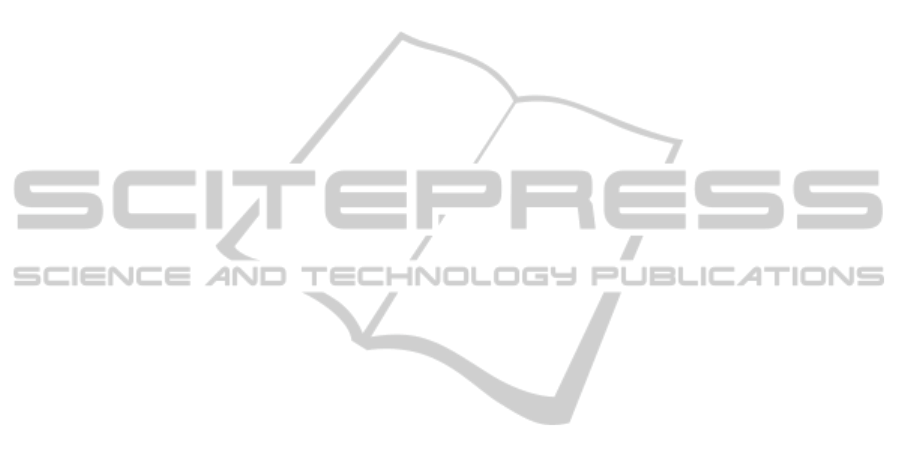
needs.
Different strategies could be applied to
implement the proposed knowledge-based model.
Some criteria for evaluating alternatives include
among others: easy representation of prior
knowledge statements, simple structure and
maintenance of facts bases, straightforward
definition of rules and reasoning mechanisms,
portability, interoperability of involved software
components, feasible scalable development, rich
data visualization resources, and web services
deployment. The decision may involve using
different specialized programming languages in
order to optimize specific modules, and in that case
the model implementation would be an embedded
engine of a larger distributed system.
Envisioned applications and services that could
make use of the proposed data integration model are
mainly related to wellness monitoring and disease
management. Given the richness of possible
integrated data analysis in these contexts, DS queries
must be controlled, precise, and constrained to
verified processing methods. For this reason,
available validated data sometimes may not be
relevant to answer a DS request, and the system
should be capable, thanks to the knowledge-based
model, to opportunely inform about such situation.
However, knowledge definitions are flexible enough
to be independently adapted at the three levels of the
model, and completed with other rules.
6 CONCLUSIONS
Regardless of the multiple ways to collect and
structure physiological sensors data and patient
observations, integration of these data for combined
analysis and interpretation requires embedded
knowledge at different levels. We formulated a
preliminary proposal towards the integration of
complex relationships between various physiological
sensors data and patient observations, in order to
produce goal oriented processing workflows. The
resulting knowledge-based data integration model is
intended to define how to process PHR data,
applying comprehensive dedicated knowledge
clusters, to validate goal oriented inferences. Further
work concerns the development and evaluation of a
complete prototype including clinical data from the
EHR, as well as the assessment of DS results for
specific use cases. Being an initial attempt to
formulate a general methodology, multiple open
questions remain beyond the conception of a
working system, like acceptance, performance, and
usability.
ACKNOWLEDGEMENTS
This work was supported in part by Telecom
Bretagne and in part by VTT and the Finnish
Funding Agency for Technology and Innovation
(Tekes) in the framework of the ITEA2/Care4Me
project.
REFERENCES
Garg, M. K., Kim, D-J., Turaga, D. S., Prabhakaran, B.,
2010. Multimodal analysis of body sensor network
data streams for real-time healthcare. In Proc. ACM
SIGMM International Conference on Multimedia
Information Retrieval, Philadelphia, USA, pp. 469-
478.
Halamka, J. D., Mandl, K. D., Tang, P. C., 2008. Early
experiences with personal health records. Journal of
the American Medical Informatics Association, vol.
15, no. 1, pp. 1-7.
Han, J., Kamber, M., 2006. Data Mining: Concepts and
Techniques. Morgan Kaufmann Series in Data
Management Systems, 2
nd
edition, pp. 467-488.
IEEE Health Informatics, 2009. Personal Health Device
Communication, Part 11073-10441: Device
Specialization - Cardiovascular Fitness and Activity
Monitor. IEEE Engineering in Medicine and Biology
Society, 11073™ Standard Committee, 85 pp.
Jovanov, E., Poon, C., Yang, G-Z., Zhang, Y. T., 2009.
Body sensor networks: from theory to emerging
applications. Guest Editorial. IEEE Transactions on
Information Technology in Biomedicine, vol. 13, no. 6,
pp. 859-863.
Kulkarni, P., Öztürk, Y., 2007. Requirements and design
spaces of mobile medical care. ACM SIGMOBILE
Mobile Computing and Communications Review, vol.
11, no. 3, pp. 12-30.
Martínez-López, R., Millán-Ruiz, D., Martín-Domínguez,
A., Toro-Escudero, M. A., 2008. An architecture for
next-generation of telecare systems using ontologies,
rules engines and data mining. In Proc. CIMCA,
IAWTIC, and ISE International Conferences, Vienna,
Austria, pp. 31-36.
Stuntebeck, E. P., Davis II, J. S., Abowd, G. D., Blount,
M., 2008. HealthSense: classification of health-related
sensor data through user-assisted machine learning. In
Proc. 9
th
workshop on Mobile Computing Systems and
Applications, Napa Valley, USA, pp.1-5.
Tang, P. C., Ash, J. S., Bates, D. W., Overhage, J. M.,
Sands, D. Z., 2006. Personal health records:
definitions, benefits, and strategies for overcoming
barriers to adoption. Journal of the American Medical
Informatics Association, vol. 13, no. 2, pp. 121-126.
TOWARDS KNOWLEDGE-BASED INTEGRATION OF PERSONAL HEALTH RECORD DATA FROM SENSORS
AND PATIENT OBSERVATIONS
285