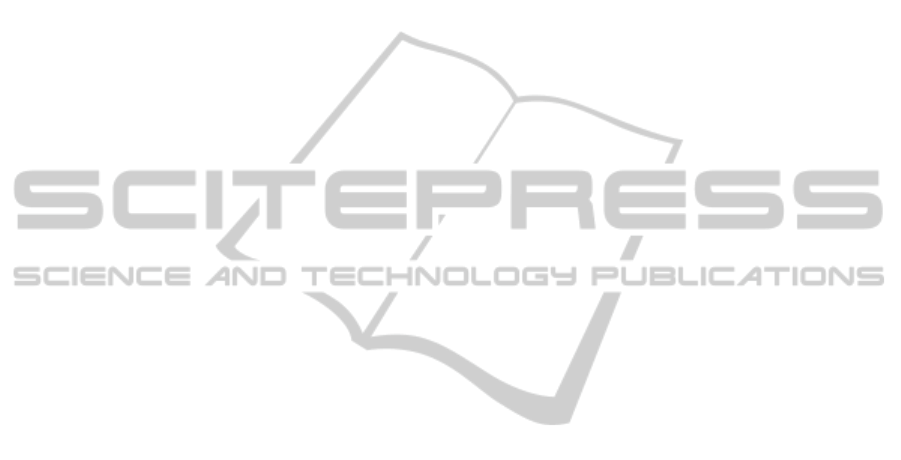
neural network topology and to learn the weights
simultaneously, outperforms the traditional
classifiers (82.5 % accuracy).
From a clinical perspective, this article illustrates
that in a general population of elderly, fall risk is
related to different underlying constructs, with clear
manifestations among different dimensions in the
gait pattern as captured by the accelerometer.
REFERENCES
Atkeson C., Moore A., and Schaal S., 1996. "Locally
weighted learning" AI Reviews.
Duda, R. O., Hart, P. E., & Stork, D. G. (2000). Pattern
Classification. Wiley-Interscience.
Giansanti, D., Maccioni, G., Cesarino, S., Benvenuti, F., &
Maccellari, V. (2008). Assessment of fall-risk by
means of a neural network based on parameters
assessed by a wearable device during posturography.
Medical Engineering and Physics, 30, 367-372.
Hausdorff, J. M., Schaafsma, J. D., Balash, Y., Bartels, a.
L., Gurevich, T., Giladi, N., et al. (2003). Impaired
regulation of stride variability in Parkinson's disease
subjects with freezing of gait. Experimental brain
research. Experimentelle Hirnforschung.
Expérimentation cérébrale, 149(2), 187-94.
Hausdorff J. M., 2005. Gait variability: methods, modeling
and meaning. Journal of NeuroEngineering and
Rehabilitation. 9:1-9.
Marschollek, M., Nemitz, G., Gietzelt, M., Wolf, K. H.,
Meyer Zu Schwabedissen, H., Haux, R., et al., 2009.
Predicting in-patient falls in a geriatric clinic: a
clinical study combining assessment data and simple
sensory gait measurements. Zeitschrift für
Gerontologie und Geriatrie : Organ der Deutschen
Gesellschaft für Gerontologie und Geriatrie, 42(4),
317-21.
Marschollek M., Wolf K., Plischke M., Haux R., 2006.
Classification of activities of daily life from long-term
realistic multi-sensor data. In: Proceedings of IEEE
Health Pervasive Systems HPS06.
Moe-Nilssen, R. (1998). Test-retest reliability of trunk
accelerometry during standing and walking *1, *2.
Archives of Physical Medicine and Rehabilitation,
79(11), 1377-1385.
Moe-Nilssen R., Helbostad J. L., 2004. Estimation of gait
cycle characteristics by trunk accelerometry. Journal
of Biomechanics. 37(1):121-126.
Moe-Nilssen, R., & Helbostad, J. L. (2005). Interstride
trunk acceleration variability but not step width
variability can differentiate between fit and frail older
adults. Gait & posture, 21(2), 164-70.
Narayanan, M. R., Redmond, S. J., Scalzi, M. E., Lord, S.
R., Celler, B. G., Lovell Ast, N. H., et al., 2010.
Longitudinal falls-risk estimation using triaxial
accelerometry. IEEE transactions on bio-medical
engineering, 57(3), 534-41.
Oshima Y., Kawaguchi K., Tanaka S., et al., 2010.
Classifying household and locomotive activities using
a triaxial accelerometer. Gait & posture. 31(3):370-4.
Platt J., 1998. "Fast Training of Support Vector Machines
using Sequential Minimal Optimization". Advances in
Kernel Methods-Support Vector Learning, B. Schoel-
kopf, C. Burges, and A. Smola, eds., MIT Press.
Quinlan R., 1993. "C4.5: Programs for Machine
Learning", Morgan Kaufmann Publishers, San Mateo.
Stanley K. O., Miikkulainen R., 2002. Evolving neural
networks through augmenting topologies. Evol
Comput 10:99–127.
Swanenburg, J., de Bruin, E. D., Uebelhart, D., & Mulder,
T. (2010). Falls prediction in elderly people: a 1-year
prospective study. Gait & posture, 31(3), 317-21.
Tan M., Hartley M., Bister M., Deklerck R., 2009.
Automated feature selection in neuroevolution.
Evolutionary Intelligence. 1(4):271-292.
Whiteson S., Stanley K. O., Miikkulainen R., 2004.
Automatic feature selection in neuroevolution. In:
Proceedings of the genetic and evolutionary
computation conference (GECCO), Seattle,
Washington, USA.
Zijlstra W., 2004. Assessment of spatio-temporal
parameters during unconstrained walking. European
journal of applied physiology
. 92(1-2):39-44.
ACCELEROMETER BASED GAIT ANALYSIS - Multi Variate Assessment of Fall Risk with FD-NEAT
143