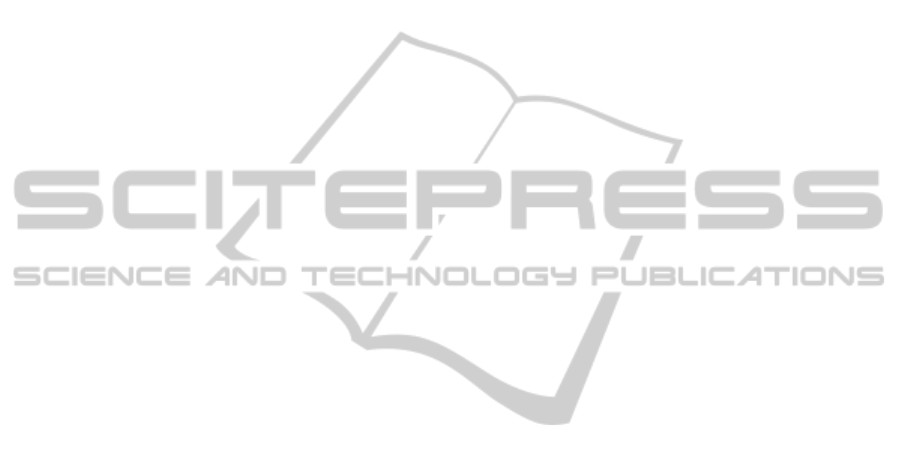
reshaping to form the other activity and the distance
value to the mean wave or to the clusters mean
values is bigger than anywhere else on the signal.
This occurred in the jumps and in the walk and run
activities.
Given the results we can affirm that our
clustering algorithm based on the mean wave
information only returned 7 errors out of 999 cycles
with pattern quality, and therefore we achieved
99.2% of efficiency.
5 CONCLUSIONS
The proposed algorithm represents an advance in the
abstract clustering area, as it has an effective
detection of signal variations, tracing different
patterns for distinct clusters, whether it’s an activity,
synthetic or physiological signal.
6 FUTURE WORK
In future work we intend to repeat this procedure to
a wide range of subjects performing the same task,
perform a noise immunity test and also run the
algorithm using a signal with more than two modes.
We intend to introduce an automatically
perception of the cycles which are too distance from
the cluster and assign those cycles to a new
“rejection class”. This will reduce the number of
errors due to a strange cycle, in particular the
mode’s transition cycles.
The local detection of the fundamental frequency
is also a future goal, as we intend to realize when
there’s a major variation of fundamental frequency
and make our algorithms adapt its behavior
according to that variation.
Finally, we have the intention of creating a
multimodal algorithm, which can receive more than
one signal, and process those at the same time and
with the same treatment. This could be useful if we
want to use the 3 axis of an accelerometer, or
conciliate the information of a BVP with an
electrocardiography (ECG) signal.
ACKNOWLEDGEMENTS
The authors would like to thank PLUX – Wireless
Biosignals for providing the acquisition system and
sensors necessary to this investigation. We also like
to thank NIH, the Norwegian School of Sports and
Science, Håvard Myklebust and Jostein Hallén for
acquiring and allowing us to work with the Skiing
signal used in this study. We acknowledge Rui
Martins and José Medeiros for their help and advices
on the BVP acquisition procedure.
REFERENCES
Andersson, E., Supej, M., Sandbakk, Ø., Sperlich, B.,
Stöggl, T., Holmberg, H. (2010) Analysis of sprint
cross-country skiing using a differential global
navigation satellite system. Eur J Appl Physiol. DOI
10.1007/s00421-010-1535-2
Baek, J., Lee G., Park, W., Yun, B. (2004). Accelerometer
Signal Processing for User Activity Detection,
Knowledge-Based Intelligent Information &
Engineering Systems, Vol. 3215/2004, 610-617. DOI
10.1007/978-3-540-30134-9_82
Ben-Arie, J., Wang, Z., Pandit, P., Rajaram, S. (2002).
Human Activity Recognition Using Multidimensional
Indexing. IEEE Transactions on Pattern Analysis and
Machine Intelligence, vol. 24, no. 8, 1091-1104.
Fridlund, A., Schwartz, G., Fowler, S. (2007) Pattern
Recognition of Self-Reported Emotional State from
Multiple-Site Facial EMG Activity During Affective
Imagery. Society for Psychophysiological Research.,
vol. 21, no. 6. DOI 10.1111/j.1469-
8986.1984.tb00249.x
Liao, T. Warren. (2005) Clustering of time series data – a
survey. Pattern Recognition 38 (2005) 1857 – 1874.
Martins, D., Mattos, M., Simões, P., Cechinel, C., Bettiol,
J.; Barbosa, A. (2008) Aplicação do Algoritmo K-
Means em Dados de Prevalência da Asma e Rinite em
Escolares. In: XI Congresso Brasileiro de Informática
em Saúde (CBIS'2008), 2008.
PLUX – Wireless Biosignals, bioPLUX Research Manual,
PLUX’s internal report, 2010.
Quiroga, Rodrigo Q. (2007) Spike sorting. Scholarpedia,
2(12):3583
Smith, J., Fishkin, K., Jiang, B., Mamishev, A., Philipose,
M., Rea, A., Roy, S., Sundara-Rajan, K. (2005).
RFID-Based Techniques for Human-Activity
Detection. Communications of the ACM, vol. 48, no.
9, 39-44.
T. Oliphant. Guide to Numpy. Tregol Publishing, 2006.
T. Oliphant. SciPy Tutorial. SciPy, http://www.scipy.org/
SciPy Tutorial, 2007.
Wu, X., Kumar, V., Quinlan J., Ghosh J., Yang, Q.,
Motoda, H., McLachlan, G., Ng, A., Liu, B., Yu, P.,
Zhou, Z., Steinbach, M., Hand, D., Steinberg, D.
(2007). Top 10 algorithms in data mining. Knowledge
Information Systems, 14:1–37. DOI 10.1007/s10115-
007-0114-2
www.opensignals.net, last accessed on 15/07/2010
BIOSIGNALS 2011 - International Conference on Bio-inspired Systems and Signal Processing
264