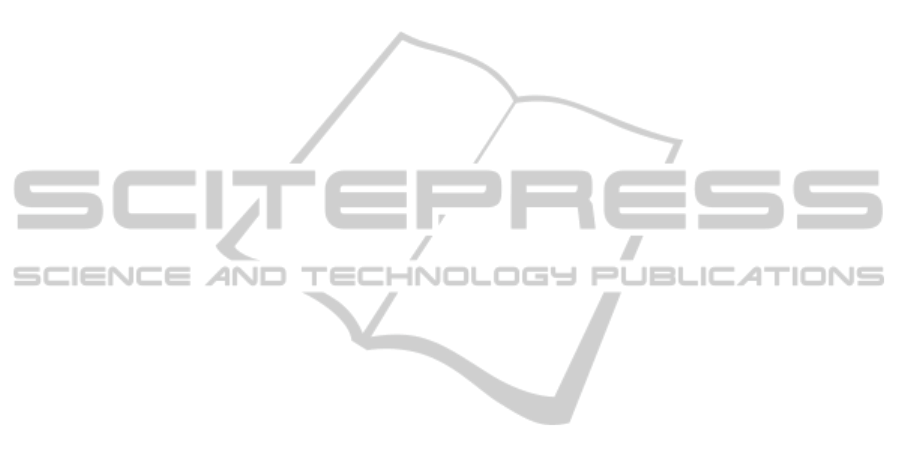
been proposed in (Habets, 2004) to enhance speech
degraded by reverberation.
In general to improve robustness of the noisy
speech, processing can be performed at signal, fea-
ture or model level. Speech enhancement techniques
aim at improving the quality of speech signal cap-
tured through single microphone or microphone ar-
ray (Omologo et al., 1998; Martin, 2001). Robust
acoustic features attempt to represent parameters less
sensitive to noise by modifying the extracted fea-
tures. Common techniques include cepstral mean nor-
malization (CMN) and cepstral mean subtraction and
variance normalization (CMSVN) and relative spec-
tral (RASTA)filtering (Droppo and Acero, 2008; Her-
mansky and Morgan, 1994). Model adaptation ap-
proach modify the acoustic model parameters to fit
better with the observed speech features (Omologo
et al., 1998; Gales and Young, 1995).
Performance of the human auditory system is
more adept at noisy speech recognition. Auditory
modeling, which simulates some properties of the
human auditory system have been applied to speech
recognition system to enhance its robustness. The in-
formation coded in auditory spike trains and the in-
formation transfer processing principles found in the
auditory pathway are used in (Holmberg et al., 2005;
Deng and Sheikhzadeh, 2006). The neural synchrony
is used for creating noise-robust representations of
speech (Deng and Sheikhzadeh, 2006). The model
parameters are fine-tuned to conform to the popula-
tion discharge patterns in the auditory nerve which
are then used to derive estimates of the spectrum on a
frame-by-frame basis. This was extremely effectivein
noise and improved performance of the ASR dramat-
ically. Various auditory processing based approaches
were proposed to improve robustness (Ghitza, 1988;
Seneff, 1988; Dau et al., 1996) and in particular, the
works described in (Deng and Sheikhzadeh, 2006;
Flynn and Jones, 2006) were focused to address the
additive noise problem. Further, in (Kleinschmidt
et al., 2001) a model of auditory perception (PEMO)
developed by Dau et al. (Dau et al., 1996) is used as
a front end for ASR, which performed better than the
standard MFCC for an isolated word recognition task.
Principles and models relating to auditory processing,
which attempt to model human hearing to some extent
have been applied for speech recognition in (Herman-
sky and Morgan, 1994; Hermansky, 1997).
The important aspect in a speech recognition sys-
tem is to have abstract representation of highly redun-
dant speech signal, which is achieved by frequency
analysis. The cochlea and hair cells of the inner
ear perform spectrum analysis to extract relevant fea-
tures. The models for auditory spectrum analysis are
based on filterbank design, which are usually char-
acterized by non-uniform frequency resolution and
non-uniform bandwidth on linear scale. Examples
include popular speech analysis techniques, namely
Mel frequency cepstrum and perceptual linear pre-
diction which try to emulate human auditory percep-
tion. Other important processing is based upon Gam-
matone filter bank, which is designed to model hu-
man cochlear filtering and is shown to provide robust-
ness in adverse noise conditions for speech recogni-
tion tasks (Flynn and Jones, 2006; Schlueter et al.,
2006). In (Flynn and Jones, 2006), gammatone based
auditory front-end exhibited robustperformance com-
pared to traditional front-ends based on MFCC, PLP
and standard ETSI frontend. For large vocabulary
speech recognition tasks, the performance of these
features have been competitive with standard fea-
tures like MFCC and PLP (Schlueter et al., 2006).
Another important psychoacoustic property is mod-
ulation spectrum of speech, which is important for
speech intelligibility (Dau et al., 1996; Drullman
et al., 1994). The relative prominence of slow tem-
poral modulations is different at various frequencies,
similar to perceptual ability of human auditory sys-
tem. Particularly, most of the useful linguistic in-
formation is in the modulation frequency components
from the range between 2 and 16 Hz, with dominant
component at around 4 Hz (R.Drullman et al., 1994;
Kanedera et al., 1999; Hermansky, 1997). Modula-
tion spectrum based features computed over longer
windows have been effective in measuring speech in-
telligibility in noisy environments (Houtgast et al.,
1980; Kingsbury, 1998).
In this work, an alternate approach based on psy-
choacoustic properties combining gammatone filter-
ing and modulation spectrum of speech, to preserve
both quality and intelligibility for feature extraction
is presented. Gammatone frequency resolution re-
duces the ASR system sensitivity to environmental
reverberant signal attributes and improve the speech
signal characteristics. Further, long-term modulation
preserves the linguistic information in the speech sig-
nal, improving the accuracy of the system. The fea-
tures derived from the combination are used to pro-
vide robustness, particularly in the context of mis-
match between training and testing reverberant envi-
ronments. The studied features are shown to be reli-
able and robust to the effects of the hands-free record-
ings in the reverberant meeting room. The effective-
ness of the proposed features is demonstrated with ex-
periments which use real-time reverberant speech ac-
quired through four different microphones. For com-
parison purposes the recognition results obtained us-
ing conventional features are tested, and usage of the
BIOSIGNALS 2011 - International Conference on Bio-inspired Systems and Signal Processing
52