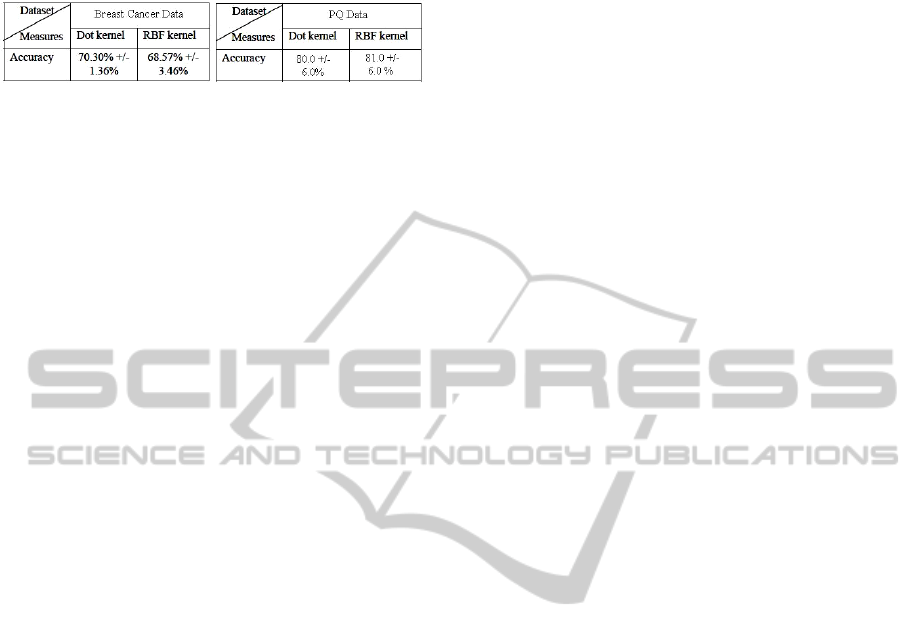
Table 1: Benchmark Data and Proposed Method Results
for SVM (Sivakumari, Praveena & Amudha 2009).
5 DISCUSSION
AND CONCLUSIONS
The aim of the proposed method is revealing the
patterns in the event data by enabling magnitude
independent event comparisons according to the
event rms values, time and duration shifts in order to
detect type similarities. The current focus on the PQ
mining is restricted on small parameters and lack of
cause and effect analysis view. The methods are
based on just examining limited PQ parameter
values for a limited time period and describe the
overall view at that period. By means of the
proposed method, long period event data
examination is made possible. The method focuses
on the magnitude independent patterns in the data by
combining the appropriate instance-based learning
algorithm results, expert knowledge and SVM
modeling. Chunk-based application of the
algorithms makes system able to handle big amount
of data, however the chunk-based structure makes
the methodology not deterministic compared to the
original versions of the selected clustering and
classification algorithms.
Although the PQ event data is time series,
throughout this paper the time component of the
events are just considered as the occurring time.
However, a complete analysis of the system should
reveal the time series based characteristics and
behavior of the system together with examining the
spatial characteristics of the system.
ACKNOWLEDGEMENTS
This research and technology development work is
carried out as a subproject of the National Power
Quality Project of Turkey. Authors would like to
thank the Public Research Support Group
(KAMAG) of the TÜBİTAK for full financial
support of the project.
REFERENCES
A. Asheibi, D. Stirling, D. Soetanto, “Analyzing
Harmonic Monitoring Data Using Data Mining”, 5th
Australian Data Mining Conference, AusDM, 2006.
A. Asheibi, D. Stirling, D. Robinson, “Identification of
Load Power Quality Characteristics Using Data
Mining”, IEEE CCECE/CCGEI, 2006.
Autoclass, viewed 5 March 2010, http://ti.arc.nasa.gov/
project/autoclass/.
B. Biswal, P. K. dash, J. B. V. Reddy, “Power Signal
Classification Using Dynamic Wavelet Network”,
Applied Soft Computing, vol. 9, pp. 118-125, 2009.
G.S. Hu, F.F. Zhu, Z. Ren, “Power quality disturbance
identification using wavelet packet entropy and
weighted support vector machines”, Expert Systems
with Applications, vol. 35, pp. 143-149, 2008.
M. Wang, G. I. Rowe, A. V. Mamishev, “Classification of
Power Quality Events Using Optimal Time-Frequency
Representations – Part 2: Application”, IEEE Trans.
on Power Delivery, vol. 19, no. 3, July 2003.
M. Uyar, S. Yildirim, M. T. Gencoglu, “An expert system
based on S-transform and neural network for
automatic classification of power quality
disturbances”, Expert Systems with Applications,
Elsevier, 2008.
Jolliffe, I. T., 2002. Principal Component Analysis.
Second ed. Springer Series in Statistics. New York:
Springer-Verlag New York.
O. N. Gerek, D. G. Ece, A. Barkana, “Covariance
Analysis of Voltage Waveform Signature for Power-
Quality Event Classification”, IEEE Trans. on Power
Delivery, vol. 21, no. 4, Oct. 2006.
P. K. Dash, I. L.W. Chun, M. V. Chilukuri, “Power
Quality Data Mining Using Soft Computing and
Wavelet Transform”, Conference on Convergent
Technologies for Asia-Pacific Region, TENCON
2003.
Snob, viewed 2 March 2010 http://
www.datamining.monash.edu.au/software/snob/.
S. Sivakumari, R. Praveena Priyadarsini, P. Amudha,
“Performance evaluation of SVM Kernels Using
Hybrid PSO-SVM”, ICGST-AIML Journal, ISSN:
1687-4846, Volume 9, Issue I, February 2009.
Y. Liao, J. B. Lee, “A fuzzy expert system for classifying
power quality disturbances”, Electrical Power and
Energy Systems, vol. 26, pp. 199-205, 2004.
INTEGRATED INSTANCE-BASED AND KERNEL METHODS FOR POWER QUALITY KNOWLEDGE MODELING
357