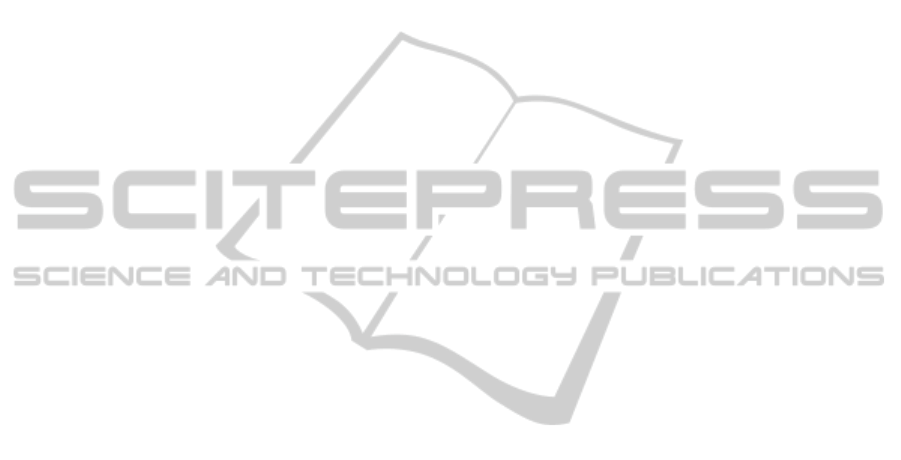
5 CONCLUSIONS AND FUTURE
WORK
Electrical Impedance Tomography was developed in
the early 1980s and it has since shown real potential
to being exploited for clinical use (bedside
monitoring in the Intensive Care Unit - ICU). Recent
developments in the field of absolute EIT
demonstrate how one may use it to estimate absolute
values of lung volumes which are key to any on-line
EIT based monitoring system. However, the current
system can be further improved, in particular in the
area of lung volume estimation accuracy. In this
study a Neural-Fuzzy modelling structure is used to
model the relationship between the lung absolute
resistivity and lung volume (lung R-V). Data
recordings were used from eight (8) healthy subjects
in a sitting position in order to train the models. It
was shown that the modelling structure can model
very accurately the aEIT lung volume estimation,
although this method forces the model to ‘inherit’
the inaccuracies associated with the aEIT theoretical
and empirical equations. In a different approach, it
was also shown how one can model the lung R-V by
‘bypassing’ the physical equations and directly
model the lung volume based on real volumetric
measurements using Spirometry (to record relative
volume) and Body Plethysmography (to record lung
Residual Volume). To our knowledge this is the first
data-driven model developed to describe the
behaviour of lung Resistivity-Volume in the
absolute EIT system. The developed models show a
very good agreement between the real data and the
model predictions, however high inter-individual
differences were also noted. Although, on an
individual basis, each ANFIS model (patient-
specific) outperforms the current aEIT system’s lung
volume estimations. In clinical science, inter-patient
variability is endemic; this is why it is of the opinion
of the authors that an extension to the presented
approach is needed, whereby the model auto-
calibrates to account for inter-individual differences
between patients. The new modelling structure
should be able to classify the ‘patient-type’ based on
the R-V behaviour curves and adjust the predictions
accordingly.
ACKNOWLEDGEMENTS
The authors would like to acknowledge the UK-
EPSRC for the financial support under Grant
Number EP/F02889X/1.
REFERENCES
Barber, D. C., Brown, B. H., 1984, ‘Applied potential
tomography’, Journal of Physics E: Scientific
Instruments, 17: 723-733.
Barber, D. C., Seagar, A. D., 1987, ‘Fast reconstruction of
resistance images’, Clinical Physics & Physiological
Measurement, vol. 8, Supplement A, pp. 47-54.
Barber, D. C., Borsic, A., 2005, ‘Electrical Impedance
Tomography: Methods, History and Application’,
Institute of Physics.
Brown, B. H., 2003, ‘Electrical impedance tomography
EIT: a review’, Journal of Medical Engineering &
Technology, vol. 27, no. 3: 97-108.
Brown, B. H., Barber D. C., 1985, ‘Applied potential
tomography: possible clinical applications’, Clinical
Physics & Physiological Measurement, vol. 6, no. 2:
109-21.
Brown, B. H., Leathard, A. D., 1995, ‘Measured and
expected Cole parameters from electrical impedance
tomographic spectroscopy images of the human
thorax’, Physiological Measurement, vol. 16,
Supplement 3A: A57-67.
Brown, B. H., Primhak, R. A., Smallwood, R. H., P.
Milnes, Narracott, A. J., Jackson, M. J., 2002,
‘Neonatal lungs-can absolute lung resistivity be
determined non-invasively?’, Med & Biological Eng
& Computing, 40, pp.388-394.
Brown, B. H., Mills, G. H., 2006, ‘Indirect measurement
of lung density and air volume from Electrical
Impedance Tomography EIT data’, World Congress
on Medical Physics and Biomedical Engineering,
Seoul, Korea.
Coulombe, Nicolas., Gagnon, Herve., Marquis, Francois.,
Skrobik, Yoanna., Guardo, Robert., 2005, ‘A
parametric model of the relationship between EIT and
total lung volume’, Physiological Measurement 26
401-411.
Harris, N. D., Suggett, A. J., Barber, D. C., Brown, B. H.,
1987 ‘Applications of applied potential tomography
APT in respiratory medicine’, Clin. Phys. Physiol.
Meas., vol. 8, pp. 155-165.
Harris, N. D., Suggett, A. J., 1988. ‘Applied potential
tomography: a new technique for monitoring
pulmonary function’, Clinical Physics &
Physiological Measurement vol. 9 Supplement A: 79-
85.
Jang, J., 1993, ‘ANFIS: Adaptive-Network-Based Fuzzy
Inference System’, IEEE Trans. On Systems, Man and
Cybernatic, 23, pp.665-685.
Panoutsos G, Tunney, D. R., Mills G. H., Al-Jabary, T.,
Mahfouf, M., Brown, B. H., 2008, ‘An improved
algorithm for accurate absolute EIT lung volume
estimation and localisation’, Proceedings of the
International Conference of the ATS (American
Thoracic Society), May 16-21, Toronto, Canada.
Panoutsos G, Tunney, D. R., Mills G. H., Al-Jabary, T.,
Mahfouf, M., Brown, B. H., 2008, ‘Electrical
Impedance Tomography: an evaluation of its ability to
detect changes in lung volume and expansion during
NEURAL-FUZZY MODELLING OF LUNG VOLUME USING ABSOLUTE ELECTRICAL IMPEDANCE
TOMOGRAPHY
49