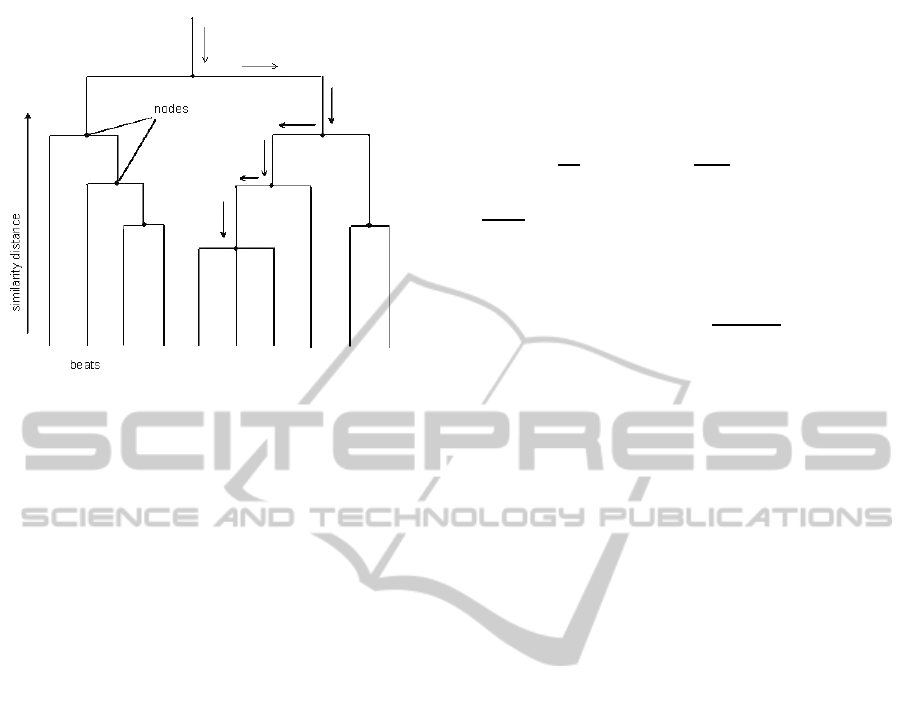
Figure 4: Schematic illustration of a dendrogram which is
the graphical representation of the cluster analysis. Follow-
ing the arrows at each node, the largest group of beats with
the smallest similarity distance is found and thus the start-
ing point for function SNR. The horizontal axis represents
the observations (beats), the vertical axis gives the distance
(or dissimilarity measure).
(Figure 4). For this reason, in order to include more
beats to the average procedure, the distance matrix D,
used in the cluster analysis, is weighted using the av-
erage of the most similar beats in the branch taken
into consideration. At each step the closest beat is
added. In this way a larger number of beats for the
average can be reached when compared to the stan-
dard CCA. Using these beats the averaging procedure
is performed. For further information related to CCA,
please refer to (Di Pietro Paolo et al., 2005).
3.4 Transformation to a Standardized
Sensor Configuration
A localized source
~
j positioned 15 cm below the sen-
sor 0 (center of the sensor system) is used for con-
version based on multipole expansion (ME). Param-
eters till octupole, as proposed by Burghoff et all,
(Burghoff et al., 2000) are used in Cartesian space.
Using these terms, it is possible to reconstruct a 55-
channel averaged signal. A ME is a series expansion
that can be used to represent the field produced by
a source (in this case heart) in terms of expansion pa-
rameters which become small as the distance from the
source increases. Katila and Karp (Katila and Karp,
1983) proved the possibility to describe the heart sig-
nal by expansion of the magnetic multipoles up to the
octupole term. It is interesting to note that the mag-
netic dipolar term serves as a good approximation in
the early ventricular activation (Trontelj et al., 1991).
By adding more terms (quadrupoles and octupole) a
very accurate reconstruction of the sources can be ob-
tained. The magnetic multipole expansion as the fol-
lowing form:
B(~r) = −
µ
0
4π
Re
∞
∑
n=0
∞
∑
m=0
∇
1
r
n+1
P
m
n
(cosθ)e
−imζ
(1)
×
γ
mn
n+ 1
Z
∇
′
h
r
′
n
P
m
n
cosθ
′
e
imζ
′
i
x,~r
′
~
j
r
′
dv
′
Here are γ
mn
the coefficients so that (Katila and Karp,
1983):
γ
mn
= [2− δ(m)]
(n− m)!
(n+ m)!
with P
m
n
(cosθ) the associated Legendre functions of
first kind. The current density
~
j in the expression 1
is the total current density over the volume v
′
and the
expansion is valid for the region lying outside the sur-
face S
′
, i.e. r should exceed the largest value of r
′
.
4 RESULTS
The preprocessing is applied to the 20 acquisitions.
The performances of the preprocessing in terms of
noise reduction are measured using the Root Mean
Square (RMS) before and after the application of
CCA and transformation to a standard sensor con-
figuration. The SNR has been applied on the av-
eraged magnetic signals in the segment [T-end, P-
onset], since in this region the signal amplitude is usu-
ally very low. A summary of the results is shown in
table 1. The application of CCA and ME improved
the SNR of the averaged magnetic signals in almost
all cases up to 90%. Only in one case the preprocess-
ing was not successful. Examples of averaged signals
are shown in Figure 5.
5 DISCUSSION AND
CONCLUSIONS
The preprocessing of MFI data outlined here is the
basis for all specific analysis that can be performed
with the MFI software of Apollo CXS (QRS frag-
mentation analysis, digital subtraction MFI for stress
induced ischemia detection etc). It has been shown
that combining a carefully designed low cost shielded
room (patent DE 10 2007 017 316 B4) with a gra-
diometric sensor system it is possible to obtain data
usable in clinical environment. Furthermore, the use
of a dedicated algorithm for the averaging procedure
PREPROCESSING IN MAGNETIC FIELD IMAGING DATA
465