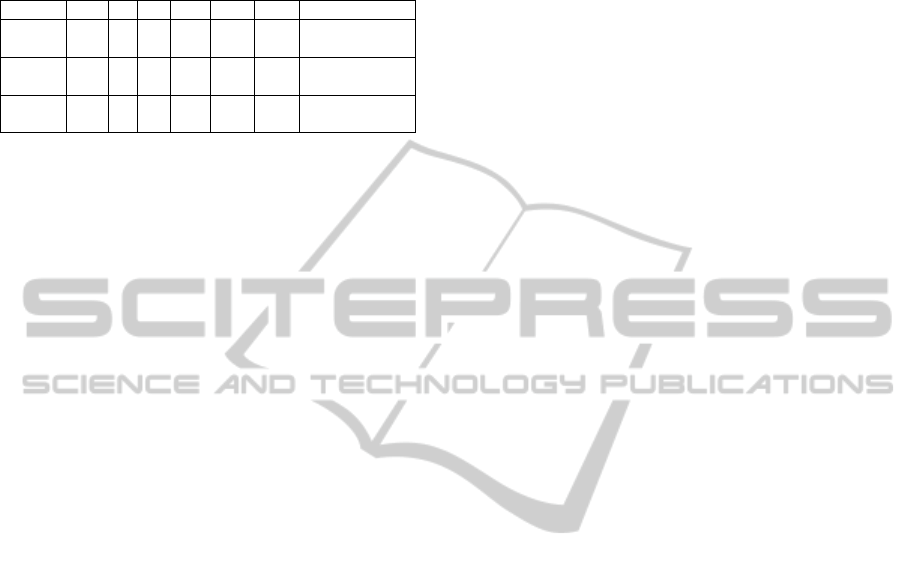
NIH prognostics were taken from the clinical dataset
as given by (Chang
et al., 2005).
Table 2: Comparative results between hybrid markers and
pure clinical indices (NIH, St Gallen).
TP FP FN TN Sens Spec Acc
Hybrid 13 12 28 81 0.32 0.87
94/134
(70.15%)
NIH 41 91 0 2 1 0.02
41/134
(32.09%)
St
Gallen
38 85 3 8 0.93 0.09
46/134
(34.33%)
Both indices have a very high sensitivity, but an
intolerable low specificity which would lead to give
unnecessary adjuvant systematic treatment to many
patients. Thus the obtained hybrid markers
outperforms also the pure clinically indices.
4 CONCLUSIONS
In this paper a new approach to perform cancer
prognosis is proposed based on a hybrid marker
selection. We evaluated our approach on a public
available breast cancer prognosis dataset. Patients
included in this dataset are classified into two groups
according to whether a distant subclinical metastasis
was occurred or not. This dataset represents two
challenges: high-dimensionality (microarray data)
and mixed-type data (clinical data). To cope
appropriately with this, a marker selection was
performed based on a fuzzy feature selection
approach which handles both challenges. It has been
shown that the obtained hybrid markers, composed
of clinical markers and genes, can improve the
prediction accuracy and outperform both genetic
based approaches (i.e. the well-known Amsterdam
70-genes signature) and pure clinical indices (St
Gallen and NIH). Moreover, the proposed approach
reduces significantly the number of markers needed
to perform a cancer prognosis task.
Future work will be devoted to test this algorithm on
other public available datasets and integrate other
sources of information than clinical and microarray
data.
REFERENCES
Aguado J. C., and Aguilar-Martin J., 1999. A mixed
qualitative-quantitative self-learning classification
technique applied to diagnosis, QR'99 The Thirteenth
International Workshop on Qualitative Reasoning.
Chris Price, 124-128.
Chang H. Y., Nuyten D. S. A., Sneddon J. B., Hastie T.,
Tibshirani R., et al., 2005. Robustness, scalability, and
integration of a wound-response gene expression
signature in predicting breast cancer survival. PNAS
2005, 102 (10): 3738-3743
Deepa, S., Claudine I., 2005. Utilizing Prognostic and
Predictive Factors in Breast Cancer. Current
Treatment Options in Oncology 2005, 6:147-159.
Current Science Inc
Gevaert O., De Smet F., Timmerman D., Moreau Y., De
Moor B., 2006. Predicting the prognosis of breast
cancer by integrating clinical and microarray data with
Bayesian network, Bioinformatics 22 (14), 184-190.
Golub T. , Slonim D., Tamayo P., Huard C., Gaasenbeek
M., et al., 1999. Molecular Classification of Cancer
Class Discovery and Class Prediction by Gene
Expression Monitoring, Science 286 (5439), 531-537.
Guyon I., Elisseeff A., 2003. An introduction to variable
and feature selection, J. Mach. Learn. Res, 3, 1157-
1182.
Hedjazi L., Aguilar-Martin J., Le Lann M. V., and
Kempowsky T., 2010a, Membership-Margin based
Feature Selection for Mixed-Type and High-
Dimensional Data, Fuzzy Sets and Systems Journal.,
submitted for publication.
Hedjazi, L., Kempowsky T., Le Lann M. V., Aguilar-
Martin J., 2010b. Prognosis of breast cancer based on
a fuzzy classification method. 3rd International Joint
Conference on Biomedical Engineering Systems and
Technologies (BIOSTEC 2010); 1st International
Conference on Bioinformatics (BIOINFORMATICS
2010). Valence (Spain), 20-23 January 2010, pp.123-
130.
Kohavi R., and John G. H., 1997. Wrapper for feature
subset selection, Artificial Intelligence 97, 273-324.
Ramaswamy S., Tamayo P., Rifkin R., Mukherjee S.,
Yeang C., et al., 2001. MultiClass Cancer Diagnosis
Using Tumor Gene Expression Signatures, Proc. Nat’l
Acad. Sc. USA 98 ( 26) , 15149-15154.
Straver M. E., Glas A. M., Hannemann J., Wesseling J.,
van de Vijver M. J., et al.,2009. The 70-gene signature
as a response predictor for neoadjuvant chemotherapy
in breast cancer, Breast Cancer Res. Treat.,
doi:10.1007/s10549-009-0333-
Sun Y., 2007. Iterative RELIEF for Feature Weighting:
Algorithms, Theories, and Applications, IEEE TPAMI,
2 (6) , 1035-1051.
Sun Y., Goodison S., Li J., Liu L., Farmerie W., 2007.
Improved breast cancer prognosis through the
combination of clinical and genetic markers.
Bioinformatics, Gene expression. Oxford University
Press 23 (1), 30-37.
Van’t Veer L.J., Dai H., van de Vijver M. J., et al., 2002.
Gene expression profiling predicts clinical outcome of
breast cancer, Nature, 415, pp. 530-536.
Van de Vijver M. J., He Y. D., Van’t Veer L. J., Dai H.,
Hart A., et al., 2002. A Gene expression signature as a
predictor of survival in breast cancer, N Engl J Med,
347 (25), pp. 1999-2009.
BIOINFORMATICS 2011 - International Conference on Bioinformatics Models, Methods and Algorithms
164