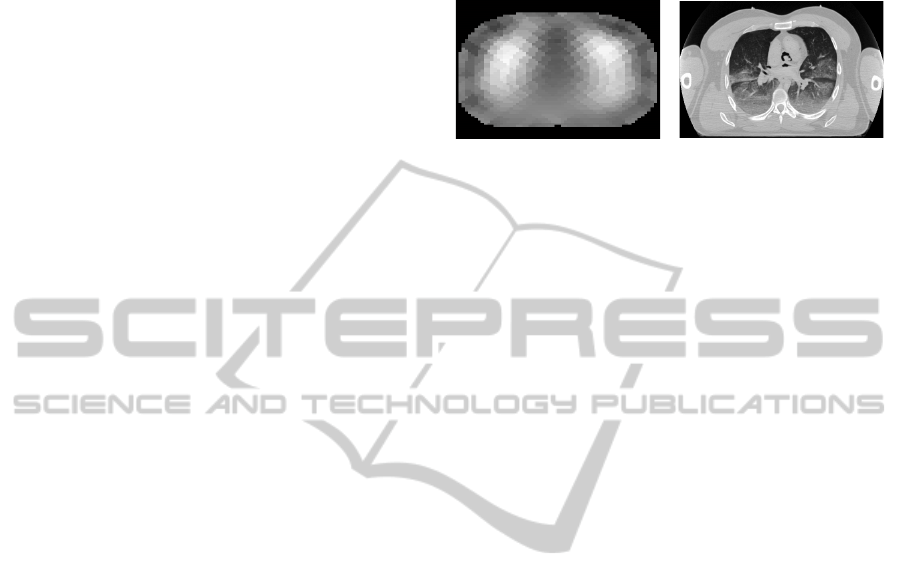
methods. A medical image can then be
reconstructed, since the structures within the human
body have different resistivities. However, this
requires the solution of a non-linear, ill-conditioned
inverse problem. The non-linearity arises in σ, since
the potential distribution. φ, is a function of the
impedance, φ= φ(σ), and the ill-conditioning stems
from the fact that small errors in the measurements
or in the forward modelling step may introduce large
errors in the reconstruction. The forward problem in
EIT is to estimate the induced electrical
measurements at the electrodes, given an excitation
signal and permittivity distribution. The inverse
problem estimates the permittivity distribution based
on the excitation signal and the terminal electrical
measurements. Once images have been
reconstructed, following regularization of the
inverse problem, in the final stage, FEM
triangulation results are rasterised to cover a
rectangular grid for subsequent image processing.
2 EIT LUNG IMAGES
2.1 Low Spatial Resolution of EIT
Images
It is well known that a reconstructed EIT image is
unique for noise-free complete boundary data
(Sylvester and Uhlmann, 1986). However, in
comparison to magnetic resonance imaging (MRI)
and computed tomography (CT), EIT suffers from
poor spatial resolution due to noise, low sensitivity
of boundary voltages to inner conductivity
perturbations and a limited number of boundary
voltage measurements (Clay and Ferree , 2002).
Moreover, the reconstructed images are usually
subtracted from a reference frame in order to
minimize errors due to electrode movement or
unknown boundary shape. A comparison of an EIT
image and its CT counterpart of the thorax is shown
in Figure 1. In spite of the above, EIT is very useful
in monitoring patient lung volume, because the air
has a large conductivity contrast compared to other
tissues in the thorax. The large change in lung
impedance with respiration, and the ease of use of
impedance tomography as a monitoring technique,
has led to a significant body of research in lung
impedance (Frerichs, 2000). However, the spatial
resolution of the EIT images reduces further with the
rasterisation process, where FEM model results are
mapped onto a rectangular grid for further image
processing. This rasterisation step introduces further
fuzziness to the reconstructed regions of
conductivity changes in the EIT images of the lungs,
and makes it even harder to determine the outline of
these rapidly changing regions during the breathing
cycle.
(a) (b)
Figure 1: (a) CT image of the thorax (Ackermann, 1995)
(b) EIT difference image (brighter regions correspond to
larger conductivity changes).
2.2 Feasibility of EIT Image
Segmentation
Due to the aforementioned problems regarding the
poor spatial resolution of EIT images, a question
arises as to whether it is possible to introduce a
robust adaptive EIT segmentation method.
Currently, there exists no method, which could
automatically segment regions with significant
conductivity changes, corresponding to the lobes for
an entire EIT breathing cycle sequence. The usual
method of segmenting or interrogating images is to
select a region of interest (a pixel or a small region)
on the image and then derive statistical measures for
the selected regions (Smallwood, 1999).
An additional problem is that EIT patient
histories generally include data from a limited
battery of tests, thus, making it difficult to train a
sufficiently complete probabilistic model.
Traditional background subtraction algorithms are
not appropriate due to the slower inflation/ deflation
rate of the lungs compared to the acquisition frame
rate (i.e., 13 fps), hence changes in the lung lobe
conductivity images appear slow moving or
temporarily stationary. Under these conditions, the
background becomes corrupted and object/blob
detection becomes erroneous.
To address the above issues, in the following
section a two-fold approach is proposed to tackle
this. In the first step, we carry out segmentation on
the FEM meshes prior to the rasterisation stage. This
prevents regions becoming even fuzzier and
facilitates the estimation of accurate measurement
results, which is a prerequisite for the extraction of
much needed ventilation parameters. In the second
step, we use a probabilistic model, which
accommodates both temporal and spatial contiguity
of mesh element values in order to segment and
BIOSIGNALS 2011 - International Conference on Bio-inspired Systems and Signal Processing
216