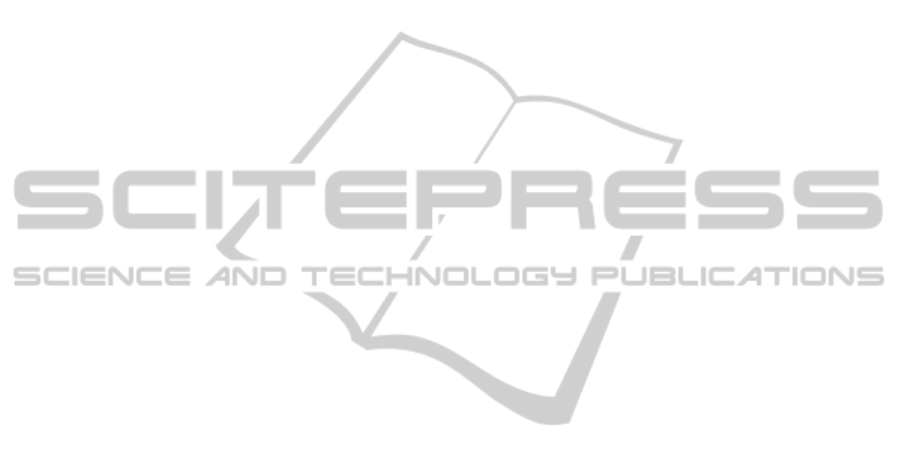
iments do not overlap, see figure 1. In our interpre-
tation this indicates the inadequacy of this approach.
However the model fits remarkably to individual ex-
periments, we would like to point out that this only
proves the models flexibility to capture the different
sigmoidal shapes.
By introducing a constant to the model, propor-
tional to the initial glucose bolus we managed to
achieve a good overall fit of the model with the same
set of parameters on different experiments (figure 2).
The trade-off is that MSE values to particular exper-
iments are moderately high. We found that our ap-
proach was adequate in fitting several experiments.
The random variables in the objective function re-
sulted that the algorithm is dynamically changing the
weights between the experiments, and ensures a good
convergence even if the sum of the error surfaces
would get difficult.
The sigmoid shape of the glucose uptake was found
slightly varying on the different experiments. This
might be a consequence of the different activity of
the glucose uptake systems revealed by (Castro et al.,
2009), or the differences between the transport of the
glucose monomers. We are also aware of that the glu-
cose uptake might be influenced by other factors, for
example the biomass, a feedback mechanism from the
inside of the cell or the energy level of the cell accord-
ing to (Papagianni et al., 2007). The model could be
extended to involve these aspects.
5 CONCLUSIONS AND FUTURE
WORK
Here we introduced a model to Lactococcus lactis
glucose uptake, and an approach based on PSO to fit it
to three glucose perturbation experiments with differ-
ent glucose input. With our approach a good overall
fit was achieved to the data using one set of parame-
ters. We think that this could be a future way towards
unified modeling of data with different experimental
conditions.
We can not exclude that our model is not complete,
and additional terms might be missing from it. Our
future work will aim to identify these terms. We
are also considering the distinct modeling of the glu-
cose monomers and to extend the identification of the
model to aerobic conditions.
ACKNOWLEDGEMENTS
This work was supported by project DynaMo
(PTDC/EEA-ACR/69530/2006) FCT, Portugal.
REFERENCES
Ashyraliyev, M., Fomekong-Nanfack, Y., Kaandorp, J. a.,
and Blom, J. G. (2009). Systems biology: parameter
estimation for biochemical models. The FEBS jour-
nal, 276(4):886–902.
Castro, R., Neves, A. R., Fonseca, L. L., Pool, W. a., Kok,
J., Kuipers, O. P., and Santos, H. (2009). Charac-
terization of the individual glucose uptake systems
of Lactococcus lactis: mannose-PTS, cellobiose-PTS
and the novel GlcU permease. Molecular microbiol-
ogy, 71(3):795–806.
Daniels, B. C., Chen, Y.-J., Sethna, J. P., Gutenkunst, R. N.,
and Myers, C. R. (2008). Sloppiness, robustness, and
evolvability in systems biology. Current opinion in
biotechnology, 19(4):389–95.
Goel, G., Chou, I.-C., and Voit, E. (2008). System estima-
tion from metabolic time-series data. Bioinformatics,
24(21):2505.
Kennedy, J. and Eberhart, R. (1995). Particle swarm opti-
mization. of IEEE international conference on neural,
4:1942–1948.
Naval, P., Sison, L., and Mendoza, E. (2006). Metabolic
network parameter inference using particle swarm op-
timization. In International Conference on Molecular
Systems Biology, pages 3–4.
Neves, A. R., Pool, W. a., Kok, J., Kuipers, O. P., and San-
tos, H. (2005). Overview on sugar metabolism and its
control in Lactococcus lactis - the input from in vivo
NMR. FEMS microbiology reviews, 29(3):531–54.
Papagianni, M., Avramidis, N., and Filiousis, G. (2007).
Glycolysis and the regulation of glucose transport in
Lactococcus lactis spp. lactis in batch and fed-batch
culture. Microbial cell factories, 6:16.
Srinath, S. and Gunawan, R. (2010). Parameter identifiabil-
ity of power-law biochemical system models. Journal
of biotechnology.
Vilela, M., Vinga, S., Maia, M. a. G. M., Voit, E. O., and
Almeida, J. S. (2009). Identification of neutral bio-
chemical network models from time series data. BMC
systems biology, 3:47.
Vinga, S., Thomaseth, K., Lemos, J., Neves, A., Santos,
H., and Freitas, A. (2008). Structural analysis of
metabolic networks: a case study on lactococcus lac-
tis. In 8th Portuguese Conference on Automatic Con-
trol (CONTROLO2008), pages 566–571.
Voit, E., Neves, A., and Santos, H. (2006). The intricate
side of systems biology. Proceedings of the National
Academy of Sciences, 103(25):9452.
BIOINFORMATICS 2011 - International Conference on Bioinformatics Models, Methods and Algorithms
312