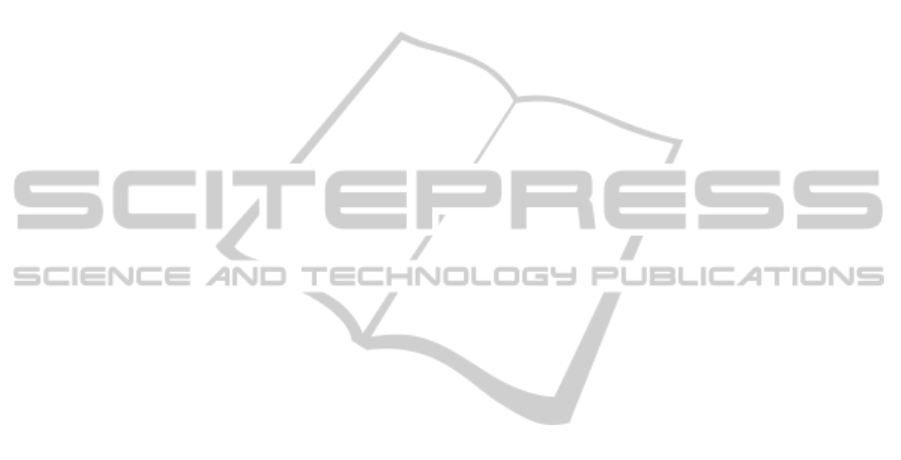
To address the issue of overfitting, we optimized
the isotopologue data for the 34-hour, 48-hour, and
72-hour time points simultaneously and calculated
an AIC for the combined optimization. The expert-
derived model had the best AIC of -428.98. The next
best model 6_G1R1A1U3_a1 was significantly
worse with an AIC of -355.87, indicating that
overall, the expert-derived model provides the best
description of the data.
4 CONCLUSIONS
We have demonstrated a novel metabolic modelling
methodology applied to FT-ICR-MS isotopologue
intensity data for UDP-GlcNAc and UDP-GalNAc.
Our implementation, GAIMS, interprets a set of
isotopologues as the flow of functional moieties
through metabolic pathways. This is represented by
a set of optimizable parameters for a given moiety
model. Figure 2 demonstrates a solid convergence of
50 individual optimizations for an expert-derived
model based on what is currently known about
UDP-GlcNAc biosynthesis. However, the standard
deviations for parameter values should not be
interpreted as a close representation of parameter
error, especially with the indication of model
overfitting in results from Table 2.
In addition, we demonstrate a robust model
selection method, which uses a form of the Akaike
information criterion (Equation 9). Our use of the
average parameter values from a set of optimizations
allows the AIC to sense smoothness of the error
surface for the target function of a given moiety
model. This application of the AIC along with the
use of isotopologues from multiple time points
enables our model selection method to overcome
issues of model overfitting for a set of isotopologues
at individual time points. We envision the coupling
of this robust model selection method with newer
non-steady-state metabolic flux analytical methods
(Selivanov et al., 2006; Wahl et al., 2008) as a
logical next step.
ACKNOWLEDGEMENTS
This work was supported in part by National Science
Foundation EPSCoR grant # EPS-0447479, NIH
NCRR Grant 5P20RR018733, 1R01CA118434-
01A2 (TWMF), 1RO1 CA101199 (TWMF)
R21CA133688-01 (ANL) from the National Cancer
Institute, DOE Grant Number DE-EM0000197
(HNBM), the Cardinal Research Cluster, the
Kentucky Challenge for Excellence, and the Brown
Foundation.
REFERENCES
Akaike, H., 1974. “A new look at the statistical model
identification”, IEEE Transactions on Automatic
Control, 19, 716–723.
Edwards, J. S., Ibarra, R. U., Palsson, B. O., 2001. “In
silico predictions of Escherichia coli metabolic
capabilities are consistent with experimental data”,
Nature Biotech, 19, 125-130.
Fell, D. A., 1984. “Fat synthesis in adipose tissue. An
examination of stoichiometric constraints”, J Biochem,
238, 781-786.
Fell, D.A., 1997. Understanding the Control of
Metabolism, London: Portland Press.
Gambetta, M. C., Oktaba, K., & Müller, J., 2009.
“Essential Role of the Glycosyltransferase Sxc/Ogt in
Polycomb Repression”, Science, 325, 93-96.
Harrigan, G. G., Goodacre, R. (Ed), 2003. Metabolic
profiling: its role in biomarker discovery and gene
function analysis, Boston: Kluwer Academic
Publishers.
Hart G. W., Housley M. P., Slawson C., 2007. "Cycling of
O-linked beta-N-acetylglucosamine on
nucleocytoplasmic proteins", Nature, 446, 1017-1022.
Hellerstein, M. K., 2003. “In vivo measurement of fluxes
through metabolic pathways: the missing link in
functional genomics and pharmaceutical research”,
Annu Rev Nutr, 23, 379-402.
Kauffman, K. J., Prakash, P., Edwards, J. S., 2003.
“Advances in flux balance analysis”, Curr Opin
Biotech, 14, 491-496.
Lane A. N., Fan T. W-M., Higashi R. M., 2008.
“Isotopomer-based metabolomic analysis by NMR and
mass spectrometry". Methods in Cell Biology,Vol 84.
Biophysical Tools for Biologists, Vol 1,ed. Correia JJ,
Detrich HW. Elsevier Science & Technology Books
Ch.18. 541-588.
Lane, A. N., Fan, T. W-M., Xie, Z., Moseley, H. N. B.,
Higashi, R. M., 2009. “Stable isotope analysis of lipid
biosynthesis by high resolution mass spectrometry and
NMR.”, Anal Chim Acta, 651, 201-208.
Moseley, H. N. B., 2010. “Correcting for the Effects of
Natural Abundance in Stable Isotope Resolved
Metabolomics Experiments Involving Ultra-High
Resolution Mass Spectrometry”, BMC Bioinformatics,
11, 139-144.
Savinell, J. M., Palsson, B. O., “Optimal selection of
metabolic fluxes for in vivo measurement. I.
Development of mathematical methods”, J Theor Biol,
155, 201-214.
Sauer, U., Lasko, D. R., Fiaux, J., Hochuli, M., Glaser, R.,
Szyperski, T., Wuthrich, K., Bailey, J. E., 1999.
“Metabolic Flux Ratio Analysis of Genetic and
Environmental Modulations of Escherichia coli
BIOINFORMATICS 2011 - International Conference on Bioinformatics Models, Methods and Algorithms
114