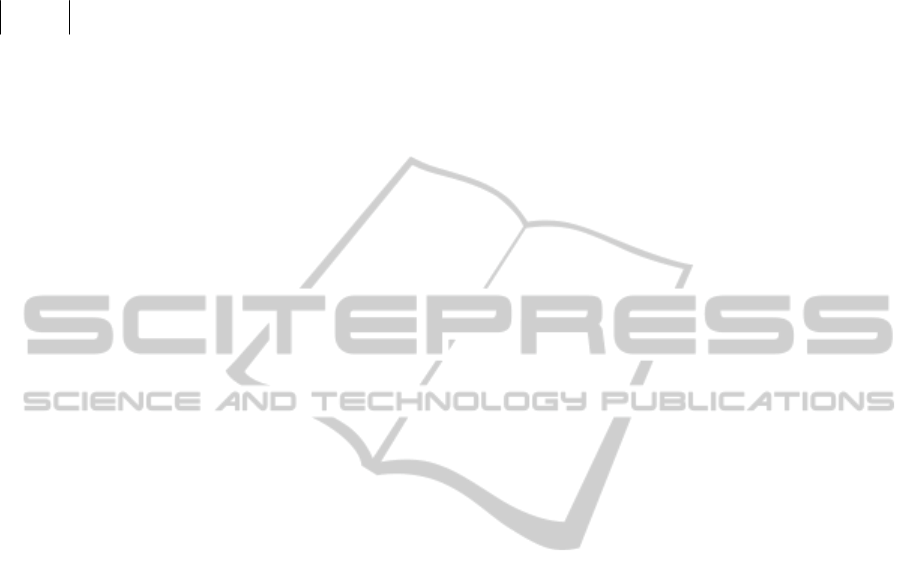
The indifference threshold is
0.01
ε
=
for any
agent. Agents speak in turns according to the order
induced by the 200 permutations on condition they
have a clear opinion: an agent
j
a
can speak if
'
jj
aa
cc
ε
−≥
.
For a same initial convictions vector it can be
observed that for each function
B
, the outcome of
the debate may depend on the order the agents
intervene. This type of situation can be interpreted as
weakly marked preferential contexts where any
perturbation can change the debate’s outcome. From
this point of view, influence function
B
is a
disturbance function in this dynamical model of a
debate. As a consequence, simulations allow
checking that the order the agents intervene in the
debate and their influence are decisive variables with
regard to the convergence of conviction state
equations.
The social influence of an agent may thus be
considered as a disturbance in the deliberation
process except if it is relevantly used by the debate
manager to govern the discussion. Indeed, in this
later case, social influence can be envisaged as an
actuator that enables controlling the outcome of the
debate or at least accelerating its convergency. For
example, when the outcome of the debate is quasi
certain (the bar is almost completely light or dark
grey), then the simplest control could consist in
choosing the order the agents intervene that
minimizes the maximal number of rounds. More
complex control can be clearly envisaged but the
aim of this paper was merely to propose a dynamical
model of the debate in a framework close to control
theory representations, then control techniques
should be naturally implemented in the future.
5.2 Debate as a Decision Making
Process
This part presents a potential application of the
presented dynamical model. The aim is to use it as
a vote system. More precisely, in this example, both
alternatives
1− and 1+ are not considered to be
equivalent: +1 is the right decision while -1 is
associated to an error. This situation may occur in
classification problems when the agents are
competitive classification algorithms.
The agents are expected to provide the right
answer most of the time but they usually disagree on
singular cases. A common solution is to use a voting
process to achieve a group decision. For example, let
the agents be 7 different classification algorithms
whose success rates are respectively: 0.6, 0.7, 0.8,
0.8, 0.6, 0.7 and 0.6; then, the group success rate
using normal vote is 0.86. Even when a weighted
vote is introduced, the same rate is obtained because
of the value of the Shapley–Shubik power index
(Shapley, 1953) which is equal to 1/7 for any
classification algorithms. Indeed as probability are
hardly, bigger than 50% for each classification
algorithms, for the normal vote as for the weighted
vote the chosen value is the one which is chosen by
at least four agents. This effect does not take place
in the proposed method because the least agents are
also the ones who change most easily his point of
view. More precisely, this issue can also be tackled
with our debate model with identity as
B
function,
and success rates for convictions. It is supposed that
7 competitive classification algorithms are available
and that the right solution is supposed to be
alternative +1. The initial probability of the 7
algorithms to choose the alternative +1 are: 0.6, 0.7,
0.8, 0.8, 0.6, 0.7 and 0.6. Moreover it is supposed
that 10 000 cases are studied by each agent. For each
case, the answer of the agent is inferred according to
his probability to be right ( this is one method to
model the aggregation function corresponding to the
different classification algorithm. Then, for each of
the 10000 cases, the choice with a majority vote
procedure and the collective decision achieved with
our model when convictions at the start are the
initial probabilities are both computed. The program
stops when all the classification algorithms do agree.
While simple and weighted majorities obtain the
right answer with a rate of 86 %, our method rate is
94 %.
For 7 agents, several values of probability to
make the right decision are randomly generated and
3 rates are computed:
- the rate of success of the vote,
-the rate of success the weighted vote,
- the rate of success of our debate.
The rate of the weighted vote and of our debate
according to the rate of simple vote are plotted in
figure 5. Note that the same rate for the simple vote
can be obtained with very different sets of
probabilities. That is why the rate of success of the
weighted vote is somewhat equal to the simple vote
for very particular sets of probabilities where several
agents (algorithms) are much better than the others.
The debate always gives a better rate but its
preferences change according to the profile of
involved probabilities.
ICAART 2011 - 3rd International Conference on Agents and Artificial Intelligence
38