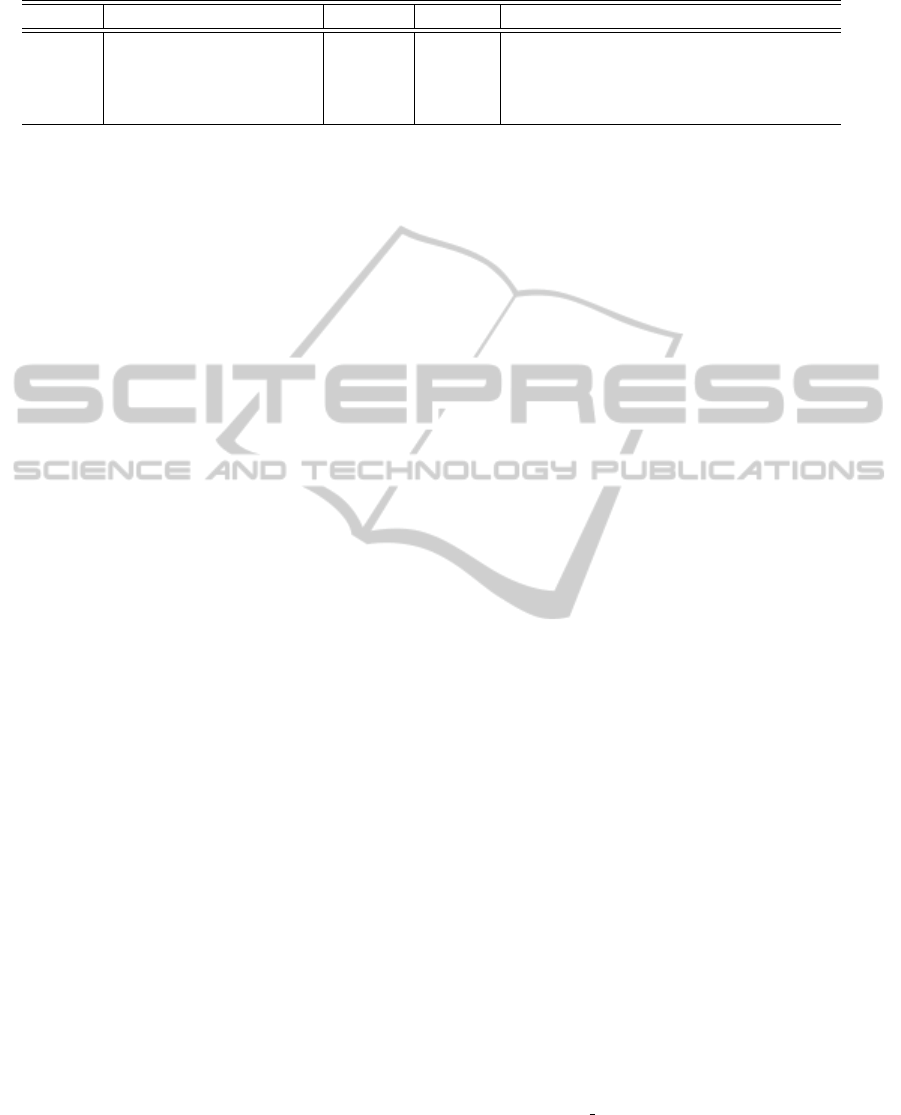
Table 3: Classification performances of the two feature selection methods compared to previously reported feature choices.
Model Feature selection Features BCR N S V F
wLDA Wrapper wLDA 2 73.00% 81.88% 70.53% 70.77% 68.81%
wLDA (Chazal et al., 2004) 50 73.83% 88.63% 44.66% 80.58% 81.44%
wSVM Ranking MI 6 82.99% 75.88% 82.63% 85.06% 88.40%
wSVM (De Lannoy et al., 2010) 36 71.55% 77.54% 42.86% 79.19% 86.60%
the weighted SVM classifier and the MI criteria to
score the features. Six features are empirically se-
lected from the ranking results. Results with the
weighted SVM classifier using only these 6 features
are significantly higher than the performances with
the same model using previously reported feature
choices with up to 36 features. In particular, the accu-
racy for the S class is improved by almost 40%. The
six selected features are the normalized previous R-R
interval, the normalized height of the T-wave and four
high order statistics.
