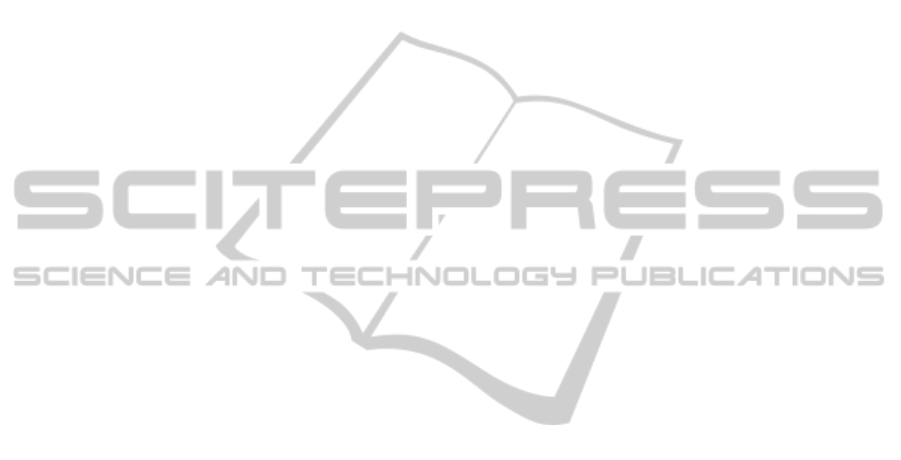
the ECG artifacts become more dominant (lower
SNR). This can be explained by the limitations of
the template subtraction technique. The algorithm
uses the quasi-periodic property of the ECG artifact
but assumes a constant waveform of successive heart
beats. Furthermore, perfect localization of the
occurrence of the heart beat is needed. If one of
these assumptions is not fulfilled, the algorithm will
introduce subtraction artifacts. In reality, the
successive waveforms are slightly varying and
perfect localization in the sEMG signal itself is
difficult. Thus, the larger the ECG interference
signal is compared to the background sEMG signal,
the larger these subtraction artifacts are. This
explains the higher RRMSE for lower SNR. These
limitations do not hold for both ICA based
algorithms as these algorithms exploit statistical
properties of both underlying signals to separate
them.
The difference in performance between the
results of wICA and EEMD-ICA can be explained
via differences in decomposing the original signal.
The EEMD is a data-driven method and has a more
natural decomposition that is able to cope with
nonstationarities in the signal. Contrary to the
wavelet decomposition, the extracted intrinsic mode
functions can be spectrally overlapping. This leads
to a more natural selection of the independent
sources of the ICA afterwards, explaining the small
differences in favor for the EEMD-ICA.
A major drawback of the EEMD-ICA algorithm
is its computational cost. The empirical mode
decomposition is a data driven, iterative process of
selecting local maxima and minima for each
empirical mode. This is a computationally intensive
decomposition. The noise robust extension of EMD,
called ensemble EMD (EEMD), needs more time as
the algorithm ensembles the outcome of at least 100
trials of a single EMD. In contrary, the wavelet
decomposition is a straight-forward method based
on a predefined wavelet waveform. The
computational load of wICA is similar to that of
template subtraction, while EEMD-ICA is in the
order of 100 times slower. This high computational
load makes a real-time implementation impossible.
5 CONCLUSIONS
In this paper, we reported on applying EEMD-ICA
to remove the ECG interference signal from single
channel sEMG recordings. The algorithm shows
better performance compared to template subtraction
and wavelet based ICA for both high and low signal-
to-artifact, but at the expense of a high
computational load. We can conclude that this
method has great potential in eliminating spike-like
artifacts in electro-physiological signals.
ACKNOWLEDGEMENTS
Research supported by: Research Council KUL:
GOA Ambiorics, GOA MaNet, CoE EF/05/006
Optimization in Engineering (OPTEC), IDO 05/010
EEG-fMRI, IDO 08/013 Autism; Belgian Federal
Science Policy Office: IUAP P6/04 (DYSCO,
`Dynamical systems, control and optimization',
2007-2011; EU: FAST (FP6-MC-RTN-035801),
Neuromath (COST-BM0601)
REFERENCES
Azzerboni, B., Carpentieri, M., La Foresta, F., Morabito,
F. C., 2004. Neural-ICA and Wavelet Transform for
artifacts removal in EMG. In Proc. Of the IEEE
International Joint Conference on Neural Networks,.
4, 3223–3228.
Bartolo, A., Roberts, C., Dzwonczyk, R., Goldman, E.,
1996. Analysis of diaphragm EMG signals:
comparison of gating vs. subtraction for removal of
ECG contamination. In J. Appl. Physiol, 80, 1898–
1902.
Davies, M. E., James, C. J., 2007. Source separation using
single channel ICA. In Signal Processing, 87 (8), 1819
– 1832.
Huang, N. E., Wu, M. L., Long, S. R., Shen, S. S., Qu, W.
D., Gloersen, P., Fan, K. L., 1998. The Empirical
Mode Decomposition and the Hilbert spectrum for
nonlinear and non-stationary time series analysis. In
Proceedings of Royal Society Lond, 454A, 1971, 903–
993.
Hyvarinen, A., Oja, E., 2000. Independent Component
Analysis: algorithms and applications. In Neural
Networks, 13 (4-5), 411 – 430.
Mijovic, B., De Vos, M., Gligorijevic, I., Taelman J., Van
Huffel, S., 2010. Source separation from single-
channel recordings by combining empirical mode
decomposition and independent component analysis.
In IEEE transactions on biomedical engineering.
Accepted.
Taelman, J., Spaepen, A., Van Huffel, S., 2007. Wavelet
Independent Component Analysis to remove
electrocardiography contamination in surface
electromyography. In Proceedings of Engineering in
Medicine and Biology Society, 2007, pp. 682–685.
Zhou, P., Kuiken, T. A., 2006 Eliminating cardiac
contamination from myoelectric control signals
developed by targeted muscle reinnervation. In
Physiol Meas, 27, 1311–1327.
BIOSIGNALS 2011 - International Conference on Bio-inspired Systems and Signal Processing
424