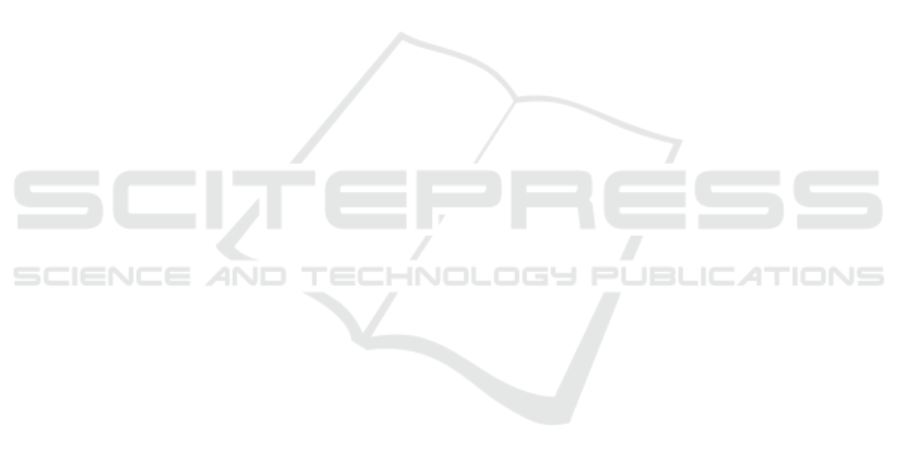
Automatic Feature Selection for Operational Scenarios
of Satellite Image Understanding using Measures
of Mutual Information
Dragos Bratasanu
1
, Ion Nedelcu
1
and Mihai Datcu
2
1
Romanian Space Agency ROSA, Mendeleev 21-25, 010362, Bucharest, Romania
2
DLR German Aerospace Center, Oberpfaffenhoffen D-82234 Wessling, Germany
Abstract. The Earth Observation processing tools operating in the recent scena-
rio need to be tailored to the new products offered by the sub-meter spatial reso-
lution imaging sensors. The new methods should provide the image analysts the
essential automatic support to discover relevant information and identify signif-
icant elements in the image. We advocate an automatic technique to select the
optimum number features used in classification, object detection and analysis of
optical satellite images. Using measures of mutual information between the tar-
get classes and the available features, we investigate the criterions of maxi-
mum-relevance and maximum-relevance-minimumredundancy for automatic
feature selection at very-low cost. Following a comprehensive set of experi-
ments on multiple sensors, applications and classifiers, the results demonstrate
the possible operational use of the method in future scenarios of human-
machine interactions in support of Earth Observation technologies.
1 Introduction
The methods and ways users operate the Earth Observation (EO) satellite data in the
present scenario are beginning to change the paradigms of classical image analysis. If
in the past the existent automatic classification and segmentation tools provided good
results for mapping of decameters resolution images, nowadays these tools fail to
offer the users the necessary support in discovering relevant information in the image.
The old methods for knowledge-based image understanding were operating on
two distinct levels: pixel level (e.g. classification techniques in which each pixel is
assigned with a label) and region level (e.g. segmentation techniques in which homo-
geneous image regions are assigned with labels). The resolution of new optical sen-
sors has reached values of centimeters (e.g. GeoEye-1 0.41m, Quickbird 0.6m and
World-View-2 0.50m) and outran the capabilities of standard information mining tools
to infer knowledge using spectral and spatial information. Future tools need to look at
the way analysts understand the data and how the current manual operations are per-
formed. We introduce an approach based on patch-level analysis, capturing contextual
information – sub-meter resolution image areas interconnect complex structures cov-
ering many pixels with high diversity of spectral information. By using this approach
we line up to the way users create cartographic products for multiple applications (e.g.
Bratasanu D., Nedelcu I. and Datcu M..
Automatic Feature Selection for Operational Scenarios of Satellite Image Understanding using Measures of Mutual Information.
DOI: 10.5220/0003143101090117
In Proceedings of the International Workshop on Semantic Sensor Web (SSW-2010), pages 109-117
ISBN: 978-989-8425-33-1
Copyright
c
2010 SCITEPRESS (Science and Technology Publications, Lda.)