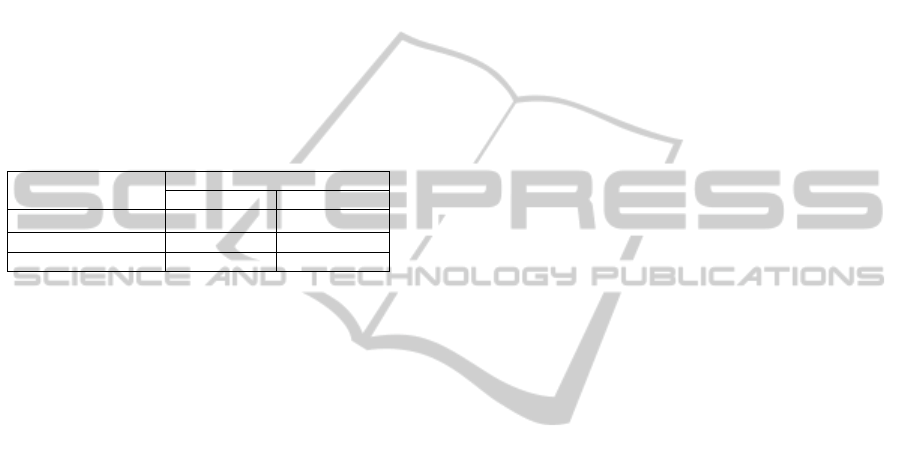
highest accuracy. The performance listed in Table 2,
indicates that the optimal number of states found by
the both evolved HMMs are identical to a manually
trained HMM. It also indicates that they are far more
optimised than a manually hand designed HMM
using the BW algorithm.
4.4 Experimental Performance
While the difference between the M-HMM and the
GA-HMM is not significantly large, comparing the
number of generations for the population based
search, using memetic algorithms to evolve the
HMM results in a faster
convergence to an optimal
solution as illustrated in Table 3.
Table 3: Convergence Times.
Model (Pop/Gen)
Convergence Generation
M-HMM GA-HMM
MA (50/60)
4
th
34
th
BV (50/30) 13
th
27
th
BG (50/60) 15
th
43
r
5 CONCLUSIONS
In summary, a novel way to represent images using
a fully automated structure discovery technique
involving Memetic Algorithms and HMM was
presented in this paper. A comparison was made
between various methods and the experimental
results have shown that M-HMM is capable of
searching for a more optimal structure than that
resulting from either the GA only approach or the
BW Algorithm.
By using evolutionary algorithms to evolve the
HMM, we can not only find the optimal number of
states to represent the image, but also manage to
optimise the initial transition probabilities for a
better trained model as indicated by its average
maximum likelihood. Although the recognition rate
of the M-HMM is just slightly better than the GA-
HMM, the former converged quicker to optimal
solutions suggesting that memetic algorithms can be
applied to situations where time is of an essence.
These results demonstrate that the EA evolved
HMMs are capable of context reasoning for
detecting micro aneurysms and thus facilitate finer
analysis during clinical sign detection on retina
images.
ACKNOWLEDGEMENTS
We would like to express our gratitude to Dr Tunde
Peto, MD, Head of Reading Centre, Department of
Research and Development, Moorfields Eye
Hospital NHS Foundation Trust, for her invaluable
advice and help. The authors also thank King Abdul-
Aziz University, Kingdom of Saudi Arabia, and the
Department of Computing, University of Surrey,
UK, for their financial support to the project.
REFERENCES
Abbass, H. A., 2002. “An evolutionary artificial neural
networks approach for breast cancer diagnosis”,
Artificial Intelligence in Medicine, Vol. 25.
Bakis, R., 1976. Continuous speech word recognition via
centisecond acoustic states, Proceedings ASA Meeting,
Washington,DC.
Bhuriyakorn, P., Punyabukkana, P., Suchato, A., 2008. “A
Genetic Algorithm-aided Hidden Markov Model
Topology Estimation for Phoneme Recognition of
Thai Continuous Speech” Ninth ACIS International
Conference on Software Engineering, Artificial
Intelligence, Networking, and Parallel/Distributed
Computing.
Goh, J., Tang, H. L., Al turk, L., Vrikki, C., Saleh, G.,
2010. “Detecting Micro aneurysms using Multiple
Classifiers and Hidden Markov Model”, 3rd
International Conference on Health Informatics,
Valencia, Spain.
Kwong, S., Chan, C. W., Man, K. F., Tang, K. S., 2001.
“Optimization of HMM topology and its model
parameters by genetic algorithms”, Pattern
Recognition, 34:509-522/
Lim, M. H., Xu, Y. L., 2005. “Application of Hybird
Genetic Algorithm in Supply Chain Management,”
Special Issue on Multi Objective Evolution: Theory
and Applications, Intternational Journal of Computers,
Systems and Signals, Vol. 6.
Liu, B., Wang, L., Jin, Y., Huang, D., 2007. “Designing
Neural Networks using PSO-Based Memetic
Algorithm”, Advances in Neural Networks, Vol. 4493.
Lu, G., Jiang, D., Zhao, R., 2007. “Single Stream DBN
Model Based Triphone for continuous speech
recognition”, Proceedings of the 9
th
IEEE
International Symposium on Multimedia Workshop.
Meindert, N., Ginneken, B., Stal, J., Suttorp-Schulten, M.,
Abramoff, M., 2005. “Automatic Detection of Red
Lesions in Digital Color Fundus Photograph”, IEEE
Transaction on Medical Imaging, Vol. 25(5).
Morizane, K., Nakamura, K., Toda, T., Saruwatari, H.,
Shikano, K., 2009. “Emphasized Speech Synthesis
Based on Hidden Markov Models”, 2009 Oriental
COCOSDA International Conference on Speech
Database and Assessments.
HEALTHINF 2011 - International Conference on Health Informatics
240