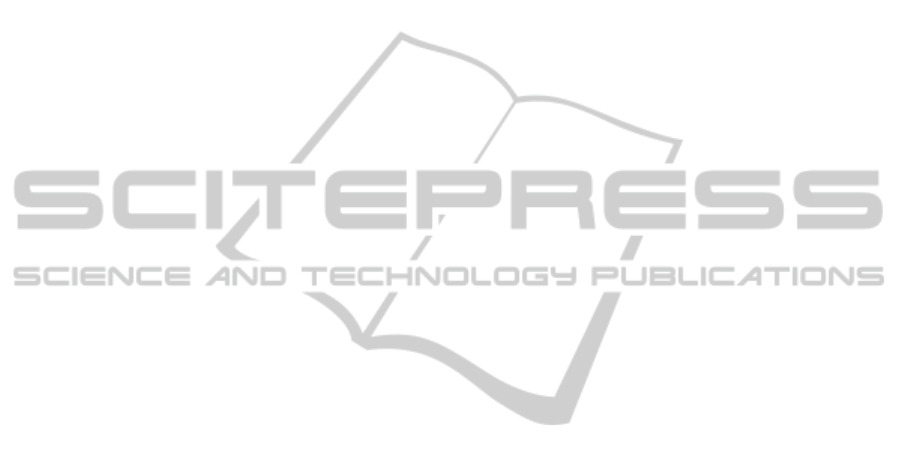
more energy efficient micro-controller that also of-
fers a lower stand-by power consumption. The func-
tion of the SleePic Watch in our experiments was to
attract attention from the users during the reaction
task (LEDs) and provide an interface for respond-
ing (button). The peripheral location of the SleePic
Watch required wireless transmission of data and an
independent energy storage. The frequent wireless
communication increases the power consumption of
the SleePic Watch and Core. In future versions it is
imaginable to replace the custom SleePic Watch with
another, already existing pervasive device such as a
smart phone or digital wristwatch.
6 CONCLUSIONS
We developed a SleePic prototype based on com-
mercially available sensors and components. Miss-
ing functionality, such as user interface and com-
putational resources for on-line classification, were
added by custom designed extension modules. A DSP
micro-controller was used to efficiently compute FFT
for extracting the frequency features of the signals and
to compute the ANN for classification. FFT and ANN
were optimized for speed and lower power consump-
tion. We used no liquid crystal display. To render the
feedback information more meaningful to the user, it
might be advantageous to integrate a liquid crystal
display in a next step into the SleePic Watch.
Although cardio-respiratory signals were mea-
sured non-invasively with a wearable belt, it was more
cumbersome to use than a wrist worn actigraphy de-
vice. It is therefore application-dependent whether
a single actigraph device or a combined system like
SleePic should be adopted. If, for example, the appli-
cation is oriented toward sleep disorder diagnosis, the
system with additional cardio-respiratory functional-
ity offer a clear advantage, because current standards
in sleep disorder diagnosis require the recording of
these signals (Patel and Davidson, 2007).
The SleePic prototype demonstrated wearable
sleep/wake classification that is less obtrusive than the
EEG monitors currently used in sleep centers. We
also showed how existing classification algorithms
can be modified in order to comply with the narrow
constraints given by wearable systems.
The SleePic prototype can be considered as a
proof-of-concept for a new generation of health and
wellness devices. It shows the feasibility of a wear-
able, on-line sleep/wake classifier using low-cost
components. The minimal processing power require-
ments of the presented system opens new doors for
the use of high-level algorithms required for context-
awareness or automated diagnostics in point-of-care
health care.
ACKNOWLEDGEMENTS
We thank all subjects for participating in the SleePic
validation study. Dr. Steffen Wischmann and Michael
Chiang provided valuable comments on previous ver-
sions of this manuscript. Adam Klaptocz and James
Roberts contributed to the PCB design. Andr
´
e
Badertscher and Peter Br
¨
uhlmeier helped with the
manufacturing and assembling of SleePic. We would
like to thank Dr. med Werner Karrer, Dr. med Thomas
Rote and Isabelle Arnold of the Luzerner H
¨
ohenklinik
Montana, Switzerland, for providing expert knowl-
edge on sleep analysis.
REFERENCES
Colten, H. and Altevogt, B. (2006). Sleep disorders and
sleep deprivation: an unmet public health problem.
National Academies Press, Washington, DC.
de Souza, L., Benedito-Silva, A., Pires, M., Poyares, D.,
Tufik, S., and Calil, H. (2003). Further validation of
actigraphy for sleep studies. Sleep, 26(1):81–5.
Karlen, W., Mattiussi, C., and Floreano, D. (2008). Improv-
ing actigraph sleep/wake classification with cardio-
respiratory signals. In Annual International Confer-
ence of the IEEE Engineering in Medicine and Biol-
ogy Society, pages 5262–5.
Karlen, W., Mattiussi, C., and Floreano, D. (2009). Sleep
and Wake Classification With ECG and Respiratory
Effort Signals. IEEE Transactions on Biomedical Cir-
cuits and Systems, 3(2):71–8.
Martin, T., Jovanov, E., and Raskovic, D. (2000). Issues
in Wearable Computing for Medical Monitoring Ap-
plications: A Case Study of a Wearable ECG Moni-
toring Device. International Symposium on Wearable
Computers ISWC 2000, Atlanta, October.
Ogilvie, R. (2001). The process of falling asleep. Sleep
Medicine Reviews, 5(3):247–70.
Patel, M. R. and Davidson, T. M. (2007). Home sleep test-
ing in the diagnosis and treatment of sleep disordered
breathing. Otolaryngologic Clinics of North America,
40(4):761–784.
Sadeh, A. (2002). The role of actigraphy in sleep medicine.
Sleep Medicine Reviews, 6(2):113–124.
Scheffler, M. and Hirt, E. (2004). Wearable devices for
emerging healthcare applications. In Proc. 26th An-
nual International Conference of the IEEE Engineer-
ing in Medicine and Biology Society IEMBS ’04, vol-
ume 2, pages 3301–3304.
SLEEPIC - Developments for a Wearable On-line Sleep and Wake Discrimination System
137