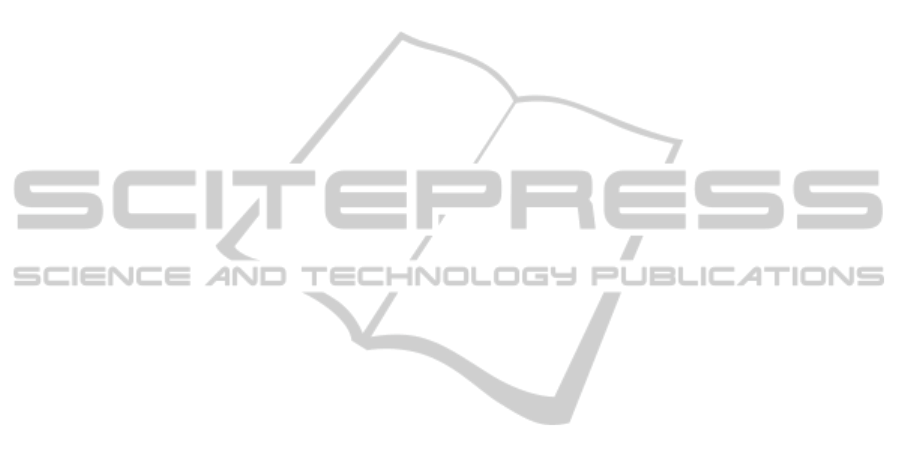
faces. A shortcoming of the proposed methods is that
since the water flow model always evolves from in-
side the region to outside, the coarse segmentation
output has to be within the target boundary of the hip-
pocampus. This limitation can be addressed by de-
signing techniques which permit a two-sided evolu-
tion of the water front.
Overall, it can be concluded that the obtained re-
sults show promise and pave way for applying of
the water flow model for segmenting other (partially)
weak-edge structures as well. Future work will be
targeted at complete automation of this method by
reliable automatic initialisation of seed points or re-
placing the region growing with any other method for
coarse segmentation.
ACKNOWLEDGEMENTS
The authors would like to thank Dr. D.Ravi Varma,
DM, KIMS hospital, Hyderabad for his input towards
anatomical structure of hippocampus and qualitative
analysis of results.
REFERENCES
Ahmed, M., Yamany, S., Mohamed, N., Farag, A., and Mo-
riarty, T. (2002). A modified fuzzy c-means algorithm
for bias field estimation and segmentation of mri data.
Medical Imaging, IEEE Transactions on, 21(3):193 –
199.
Akhondi-Asl, A., Jafari-Khouzani, K., Elisevich, K., and
Soltanian-Zadeh, H. (2010). Hippocampal volume-
try for lateralization of temporal lobe epilepsy: Auto-
mated versus manual methods. NeuroImage, In Press,
Corrected Proof:–.
Akselrod-Ballin, A., Galun, M., Gomori, J. M., Brandt,
A., and Basri1, R. (2007). Prior knowledge driven
multiscale segmentation of brain mri. MICCAI 2007,
4792/2007:118–126.
Bernasconi, N., Bernasconi, A., Caramanos, Z., Antel,
S. B., Andermann, F., and Arnold, D. L. (2003).
Mesial temporal damage in temporal lobe epilepsy: a
volumetric MRI study of the hippocampus, amygdala
and parahippocampal region. Brain, 126(2):462–469.
Chupin, M., Cuingnet, R., Lemieux, L., Lehericy, S., Be-
nali, H., Garnero, L., and Colliot, O. (2008). Fully au-
tomatic hippocampus segmentation discriminates be-
tween alzheimer’s disease and normal aging - data
from the adni database. In MICCAI Workshop on
CAPH.
Hurley, D. J., Nixon, M. S., and Carter, J. N. (2005). Force
field feature extraction for ear biometrics. Computer
Vision and Image Understanding, 98(3):491 – 512.
Jafari-Khouzani, K. (2010). Mri data set for hip-
pocampus segmentation by department of di-
agnostic radiology at henry ford hospital.
http://www.radiologyresearch.org/HippocampusSeg
mentationDatabase/.
Liu, X. U. and Nixon, M. S. (2007). Image and volume
segmentation by water flow. In ISVC’07: Proceed-
ings of the 3rd international conference on Advances
in visual computing, pages 62–74, Berlin, Heidelberg.
Springer-Verlag.
Ltjnen, J. M., Wolz, R., Koikkalainen, J. R., Thurfjell, L.,
Waldemar, G., Soininen, H., and Rueckert, D. (2010).
Fast and robust multi-atlas segmentation of brain mag-
netic resonance images. NeuroImage, 49(3):2352 –
2365.
McInerney, T. and Terzopoulos, D. (1999). Topology adap-
tive deformable surfaces for medical image volume
segmentation. Medical Imaging, IEEE Transactions
on, 18(10):840 –850.
Morra, J., Tu, Z., Apostolova, L., Green, A., Toga, A., and
Thompson, P. (2008). Automatic subcortical segmen-
tation using a novel contextual model. MICCAI.
Morra, J., Tu, Z., Apostolova, L., Green, A., Toga, A., and
Thompson, P. (2010). Comparison of adaboost and
support vector machines for detecting alzheimer’s dis-
ease through automated hippocampal segmentation.
Medical Imaging, IEEE Transactions on, 29(1):30 –
43.
Pohle, R., Behlau, T., and Toennies, K. D. (2003). Segmen-
tation of 3d medical image data sets with a combi-
nation of region-based initial segmentation and active
surfaces. Medical Imaging 2003: Image Processing,
5032:1225–1232.
Sabuncu, M. R., Yeo, B. T., Leemput, K., Fischl, B., and
Golland, P. (2009). Supervised nonparametric im-
age parcellation. In MICCAI 2009, pages 1075–1083,
Berlin, Heidelberg. Springer-Verlag.
UNSUPERVISED 3D SEGMENTATION OF HIPPOCAMPUS IN BRAIN MR IMAGES
187