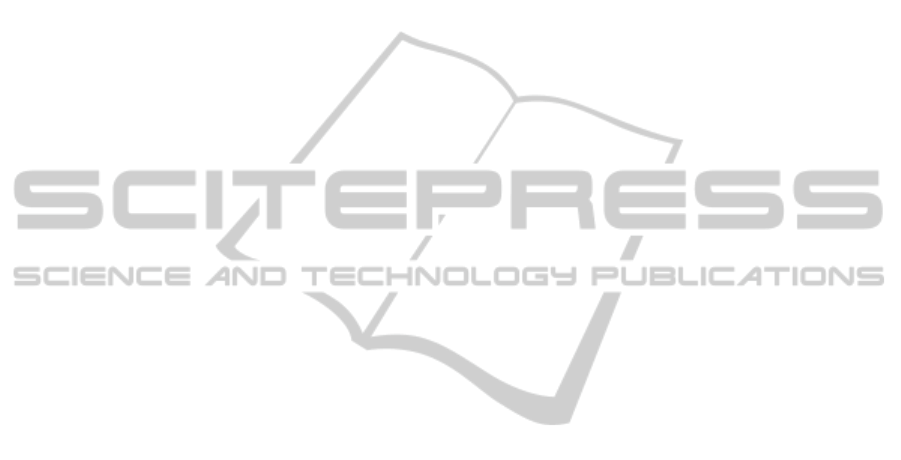
differences in pharmacokinetics (Ranta, 2002, Myles
et al., 2003). This is one of the most distressing
aspects of surgery as in the majority of times the
patients are unable to alert the anaesthetist that they
have regained consciousness during surgery, and are
in pain, due to the routine co-administration of
neuromuscular blocking agents with the anaesthetic
agents.
The most widespread commercial monitors of
hypnosis currently in use are the BIS monitor
(Aspect Medical Systems, Natick, MA) (Sigl and
Chamoun, 1994) and Datex-Ohmeda S/5
TM
Entropy
Module (originally by Datex-Ohmeda Division,
Instrumentation Corp., Helsinki; now with GE
Healthcare) (Viertiö-Oja et al., 2004). Such devices
operate by extracting a number of features from the
EEG in order to deduce the relative depth of
hypnosis, which is then easily visualised as a
number from 0-100 (100: fully awake, 0: no
activity). Even though these devices offer additional
support to the work of the anaesthetist, they still
suffer from some important reliability issues
(Russell, 2006, Barr et al., 1999, Dahaba, 2005,
Messner et al., 2003).
The main reason behind some of the problems
faced by existing monitors can be pinpointed to the
fact that they utilise a number of empirical measures
from the EEG, which are then combined in a
proprietory way into a single number denoting the
depth of anaesthesia. The information utilized is,
thus, representative of the characteristics of the
observed activity and not of the physiological
process that occurs during anaesthesia. Strong
evidence for this provide reported incidences of
awareness despite monitors displaying adequate
depth of anaesthesia (Rampersad and Mulroy, 2005,
Mychaskiw et al., 2001), and the inability of some
monitors to distinguish between the EEG of an
anaesthetized patient and the EEG of somebody who
is asleep (Russell, 2006, Sleigh et al., 1999). The
latter is not surprising considering that sleep and
anaesthesia share some common mechanisms (Voss
and Sleigh, 2007). However, despite the large
similarities, there are fundamental differences in the
particular physiological mechanisms of the two
processes which a true monitor of anaesthetic depth
should be able to identify. Thus, a successful
monitor should extract information from the
observed activity that is representative of the deeper
interactions and which reflects the physiological
changes that occur from administration of the
anaesthetic agents as these are manifest in the
observed activity. In other words, the measures
utilised must be based on ‘neurobiologic phenomena
that represent the necessary and sufficient conditions
for consciousness in a specific individual’ (Hudetz,
2008).
In recent years it has been shown that measures
which characterise the interactions between different
brain areas can provide an insight into how
integration of information is achieved in the brain
during various cognitive tasks. One such measure is
Granger causality, a linear measure quantifying the
bidirectional interaction between two time series.
Even though Granger causality has provided useful
information from EEG activity in a number of
applications (see (Pereda et al., 2005) and references
within), it has yet to be applied in the study of
general anaesthesia. In this work, the interactions
between different brain areas during induction of
anaesthesia are investigated using Granger causality.
Anaesthetic induction is important as one can readily
study the point of loss of consciousness that occurs
from the administration of a bolus of anaesthetic
agent. Such information is important in subsequent
monitoring of anaesthetic depth and identification of
potential regaining of consciousness during surgery.
2 METHODOLOGY
2.1 Dataset
The data has been collected from 10 male patients
(mean age 34.6±18) undergoing general and
urological surgery at Nicosia General Hospital,
Cyprus. The study has been approved by the Cyprus
National Bioethics Committee and patients gave
written informed consent for their participation.
Participants were not taking any medication acting
on the central nervous system and were of normal
weight. EEG data was collected using the 24-
channel configuration of the TruScan32 system
(Deymed Diagnostic) at a sampling rate of 256Hz.
Electrodes were placed at positions Fp1, Fp2, F7,
F3, Fz, F4, F8, T3, C3, Cz, C4, T4, T5, P3, Pz, P4,
T6, O1 and O2, according to the International 10/20
system. Data was recorded with an FCz, and ground
was located on the head. No filtering was performed
during data collection. Data recording commenced
while patients were still awake prior to
administration of the anaesthetic agents, continued
through loss of consciousness, during the entire
surgery, and until patients regained consciousness at
surgery end. During the recording event markers
were manually inserted to indicate important events,
such as administration of anaesthetic agents.
USING GRANGER CAUSALITY TO CHARACTERISE BIDIRECTIONAL INTERACTIONS IN THE HUMAN
BRAIN DURING INDUCTION OF ANAESTHESIA
189