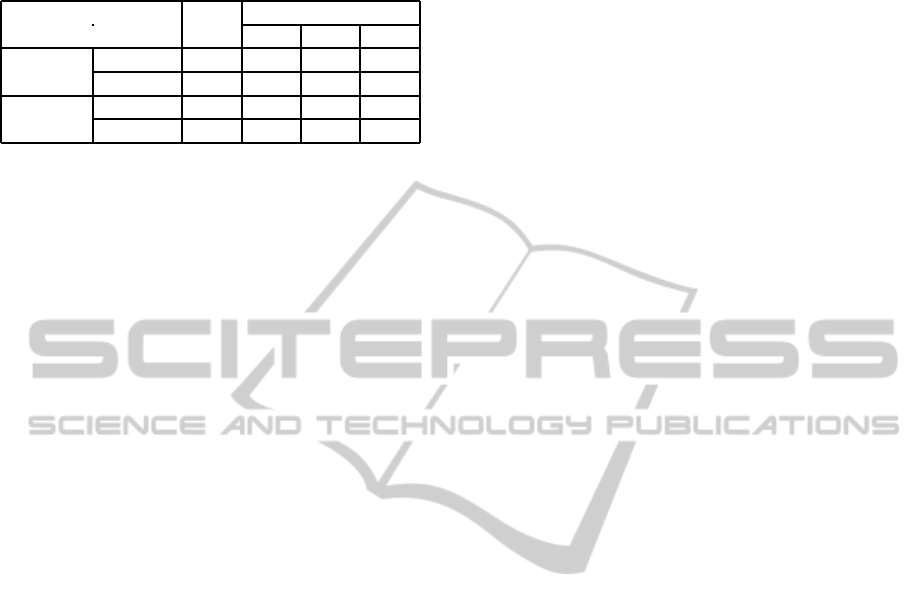
Table 2: Deviations for theoretical and neural PCS from ex-
pected compensations (with original non perturbated data)
using perturbated training data and 3000 epochs.
TPCS and neural Th. Training data
CPCS deviations PCS 50 100 200
Carbo- Average 0 0 0 0
hydrates Max 2 4 4 4
Insulin
Average 1.12 1.10 0.95 1.10
Max 3.29 3.22 1.84 3.20
on initial values introduce a dynamic modification of
the initial sensitivity to insulin which cannot be mod-
elled by the TPCS: the mathematical formulaes lead
to generate errors proportional to the deviation intro-
duced by the perturbation. On the contrary, the neural
CPCS, thanks to its neural predictor, demonstrates its
ability to suggest more acceptable evaluations.
Once again, the neural CPCS trained with 100
data seems to provide the best measurements: in this
case, the maximum insulin deviation is divided by
a factor of 1.8 between the TPCS and the neural
CPCS. The results of table 2 demonstrate that the neu-
ral CPCS suggests either comparable or more accu-
rate values than the TPCS. The neural CPCS is able
to genealize an average compensation function from
perturbated training data and give good approxima-
tions, whereas the TPCS is more rigid. The neural
CPCS ability to approximate the compensation func-
tion even when perturbated data are used is mostly
due to the training process tuning (in terms of num-
ber of training data and maximum number of epochs)
which reduces the noise generated by perturbations
on training data.
4 CONCLUSIONS
An adaptive GPCS has been proposed. Theoretical
and neural variants of this global system (TPCS and
neural CPCS, respectively) have been presented and
compared using both exact and up to 20% perturbated
data. Each datum is randomly generated, though con-
forming to the theoretical model proposed by (Char-
pentier et al., 2005). As a result, the neural approach
appeared to be accurate and adaptable to each pa-
tient’s reaction to insulin and glucose. This study
highlights that NPC techniques provide similar mea-
surement to empirical models and are able to handle
perturbations. The main drawback of NPC lies in the
amount of training data required to perform an ac-
ceptable training. This can be problematic when the
system does not have access to enough information.
Use of predetermined ANN based on a type 1 diabetes
database is investigated.
Compared with other type 1 diabetes closed-loop
control systems in the literature (Takahashi et al.,
2008), the Global PCS architecture is highly modu-
lar thanks to programming interfaces which allow to
define its effective behaviour.
Although this study demonstrated that the neu-
ral predictor provides acceptable glycaemia devia-
tions, complementary work is still necessary to eval-
uate other ANN configurations and activation func-
tions. The neural CPCS can be used “as is”. How-
ever, further testing is needed in case of meals recov-
ery (which occurs when a patient eats while the previ-
ous meal short-term insulin has not fully taken effect).
Long-term insulin regulation is currently left for fu-
ture works as the corresponding controlled and math-
ematical models are much more complex due to the
combined short-term and long-term insulin effects.
Future works will also concern the definition of train-
ing data selection heuristics. These heuristics will be
used to retain the most relevant training information
and leave unnecessary information out.
Finally, similar experiments could also be pro-
posed on systems which make use of Support Vector
Machine (SVN) techniques in place of ANN.
ACKNOWLEDGEMENTS
Jointly to the University of Technology of Belfort-
Montbeliard (UTBM), France, this work is supported
by the European Center for Diabetes Studies, Stras-
bourg, France and The ACTIMAGE Group, France.
REFERENCES
Charpentier, G., Virally, M., Varroud-Vial, M., Hochberg,
G., Riveline, J.-P., Stevenin, C., Lejeune, M., Re-
queda, E., and Benoit, A. (2005). Libert´e Alimentaire
et Diab`ete. Centre Hospitalier Sud Francilien.
Mhaskar, H. (2005). Approximation properties of a mul-
tilayered feedforward artificial neural network. Ad-
vances in Computational Mathematics, 1(1):61–80.
Riedmiller, M. and Braun, H. (1993). A direct adapta-
tive method for faster backpropagation learning: The
RPROP algorithm. In ICNN’93, IEEE International
Conference on Neural Networks, pages 563–568.
Takahashi, D., Xiao, Y., and Hu, F. (2008). A survey of
insulin-dependent diabetes-part ii: control methods.
International Journal of Telemedicine and Applica-
tions, 2008:1–14.
HEALTHINF 2011 - International Conference on Health Informatics
528