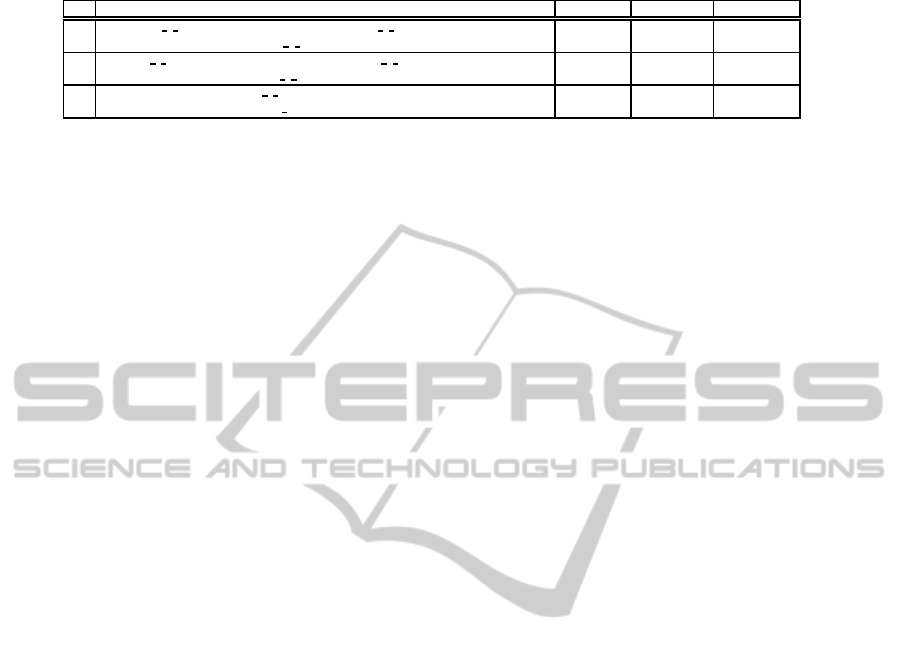
Table 1: Analyzed Rules.
Id Rule Sup. (%) Conf. (%) Ampl. (%)
1 215091 s at ∈ [98.35 , 376.99] and 215760 s at ∈ [527.04 , 1168.82] 52 100 17
=⇒ 203944 x at ∈ [890.80 , 5308.61]
2 205119 s at ∈ [783.83 , 1527.60] and 215597 x at ∈ [8301.78 , 9819.85] 20 100 16
=⇒ 212967 x at ∈ [2076.59 , 2592.60]
3 222099 s at ∈ [859.491 , 1425.210] 55 100 17
=⇒ 49327 at ∈ [1517.45 , 2239.45]
with support values less than 50 % where in almost
cases cover records or endotoxin-treated group or
placebo group.
The number of rules covering the placebo group is
significantly higher, which makes sense because this
group has gene expression values more stable and fre-
quent than the group treated with endotoxin(Rubio-
Escudero, 2007). To examine the relevance of the
rules obtained in the studied problem, we have used
the Onto-CC software (Romero-Zliz et al., 2008),
which retrieves information regarding the functional-
ity of a set of genes that is passed as a query, and a PI
value (the probability of intersection) associated with
the relevance of these genes appear together in one
rule. PI is a value to minimize between 0 and 1 and
considered relevant those obtained under 0.05.
The results of only 3 rules are listed in Table 1 for
readability. When Onto-CC is applied, the PI values
obtained for every rule are quite low, indicating the
relevance of grouping these three genes with respect
to these terms, immune response and related terms are
explicitly included.
4 CONCLUSIONS
In this paper we present the result of applying an evo-
lutive technique for extracting association rules from
microarray data. We have seen the rules obtained are
able to successfully characterize the dataset applied,
either covering almost all samples, or covering sam-
ples only one of the two groups in the data: treated
with endotoxin or treated with placebo. In addition,
the mean amplitude of the intervals was 24.7%, which
justifies the use of quantitative rules in place of the
classical rules.
We have shown the relevance of the rules obtained
for the problem studied using the Onto-CC program.
The PI values obtained show significance in the group
of genes found in the rules, and secondly the terms
obtained querying Gene Ontology are closely related
to the problem of inflammation.
Thus, we conclude that the use of quantitative as-
sociation rules, in particular those obtained by the
algorithm proposed, is a valid method for analyzing
microarray data, and we consider it a starting point
for future work, applying this technique to other mi-
croarray data, comparing with other analytical tech-
niques and seeing the importance of the influence
antecedent-consequent obtained by the rules with re-
gard to genetic networks.
ACKNOWLEDGEMENTS
The financial support from the Spanish Ministry of
Science and Technology, project TIN 2007-68084-C-
02, and from the Junta de Andaluca, project P07-TIC-
02611, is acknowledged.
REFERENCES
Calvano, S. E., Xiao, W., Richards, D. R., Felciano, R. M.,
Baker, H. V., Cho, R. J., Chen, R. O., Brownstein,
B. H., Cobb, J. P., Tschoeke, S. K., Miller-Graziano,
C., Moldawer, L. L., Mindrinos, M. N., Davis, R. W.,
Tompkins, R. G., Lowry, S. F., and Large Scale Collab
Res Program, I. A. (2005). A network-based analysis
of systemic inflammation in humans. Nature, 437.
Durbin, R., Eddy, S., Krogh, A., and Mitchison, G. (1998).
Biological Sequence Analysis: Probabilistic Models
of Proteins and Nucleic Acids. Cambridge University
Press.
McIntosh, T. and Chawla, S. (2007). High-confidence rule
mining for microarray analysis. IEEE/ACM Transac-
tions on Computational Biology and Bioinformatics,
4(4):611–623.
Romero-Zliz, R., del Val, C., Cobb, J., and Zwir, I. (2008).
Onto-cc: a web server for identifying gene ontology
conceptual clusters. Nucleic Acids Res, 36(4):W352–
W357.
Rubio-Escudero, C. (2007). Fusion of Knowledge towards
Identification of Genetic Profiles in the Systemic In-
flammation Problem. Ph.D Thesis. Unversidad de
Granada.
Vannucci, M. and Colla, V. (2004). Meaningful discretiza-
tion of continuous features for association rules min-
ing by means of a som. In Proceedings of the Euro-
pean Symposium on Artificial Neural Networks, pages
489–494.
Venturini, G. (1993). SIA: a Supervised Inductive Algo-
rithm with genetic search for learning attribute based
concepts. In Proceedings of the European Conference
on Machine Learning, pages 280–296.
MINING QUANTITATIVE ASSOCIATION RULES IN MICROARRAY DATA USING EVOLUTIVE ALGORITHMS
577