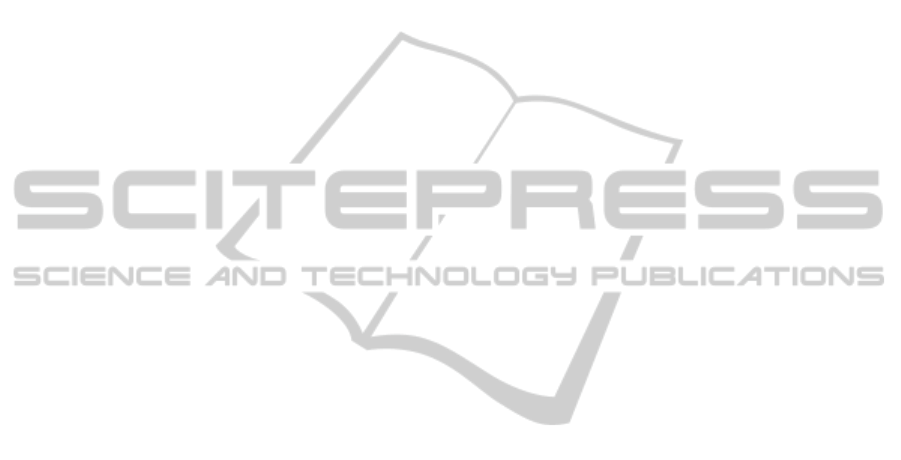
versus post-VNS and epileptic versus control
groups.
We found no significant changes in other
parameters due to the VNS, and when compared to
the control group.
4 DISCUSSION
As no remarkable difference was found between pre-
VNS and post-VNS implantation in epileptic
subjects, even when compared to the control group
for the parameters SDNN, SDANN, RMSSD, 1/f
slope, FD and SampEn, no discussion is made.
The major previous studies regarding the effects
of the VNS on HRV were performed during wake-
fulness. Setty et al. (1998) didn’t find any significant
effect of the VNS on the heart rate and heart rate
variability. This study confirmed that VNS does not
influence the heart rate in epileptic children (mean
RR interval in the Figure 1), which is significantly
higher compared to their controls. We found a
reduction of vagal tone after long-term VNS during
night-time, when autonomic control on heart rate
should be mainly sustained by vagal influence
(Pagani et al., 1997). This is however, in contrast to
the study of Kamath et al. (1992), which reported
significant increase in HF component of the power
spectrum.
LF/HF ratio after the implantation of the VNS
stimulation still remains below 1 for most of the
children with refractory epilepsy, meaning that vagal
modulation of the heart rate is still dominant. On the
group level, there are changes in cardiac
sympathovagal balance towards sympathetic
predominance in phase 2 of sleep (p=0.177) and also
during deep sleep (p=0.035) after the VNS
implantation. These findings require further
observations.
In addition, LF/HF and DFA coefficients have
similar pattern of behaviour due to the implantation
of the VNS and compared to the control group. In
other nonlinear parameters, we found no significant
changes due to the implantation of the VNS.
In conclusion, our data indicate that: (a) VNS do
not influence heart rate during phase 2 of sleep and
deep sleep; and (b) VNS affects cardiac
sympathovagal balance.
ACKNOWLEDGEMENTS
Research supported by:
Research Council KUL: GOA Ambiorics, GOA
MaNet, CoE EF/05/006 Optimization in Engineering
(OPTEC), IDO 05/010 EEG-fMRI, IDO 08/013
Autism, IOF-KP06/11 FunCopt; Flemish
Government: FWO: PhD/postdoc grants, G.0302.07
(SVM), G.0341.07 (Data fusion), G.0427.10N
(Integrated EEG-fMRI) research communities
(ICCoS, ANMMM); IWT: TBM070713-Accelero,
TBM070706-IOTA3, TBM080658-MRI (EEG-
fMRI), PhD Grants; Belgian Federal Science Policy
Office: IUAP P6/04 (DYSCO, `Dynamical systems,
control and optimization', 2007-2011); ESA
PRODEX No 90348 (sleep homeostasis); EU: FAST
(FP6-MC-RTN-035801), Neuromath (COST-
BM0601)
REFERENCES
Deng, Z. D., Poon, C. S., Arzeno, N. M., Katz, E. S.,
2006. Heart rate variability in pediatric obstructive
sleep apnea. In Ann. Int. Conf. New Jork City, USA
Kamath, M. V., Upton, A. R. M., Talalla, A., Fallen, E. L.,
1992. Neurocardiac responses to vagaoafferent
electrostimulation in humans. In Pace 15, 1581-1587
Katz, M. J., 1988. Fractals and the analysis of waveforms.
In Comput Biol Med, 18(3):145-56
Kobayashi, M., Musha, T., 1982. 1/f fluctuations of heart
beat period. In IEEE Trans Biomed Eng, 29:456-7
Pagani, M., Montano, N., Porta, A., Milliani, A., Abboud,
F. M., Birkett, C., Somers, V. K., 1997. Relationship
between spectral components of cardiovascular
variabilities and direct measures of muscle
sympathetic nerve activity in humans. In Circulation
95, 1441-1448
Peng, C. K., Havlin, S., Hausdorff, J. M., Mietus, J. M.,
Stanley, H. E., Goldberger, A. L., 1996. Fractal
mechanisms and heart rate dynamics. In J
Electrocardiol, 28(Suppl):59-64
Richman, J. S., Moorman, R. J., 2000. Physiological time-
series analysis using approximate entropy and sample
entropy. In Am J Physiol Heart Circ Physiol,
278:H2039-49
Setty, A. B., Vaughn, B. V., Quint, S. R., Robertson, K.
R., Messenheimer, J. H., 1998. Heart period variability
during vagal nerve stimulation. In Seizure
Task Force of the European Society of Cardiology and the
North American Society of Pacing and
Electrophysiology, 1996. Heart rate variability
standards of measurement, physiological
interpretation and clinical use, 71:1043-65
BIOSIGNALS 2011 - International Conference on Bio-inspired Systems and Signal Processing
412