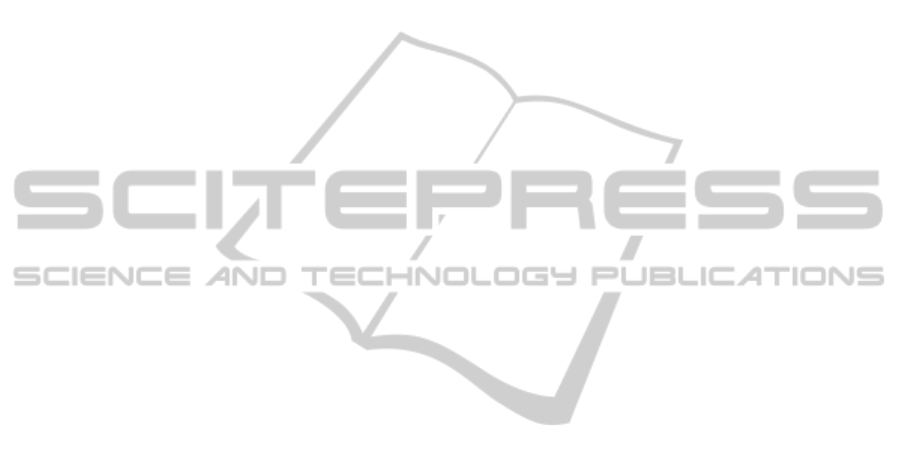
measurement between the two methods),
reproducibility (intra/inter variability given by the
coefficient of variation values or relative percentage
of agreement among the experts), accuracy (mean
segmentation error), sensitivity (True Positive Rate),
and specificity (True Negative Rate).
The most part of the studies, conclude that
automatic segmentation closely reproduce manual
contours with no significant volume difference and
with a significant time difference. The automatic
approach is faster with equivalent accuracy to
manual method. In concern with the observer’s
variability, automatic segmentation reaches
significant gains in reproducibility. This may be
attributed to the reduced user interaction required for
efficient segmentation of the organs.
In general, the studies used small samples,
except the Hass et al, and one of the studies, Neal et
al, contrary of the general outcomes, concluded that
the time taken to segment automatically the prostate
was superior compared with the manual tracing.
This fact could be explained due to the algorithm
limitations in the soft tissue segmentation.
In the automatic segmentation, the limitations
found in the studies were: underestimation of
prostate, distinguish the base of bladder from
prostate, segment the real boundary of rectum and
separation of the rectum from seminal vesicles.
All studies have emphasized the potential of the
automatic approach to improve radiotherapy
planning conditions. In contrast to manual slice
delineation, organ segmentation can be done within
a few minutes with no significant mean
segmentation error. However, some problematic
contours of soft tissues have to be corrected
interactively.
In general, many published approaches in image
segmentation are validated on a small set of test
images and few methods in the domain of automated
organ segmentation for RTP have been
quantitatively validated so far.
As future directions, it is recommended the
improvement of the segmentation algorithms in the
delineation of problematic soft tissues and future
validation studies with large scale trials.
Furthermore, a thorough systematic review
aiming at a study of meta-analysis is required to
critically access the differences between automatic
and manual segmentation, especially for prostate
cancer.
REFERENCES
Boehmer, D., Maingon, P., et al. 2006. Guidelines for
primary radiotherapy of patients with prostate cancer.
Radiotherapy and Oncology, 79, 259-269.
Bueno, G., Fisher, M., et al. 2001. Automatic
segmentation of clinical structures for RTP:
Evaluation of a morphological approach. Proceedings
of Medical Image Understanding and Analysis (MIUA
'01), 73-76.
Freedman, D., Radke, R., et al. 2005. Model-based
segmentation of medical imagery by matching
distributions. IEEE Transactions on Medical Imaging,
24, 281-292.
Haas, B., Coradi, T., et al. 2008. Automatic segmentation
of thoracic and pelvic CT images for radiotherapy
planning using implicit anatomic knowledge and
organ-specific segmentation strategies. Journal
Physics in Medicine and Biology, 53, 1751-1771.
Huyskens, D. P., Maingon, P., et al. 2009. A qualitative
and a quantitative analysis of an auto-segmentation
module for prostate cancer. Radiotherapy and
Oncology, 90, 337-345.
Li, T., Xing, L., et al. 2006. Four-dimensional cone-beam
computed tomography using an on-board imager.
Medical Physics, 33, 3825-3833.
Mazonakis, M., Damilakis, J., et al. 2001. Image
segmentation in treatment planning for prostate cancer
using the region growing technique. British Journal of
Radiology, 74, 243-248.
Mcbain, C. A., Moore, C. J., et al. 2008. Early clinical
evaluation of a novel three-dimensional structure
delineation software tool (SCULPTER) for
radiotherapy treatment planning. British Journal of
Radiology, 81, 643-652.
Neal, A. J., Sivewright, G., et al. 1994. Technical note:
Evaluation of a region growing algorithml for
segmenting pelvic computed tomography images
during radiotherapy planning. BJR, 67, 392-395.
Pekar, V., Mcnutt, T., et al. 2004. Automated model-based
organ delineation for radiotherapy planning in
prostatic region. International Journal of Radiation
Oncology Biology Physics, 60, 973-980.
Quicken, M. 2000. Generation and Application of
Statistical Shape Models for the Segmentation of
Abdominal Organs in Radiotherapy Planning. Doctor
of Technical Sciences, Swiss Federal Institute of
Technology.
AUTOMATIC ORGAN DELINEATION OF COMPUTED TOMOGRAPHY IMAGES FOR RADIOTHERAPY
PLANNING IN PROSTATE CANCER - An Overview
485