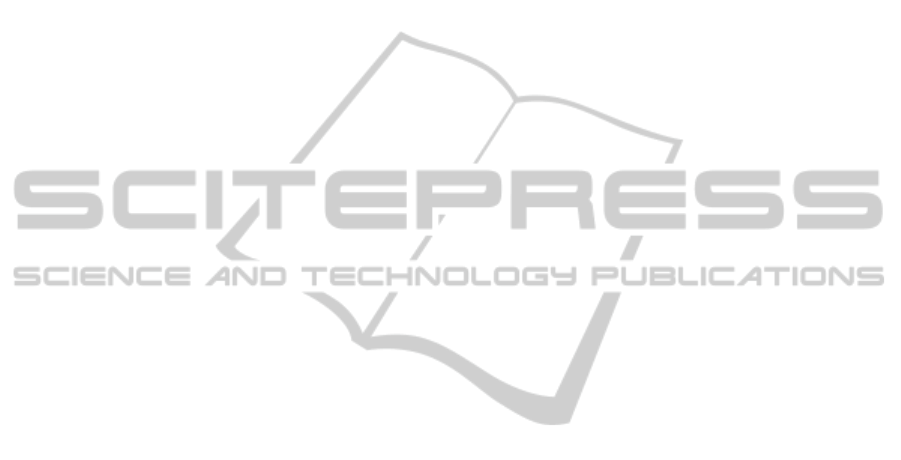
tems Modelling (SCI-SYM) in Dublin City Univer-
sity, and the Irish Centre for High End Computing
(ICHEC).
REFERENCES
Abraham, E. P. and Chain, E. (1940). An enzyme from
bacteria able to destroy penicillin. Nature, 146:837.
Bush, K., Macielag, M., and Weidner-Wells, M. (2004).
Taking inventory: antibacterial agents currently at or
beyond phase 1. Current opinion in microbiology,
7(5):466–476.
Fisher, J. F., Meroueh, S. O., and Mobashery, S. (2005).
Bacterial resistance to beta-lactam antibiotics: com-
pelling opportunism, compelling opportunity. Chemi-
cal reviews, 105(2):395–424.
Ginovart, M., Lopez, D., and Valls, J. (2002). Indisim, an
individual-based discrete simulation model to study
bacterial cultures. Journal of theoretical biology,
214(2):305–319.
Jennings, N. R., Sycara, K., and Wooldridge, M. J. (1998).
A roadmap of agent research and development. Au-
tonomous Agents and Multi-Agent Systems, 1(1):7–
38.
Levy, S. B. and Marshall, B. (2004). Antibacterial resis-
tance worldwide: causes, challenges and responses.
Nature medicine, 10(12 Suppl):S122–9.
Li, Q., Lee, J. Y., Castillo, R., Hixon, M. S., Pujol, C.,
Doppalapudi, V. R., Shepard, H. M., Wahl, G. M.,
Lobl, T. J., and Chan, M. F. (2002). Nb2001, a novel
antibacterial agent with broad-spectrum activity and
enhanced potency against beta-lactamase-producing
strains. Antimicrobial Agents and Chemotherapy,
46(5):1262–1268.
Murphy, J. T., Walshe, R., and Devocelle, M. (2007).
Agent-based model of methicillin-resistant staphylo-
coccus aureus and antibiotics in batch culture. In
Proceedings of 21st Annual European Simulation and
Modelling Conference, pages 409–414. Eurosis-ETI.
Murphy, J. T., Walshe, R., and Devocelle, M. (2008). A
computational model of antibiotic-resistance mecha-
nisms in methicillin-resistant staphylococcus aureus
(mrsa). Journal of theoretical biology, 254(2):284–
293.
Murphy, J. T., Walshe, R., and Devocelle, M. (2009). Mod-
elling the population dynamics of antibiotic-resistant
bacteria: An agent-based approach. International
Journal of Modern Physics C, 20(3):435–457.
Murphy, J. T., Walshe, R., and Devocelle, M. (2010). A
theoretical analysis of the pro-drug delivery system
for treating antibiotic-resistant bacteria. IEEE/ACM
Transactions on Computational Biology and Bioinfor-
matics, 99.
Neu, H. C. (1992). The crisis in antibiotic resistance. Sci-
ence, 257(5073):1064–1073.
Rautio, J., Kumpulainen, H., Heimbach, T., Oliyai, R., Oh,
D., Jarvinen, T., and Savolainen, J. (2008). Prodrugs:
design and clinical applications. Nature reviews.Drug
discovery, 7(3):255–270.
Slater-Radosti, C., Aller, G. V., Greenwood, R., Nicholas,
R., Keller, P. M., Jr, W. E. D., Fan, F., Payne, D. J., and
Jaworski, D. D. (2001). Biochemical and genetic char-
acterization of the action of triclosan on staphylococ-
cus aureus. The Journal of antimicrobial chemother-
apy, 48(1):1–6.
Smyth, T. P., O’Donnell, M. E., O’Connor, M. J.,
and StLedger, J. O. (2000). beta-lactamase-
dependent prodrugsrecent developments. Tetrahe-
dron, 56(31):5699–5707.
Stone, G. W., Zhang, Q., Castillo, R., Doppalapudi, V. R.,
Bueno, A. R., Lee, J. Y., Li, Q., Sergeeva, M., Kham-
batta, G., and Georgopapadakou, N. H. (2004). Mech-
anism of action of nb2001 and nb2030, novel antibac-
terial agents activated by beta-lactamases. Antimicro-
bial Agents and Chemotherapy, 48(2):477–483.
Zygmunt, D. J., Stratton, C. W., and Kernodle, D. S. (1992).
Characterization of four beta-lactamases produced by
staphylococcus aureus. Antimicrobial Agents and
Chemotherapy, 36(2):440–445.
BIOINFORMATICS 2011 - International Conference on Bioinformatics Models, Methods and Algorithms
308