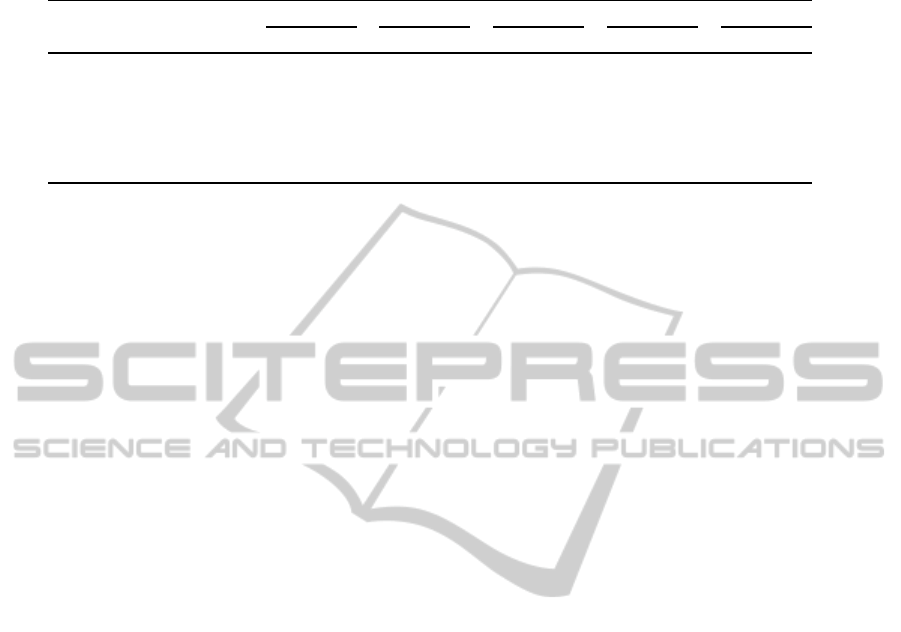
Table 4: Comparison with previous works on disulfide connectivity prediction. These results were generated on a benchmark
dataset called SP39 (Fariselli and Casadio, 2001).
Proposed Method
B = 2 B = 3 B = 4 B = 5 Overall
Q
c
Q
p
Q
c
Q
p
Q
c
Q
p
Q
c
Q
p
Q
c
Q
p
R
p
33 33 20 7 14 1 11 0 19 10
Fariselli et al. (2002) 68 68 37 22 37 20 26 2 42 34
Vullo and Frasconi. (2004) 73 73 51 41 37 24 30 13 49 44
Baldi et al. (2005) 74 74 61 51 44 27 41 11 56 49
ELM 72 72 45 33 49 31 44 22 52 45
and that the trained neural system well compares with
the state of the art methods.
ACKNOWLEDGEMENTS
The authors would like to acknowledge Dr. Dianhui
Wang, from the Department of Computer Science and
Computer Engineering at La Trobe University in Aus-
tralia, for his valuable suggestions to utilize ELMs for
the problem at hand.
REFERENCES
Altschul, S. F., Gish, W., Miller, W., Myers, E. W., and
Lipman, D. J. (1990). Basic local alignment search
tool. Journal of Molecular Biology, pages 403–410.
Altschul, S. F., Madden, T. L., Schaffer, A. A., Zhang,
J., Zhang, Z., Miller, W., and Lipman, D. J. (1997).
Gapped blast and psi-blast: a new generation of pro-
tein database search programs. Nucleic Acids Re-
search, 25(17):3389–3402.
Baldi, P., Cheng, J., and Vullo, A. (2005). Large-scale pre-
diction of disulphide bond connectivity. In Saul, L.,
Weiss, Y., and Bottou, L., editors, Advances in Neural
Information Processing Systems, 17, pages 97–104.
MA MIT Press.
Berman, H. M., Westbrook, J., Feng, Z., Gilliland, G., Bhat,
T. N., Weissig, H., Shindyalov, L. N., and Bourne,
P. E. (2000). The protein data bank. Nucleic Acids
Research, 28(1).
Chen, G., Deng, H., Gui, Y., Pan, Y., and Wang, X. (2006).
Cysteine separations profiles on protein secondary
structure infer disulfide connectivity. In IEEE Inter-
national Conference on Granular Computing.
Chen, Y., Lin, Y.-S., Lin, C.-J., and Hwang, J.-K. (2004).
Prediction of the bonding states of cysteines using
the support vector machines based on multiple fea-
ture vectors and cysteine state sequences. Proteins,
55:1036–1042.
Fariselli, P. and Casadio, R. (2001). Prediction of disulfide
connectivity in proteins. Bioinformatics, 17(10):957–
964.
Fariselli, P., Martelli, P. L., and Casadio, R. (2002). A
neural network-based method for predicting the disul-
fide connectivity in proteins. In Damiani, E., edi-
tor, Knowledge Based Intelligent Information Engi-
neering Systems and Allied Technologies (KES), pages
464–468. Amsterdam IOS Press.
Fariselli, P., Riccobelli, P., and Casadio, R. (1999). Role of
evolutionary information in predicting the disulfide-
bonding state of cysteine in proteins. Proteins,
36:340–346.
Ferr`e, F. and Clote, P. (2005). Disulfide connectivity
prediction using secondary structure information and
diresidue frequencies. Bioinformatics, 21(10):2336–
2346.
Fiser, A., Cserzo, M., Tudos, E., and Simon, I. (1992). Dif-
ferent sequence environments of cysteines and half
cystines in proteins: Application to predict disulfide
forming residues. FEBS, 302(2):117–120.
Fiser, A. and Simon, I. (2000). Predicting the oxidation state
of cysteines by multiple sequence alignment. Bioin-
formatics, 16(3):251–256.
Gabow, H. N. (1975). An efficient implementation of ed-
monds al- gorithm for maximum weight matching on
graphs. Technical Report CU-CS-075-75, Department
of Computer Science, Colorado University.
Gasteiger, E., Hoogland, C., Gattiker, A., Duvaud, S.,
Wilkins, M. R., Appel, R. D., and Bairoch, A. (2005).
Protein identification and analysis tools on the expasy
server. In Walker, J. M., editor, The Proteomics Pro-
tocols Handbook, pages 571–607. Humana Press.
Huang, G.-B. (2003). Learning capability and storage
capacity of two-hidden-layer feedforward networks.
IEEE Transaction on Neural Networks, 14(2).
Huang, G.-B. and Siew, C.-K. (2004). Extreme learning
machine: Rbf network case. In he Proceedings of the
Eighth International Conference on Control, Automa-
tion, Robotics and Vision (ICARCV).
Huang, G.-B. and Siew, C.-K. (2005). Extreme learning
machine with randomly assigned rbf kernels. Interna-
tional Journal of Information Technology, 11(1):16–
24.
Huang, G.-B., Zhu, Q.-Y., and Siew, C.-K. (2004). Extreme
learning machine: A new learning scheme of feedfor-
ward neural networks. In Proceedings of International
Joint Conference on Neural Networks (IJCNN).
DISULFIDE CONNECTIVITY PREDICTION WITH EXTREME LEARNING MACHINES
13