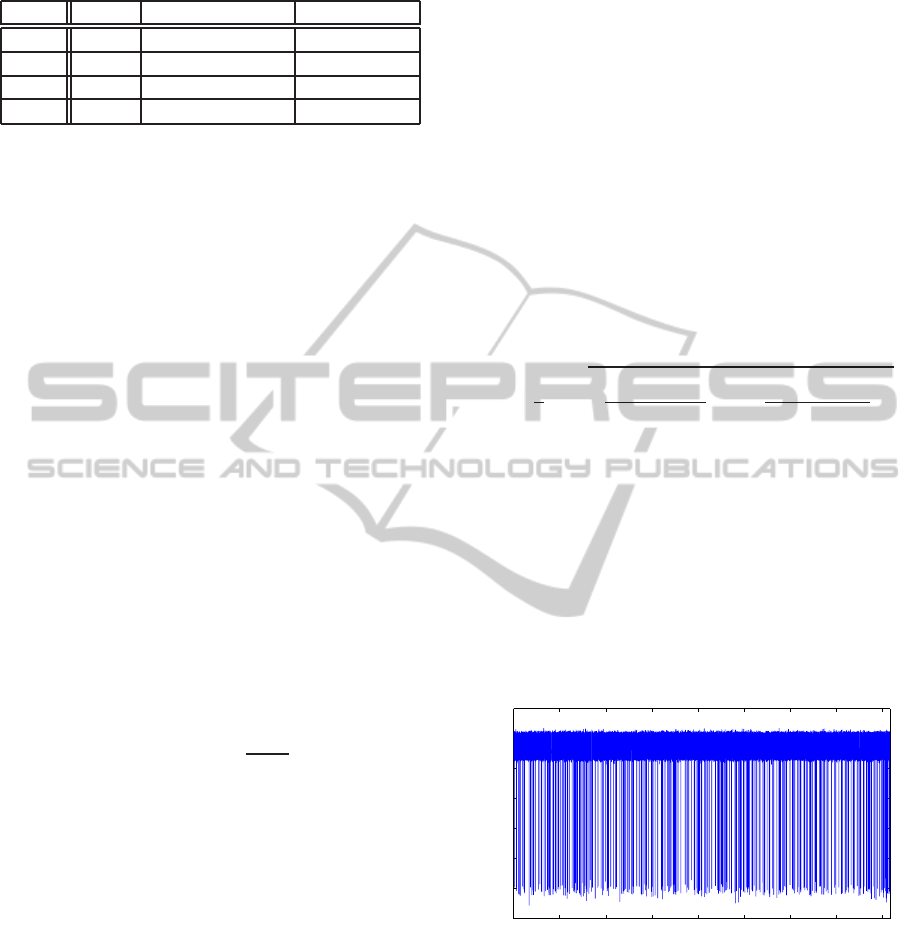
Table 1: Distributions features.
Dist param. 1 param. 2
NTS Gaus. µ = 93.7 pA σ = 1 pA
AMP Gaus. µ = 89.2 pA σ = 7.33 pA
DUR Ray. r = 924 µs
DBT Exp. α = (17.6 ms)
−1
3 PERFORMANCES OF A
NO-PARAMETRIC
AMPLITUDE-DURATION
ESTIMATION ALGORITHM
In this section, the DNA translocation signal charac-
terization results are used to evaluate, in a stochas-
tic framework, the performances of an elementary
translocation characterization algorithm. The con-
sidered algorithm is based on a breakdown detec-
tion technique, presented in (Osaki et al., 2010)
which allows to estimate the amplitude and duration
of translocation events, respectively denoted
[
AMP
and
[
DUR. To evaluate the performances of this char-
acterization algorithm for various signal features, we
build up artificial biomimetic signal considering the
AMP, DUR, DBT and NTS distributions estimated in
the previous section. Moreover, in order to take the
possible experimental noise variance variations into
account relative to the experimental set-up, we elab-
orate artificial signals featured by various signal to
noise ratios (SNR), defined as:
SNR = 20log|
µ
AMP
σ
NTS
| (8)
An example of a 319 translocation signal se-
quence featured by a 30 dB SNR is represented in
figure 8, and figure 9 exhibits the detail of a sin-
gle artificial translocation event. The implementation
of the breakdown detection algorithm applied to this
translocation sequence allows the AMP and DUR val-
ues of the 319 translocations to be estimated. The cor-
responding amplitude vs duration representation dia-
gram is depicted in figure 10.
In order to quantify the characterization perfor-
mances of the algorithm, we compute the true posi-
tive rate and the false positive rate of the character-
ization algorithm, considering SNRs ranging from 6
to 46 dB. The true positive rate (TPR) is computed
as the rate of the estimated AMP-DUR values of each
considered translocation event which are close to the
actual values whithin a predifined distance ν. On
the other hand, the false positive rate (FPR) is de-
fined as the rate of the estimated AMP-DUR values
which are wrongly positionned at a distance smaller
than a varying distance ν. Then, the receiver opera-
tional characteristic (ROC) which plots the TPR as a
function of FPR for various values of ν can be con-
sidered to quantify the characterization performances
(Bradley, 1997). ROC curves obtained for the con-
sidered translocations data are presented in figure 11.
One can note for example that a 90 % TPR is reached
at the cost of a 0.01 % FPR considering a transloca-
tion sequence with SNR = 28 dB, and that the same 90
% TPR is reached at the cost of a 1 % FPR when the
SNR falls down to 18 dB. An other means of quanti-
fying the performance of the amplitude-duration esti-
mation algorithm is to evaluate the mean square error
(MSE) defined in equation (9), of the characterization
as a function of the SNR of the translocation signal.
MSE =
1
n
n
∑
i=1
v
u
u
t
[
AMP
i
−AMP
i
µ
AMP
!
2
+
[
DUR
i
−DUR
i
µ
DUR
!
2
(9)
where
[
AMP and
[
DUR are the estimated values
of AMP and DUR respectively, n is the number of
translocations equal to 319, and where the contribu-
tion of the amplitude and duration errors are normal-
ized by their mean values in order to give them the
same weight in the computation of the MSE. The
MSE computed according to equation (9) and ex-
pressed in percent is represented in figure 12. One can
note that the MSE falls from 30 % to 0.02 % when the
SNR rises from 6 dB up to 46 dB, respectively.
Time (sec)
Current amplitude (pA)
0
10
20
30
40
50 60 70 80
-20
0
20
40
60
80
100
120
Figure 8: Generated artificial signal.
4 DISCUSSION
We have proposed a statistical characterization of
nanopore DNA translocation current allowing well
known methods of amplitude/duration characteriza-
tions (Basseville and Nikiforov, 1993) to be imple-
mented and evaluated using intensive computer simu-
lations.
BIOSIGNALS 2011 - International Conference on Bio-inspired Systems and Signal Processing
224