ACKNOWLEDGEMENTS
G. Doquire and G. de Lannoy are funded by a Belgian
F.R.I.A grant.
REFERENCES
Association for the Advancement of Medical Instrumenta-
tion (1998). Testing and reporting performance results
of cardiac rhythm and st segment measurement algo-
rithms. ANSI/AAMI EC38:1998.
Chazal, P. D., O’Dwyer, M., and Reilly, R. B. (2004). Auto-
matic classification of heartbeats using ecg morphol-
ogy and heartbeat interval features. Biomedical Engi-
neering, IEEE Transactions on, 51:1196–1206.
Christov, I., G
´
omez-Herrero, G., Krasteva, V., Jekova, I.,
Gotchev, A., and Egiazarian, K. (2006). Comparative
study of morphological and time-frequency ecg de-
scriptors for heartbeat classification. Med. Eng. Phys.,
28(9):876–887.
De Lannoy, G., Francois, D., Delbeke, J., and Verleysen, M.
(2010). Weighted svms and feature relevance assess-
ment in supervised heart beat classification. Commu-
nications in Computer and Information Science (Se-
lected and extended papers of the BIOSIGNALS2010
conference), TO APPEAR.
Franc¸ois, D. (2008). Feature selection. In Wang, J., ed-
itor, Encyclopedia of data mining and warehousing,
second edition, Information Science Reference. Idea
Group Publishing.
Goldberger, A., Amaral, L., Glass, L., Hausdorff, J., Ivanov,
P. C., Mark, R., Mietus, J., Moody, G., Peng, C.-K.,
and Stanley, H. (2000). PhysioBank, PhysioToolkit,
and PhysioNet: Components of a new research re-
source for complex physiologic signals. Circulation,
101(23):e215–e220.
Gomez-Verdejo, V., Verleysen, M., and Fleury, J. (2009).
Information-theoretic feature selection for functional
data classification. NEUROCOMPUTING, 72(16-18,
Sp. Iss. SI):3580–3589.
Lagerholm, M., Peterson, C., Braccini, G., Edenbrandt,
L., and Sornmo, L. (2000). Clustering ecg com-
plexes using hermite functions and self-organizing
maps. Biomedical Engineering, IEEE Transactions
on, 47(7):838–848.
Llamedo-Soria, M. and Martinez, J. (2007). An ecg classi-
fication model based on multilead wavelet transform
features”. In Computers in Cardiology, volume 35.
Melgani, F. and Bazi, Y. (2008). Classification of electro-
cardiogram signals with support vector machines and
particle swarm optimization. Information Technology
in Biomedicine, IEEE Transactions on, 12(5):667–
677.
Moddemeijer, R. (1989). On estimation of entropy and mu-
tual information of continuous distributions. Signal
Processing, 16(3):233–246.
Nguyen, G. H., Bouzerdoum, A., and L., P. S. (2009).
Learning Pattern Classification Tasks with Imbal-
anced Data Sets. INTECH.
Osowski, S. and Hoai, L. (2001). Ecg beat recognition us-
ing fuzzy hybrid neural network. Biomedical Engi-
neering, IEEE Transactions on, 48(11):1265–1271.
Osowski, S., Hoai, L., and Markiewicz, T. (2004). Sup-
port vector machine-based expert system for reliable
heartbeat recognition. Biomedical Engineering, IEEE
Transactions on, 51(4):582–589.
Park, K., Cho, B., Lee, D., Song, S., Lee, J., Chee, Y., Kim,
I., and Kim, S. (2008). Hierarchical support vector
machine based heartbeat classification using higher
order statistics and hermite basis function. In Com-
puters in Cardiology, pages 229–232.
Shannon, C. E. (1948). A mathematical theory of com-
munication. Bell Systems Technical Journal, 27:379–
423,623–656.
Steuer, R., Kurths, J., Daub, C. O., Weise, J., and Selbig, J.
(2002). The mutual information: Detecting and eval-
uating dependencies between variables. Bioinformat-
ics, 18(suppl 2):S231–240.
FEATURE SELECTION FOR INTER-PATIENT SUPERVISED HEART BEAT CLASSIFICATION
73