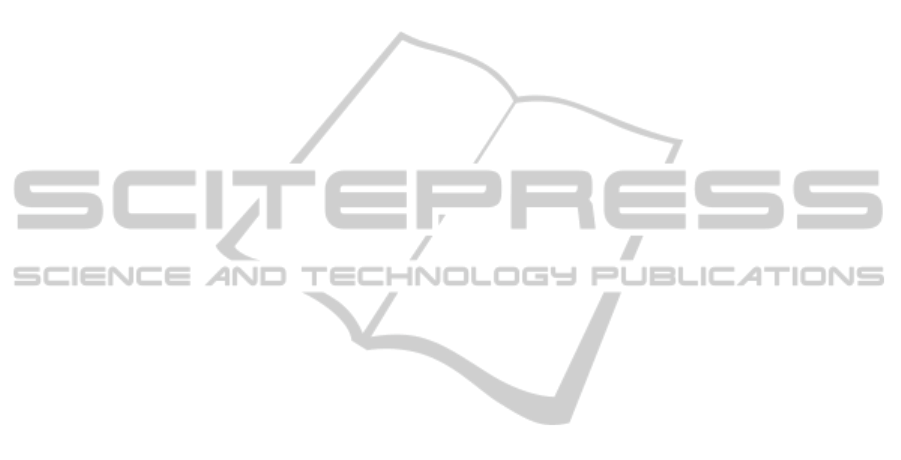
pipeline method (Dale et al., 1999; Hahn and Peitgen,
2000) introduced in FreeSurfer (Martinos Center for
Biomedical Imaging, USA) is applied.
The segmentation stage allows to extract the
topology of gray/white matter with respect to the po-
sition of each electrode.
3 RESULTS
Four patients underwent MR imaging studies prior to
depth electrode placement (the number of multicap-
tors for the first patient was 10, for the second - 11,
the third - 9, and the fourth - 8), altogether 456 elec-
trodes. In those 4 cases CT scan was made to track
the electrode positions. In all CT scans, electrodes
were noticeably blurred and artifacts of wires, hold-
ing frames, were visible. Successful skull stripping
was carried out. After applying correlation with pat-
tern to CT, not only true but also many false electrode
points were calculated. Mainly the false maximum
were located in the area of end of the multicaptors
and in the headholders. Nevertheless, all multicap-
tors were found and center of electrodes were located.
Due to the end of the multicaptor artifact, the algo-
rithm provides 14 false electrodes additionally. How-
ever, for each multicaptor, the number of electrodes
is known, and false electrodes can be eliminated au-
tomatically. Once the electrodes had been identified,
each patient’s MRI and CT co-registration were com-
puted, and then, transformations of the electrode po-
sitions were calculated. Finally, matter segmentation
was applied respectfully of the gray/white matter.
4 CONCLUSIONS
The electrode localization in the matter can be ap-
plied automatically (except in few minor cases at seg-
mentation stage). A electrode recognition in CT scan
image, and a register of MRI together with CT was
done. Lastly we segment the matter and calculate the
electrode’s position in the brain matter. We put for-
ward a new approach for automatic electrode local-
ization in CT and used some already developed tech-
niques which presented the full circle of automatic
depth electrode localization in the brain matter. This
proposed method is preprocessing stage of forward
modeling within the framework of electrophysiologi-
cal propagation in cerebral structures.
REFERENCES
Bricq, S., Collet, C., and Armspach, J. (2008). Unify-
ing framework for multimodal brain MRI segmenta-
tion based on Hidden Markov Chains. Medical Image
Analysis, 12(6):639–652.
Collignon, A., Maes, F., Delaere, D., Vandermeulen, D.,
Suetens, P., and Marchal, G. (1995). Automated
multi-modality image registration based on informa-
tion theory. Information Processing in Medical Imag-
ing, 1(1):263–274.
Dale, A., Fischl, B., and Sereno, M. (1999). Cortical
surface-based analysis. i. segmentation and surface re-
construction. Neuroimage, 9:179–194.
Dugas-Phocion, G., Ballester, M., Malandain, G., Lebrun,
C., and Ayache, N. (2004). Improved EM-based tis-
sue segmentation and partial volume effect quantifi-
cation in multi-sequence brain MRI. Medical Im-
age Computing and Computer-Assisted Intervention,
pages 26–33.
Duncan, J., Papademetris, X., Yang, J., Jackowski, M.,
Zeng, X., and Staib, L. (2004). Geometric strategies
for neuroanatomic analysis from mri. Neuroimage,
23:34–45.
Ekstrom, A., Suthana, N., Behnke, E., Salamon, N.,
Bookheimer, S., and Fried, I. (2009). High-resolution
depth electrode localization and imaging in patients
with pharmacologically intractable epilepsy. Stereo-
tactic and Functional Neurosurgery, 4(108):812–815.
Hahn, H. and Peitgen, H. (2000). The skull stripping prob-
lem in mri solved by a single 3d watershed transform.
Medical image computing and computer-assisted in-
tervention, 1935:134–143.
Lee, T., Fauzi, M., and Komiya, R. (2008). Segmentation
of ct head images. BioMedical Engineering and In-
formatics, 2:233–237.
Maldjiana, J., Chalelaa, J., Kasnera, S., Liebeskinda, D.,
and Detrea, J. (2001). Automated ct segmentation and
analysis for acute middle cerebral artery stroke. Amer-
ican Journal of Neuroradiology, 22:1050–1055.
Miller, K., Makeig, S., Hebbb, A., Raoc, R., Dennijs, M.,
and Ojemannb, J. (2007). Cortical electrode local-
ization from x-rays and simple mapping for electro-
corticographic research: The location on cortex (loc)
package for matlab. Journal of Neuroscience Meth-
ods, 162:303–308.
Oya, H., Kawasaki, H., Dahdaleh, N., Wemmie, J., and
Howard, M. (2009). Stereotactic atlas-based depth
electrode localization in the human amygdala. Stereo-
tactic and Functional Neurosurgery, 4(87):219–228.
Talairach, J., Bancoud, J., Szikla, G., Bonis, A., Geier, S.,
and Vedrenne, C. (1974). New approach to the neu-
rosurgery of epilepsy. stereotaxic methodology and
therapeutic results. 1. introduction and history. Neu-
rochirurgie, 20:1–240.
Wells, W., Viola, P., Atsumi, H., Nakajima, S., and Kiki-
nis, R. (1996). Multi-modal volume registration by
maximisation of mutual information. Medical Image
Analysis, 1(1):35–51.
BIOSIGNALS 2011 - International Conference on Bio-inspired Systems and Signal Processing
462