematical Foundations for a General
Theory. Springer–Verlag, Berlin Heidellberg New
York, 1st edition.
H
´
ajek, P., Havr
´
anek, T., and Chytil, M. (1983). The GUHA
Method (in Czech). Academia, Praha, 1st edition.
H
´
ajek, P., Hole
ˇ
na, M., and Rauch, J. (2010). The guha
method and its meaning for data mining. Journal of
Computer and System Science, 76(1):34–48.
Kliegr, T., Ralbovsk
´
y, M., Sv
´
atek, V., Simunek, M.,
Jirkovsk
´
y, V., Nemrava, J., and Zem
´
anek, J. (2009).
Semantic analytical reports: A framework for post-
processing data mining results. In Rauch, J., Ras,
Z. W., Berka, P., and Elomaa, T., editors, ISMIS,
volume 5722 of Lecture Notes in Computer Science,
pages 88–98. Springer.
Rauch, J. (1997). Logical calculi for knowledge discovery
in databases. In Komorowski, J. and Zytkow, J., edi-
tors, Proceedings of the 1st European Symposium on
Principles of Data Mining and Knowledge Discovery,
volume 1263 of LNAI, pages 47–57, Berlin. Springer.
Rauch, J. (2005). Logic of association rules. Applied Intel-
ligence, 22(1):9–28.
Rauch, J. (2007). Project SEWEBAR considerations on se-
mantic web and data mining. In IICAI, pages 1763–
1782.
Rauch, J. (2008). Classes of association rules: An overview.
In Lin, T. Y., Xie, Y., Wasilewska, A., and Liau, C.-
J., editors, Data Mining: Foundations and Practice,
volume 118 of Studies in Computational Intelligence,
pages 315–337. Springer.
Rauch, J. (2009). Considerations on logical calculi for deal-
ing with knowledge in data mining. In W., R. Z. and
Dardzinska, A., editors, Advances in Data Manage-
ment, volume 118 of Studies in Computational Intel-
ligence, pages 177–199. Springer.
Rauch, J. (2010). Considerations on logic of discovery and
data mining. In Suggested for publication at CLA
2010.
Rauch, J. and
ˇ
Sim
˚
unek, M. (2005). An alternative approach
to mining association rules. In Lin, T. Y., Ohsuga, S.,
Liau, C.-J., Hu, X., and Tsumoto, S., editors, Founda-
tions of Data Mining and knowledge Discovery, vol-
ume 6 of Studies in Computational Intelligence, pages
211–231. Springer.
Rauch, J. and
ˇ
Sim
˚
unek, M. (2007). Semantic web presen-
tation of analytical reports from data mining - prelim-
inary considerations. In Web Intelligence, pages 3–7.
IEEE Computer Society.
Rauch, J. and
ˇ
Sim
˚
unek, M. (2009). Dealing with back-
ground knowledge in the sewebar project. In Berendt,
B., Mladeni
ˇ
c, D., de Gemmis, M., Semeraro, G.,
Spiliopoulou, M., Stumme, G., Sv
´
atek, V., and
ˇ
Zelezn
´
y, F., editors, Knowledge Discovery Enhanced
with Semantic and Social Information, volume 220 of
Studies in Computational Intelligence, pages 89–106.
Springer.
Suzuki, E. (2004). Discovering interesting exception rules
with rule pair. In In J. Fuernkranz (Ed.), Proceedings
of the ECML/PKDD Workshop on Advances in Induc-
tive Rule Learning, pages 163–178.
LOGIC OF DISCOVERY, DATA MINING AND SEMANTIC WEB - Position Paper
351