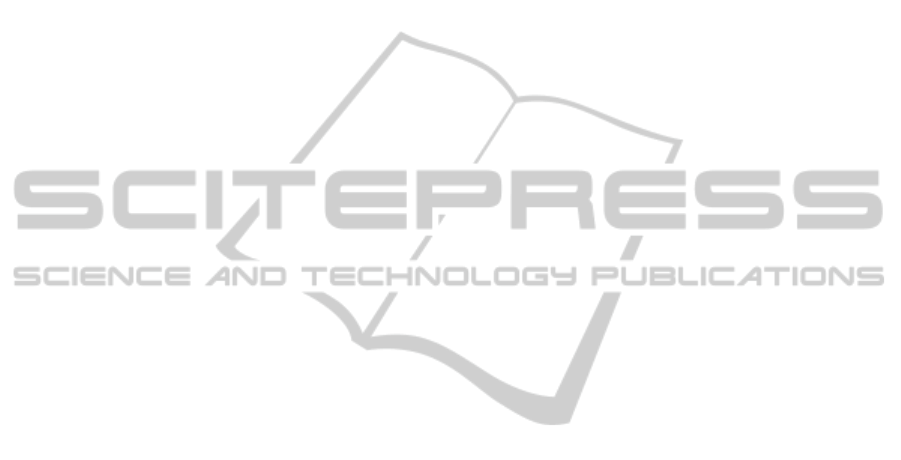
elective admissions, likely emergency inpatient
admissions and likely inpatient discharges.
2 METHODS
A literature search was performed in December 2009
with the goal of identifying studies evaluating the
use of decision support systems when applied to
hospital inpatient bed management. The search was
conducted both on PubMed and ISI Web of
Knowledge resulting in a base data collection of 65
studies. Thirteen studies were excluded by lack of
available abstract or full text and 38 studies were
excluded after abstract and/or full text review (e.g.
studies that didn’t address explicitly hospital bed
capacity and/or hospital bed allocation management
subjects). The final size of the overall data collection
was of 14 studies. Ten studies (~71,4%) addressed
the use of mathematical models to forecast and/or
plan hospital bed capacity (Green and Nguyen,
2001; Nguyen et al., 2005, 2007; Kokangul, 2008;
Mackay and Lee, 2005; Millard et al., 2000;
Cochran and Roche, 2008; Gorunescu, McClean and
Millard, 2002; Belien and Demeulemeester, 2007;
Ridge et al., 1998). Four studies (~28,6%) addressed
the use of information technologies to support
inpatient bed management, currently under use
(Blair, 2005; Szabo, 2003; Reuille, 2004) or under
evaluation (Kannry et al., 2007). One literature
source with publication date in the current decade
(National Audit Office, 2000) was handpicked and
added to the overall data collection in order to
support theoretical and practical concepts
surrounding the bed management subject.
3 FINDINGS
From the analysis of the selected data collection
from both PubMed and ISI Web of Knowledge, it
was perceived that the subject under revision (the
use of decision support systems for hospital inpatient
bed management) can be undertaken by two
distinctive approaches. One approach is based on the
use of mathematical models to support the planning
and allocation of hospital inpatient beds. Another
approach consists on the utilization of information
technologies to support the timely-decision/timely-
action of the hospital staff (administrative clerk,
clinicians and housekeepers) in order to facilitate
inpatient placement thus optimizing the bed
management process. The main conclusions and
recommendations extracted from the reviewed
studies are sketched in table 1.
The studies that addressed the planning and
allocation of inpatient beds by means of
mathematical models covered such diverse
techniques as: queuing models, stochastic models,
flow models and other general proposed models.
Different distributions of patient
arrival/department service rates were analyzed for
different department/unit profiles: geriatric
department (Gorunescu, McClean and Millard,
2002), intensive care unit (Ridge et al., 1998;
Cochran and Roche, 2008), intermediate care,
obstetrics/gynaecology and surgical departments
(Cochran and Roche, 2008) and paediatric intensive
care unit (Kokangul, 2008).
Mathematical distributions were originated from
data collections as diverse as: department historical
patient arrival rate/LoS, midnight census by
department, midnight bed holds by department and
patient billed nights by level of care. All of the
analyzed studies point out advantages in the use of
mathematical models to forecast department
demands in terms of allocating the right number of
beds. It was concluded that the mathematical models
also provide better information concerning cost
structures and revenue characteristics and how these
affect capacity and resource allocation decisions.
Given accurate data about the actual costs of
occupied and empty beds, modelling could be used
to balance the cost of providing excess standby beds
(accessibility), with the costs associated with
rejecting patients. Thus, the modelling approach
facilitates the provision of cost efficient and cost
effective services.
Four studies addressed the use of information
technologies to support and facilitate inpatient
placement. Reuille (2004) proposed a centralized
bed management system (Bed Control Report) based
on five Excel spreadsheets, dispersed by four
departments, were information was inputted by the
target department staff. Szabo (2003) describes the
use of the Bed Tracking System, developed by Tele-
Tracking Technologies, to support the bed
placement workflow and centralize bed status
information (bed released, bed in maintenance and
bed available), that is entered into the system by the
hospital staff (administrative clerk, clinicians and
housekeepers) using specific coded calls. A very
similar approach is described by Blair (2005) were
the BedCentral system, developed by MediLogistics,
is also used to centralize bed status information
entered by the hospital staff. Unlike the Bed
Tracking System, the information is inputted on both
MODELING DECISIONS FOR HOSPITAL BED MANAGEMENT - A Review
505