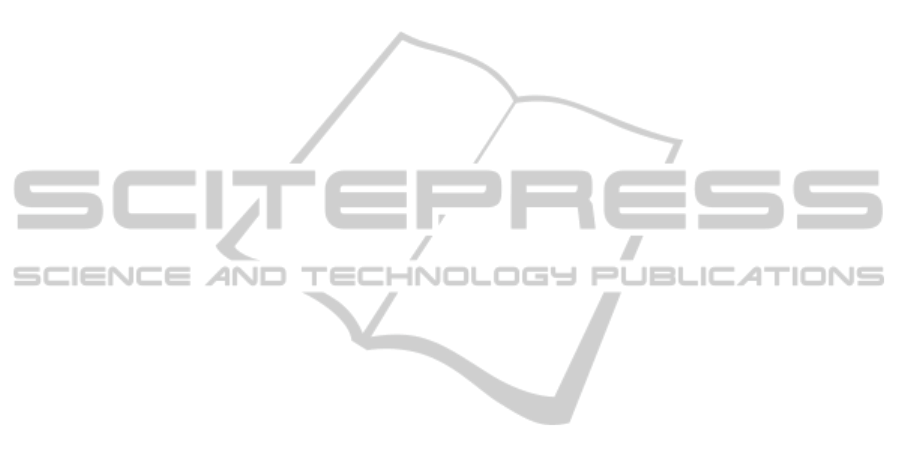
Gauvain, J.-L. and Lee, C.-H. (1994). Maximum a poste-
riori estimation for multivariate gaussian mixture ob-
servations of markov chains. IEEE Transactions on
Speech and Audio Processing, 2(2):291–298.
Geurts, P. (2001). Pattern extraction for time-series clas-
sification. Lecture Notes in Artificial Intelligence,
2168:115–127.
Inoue, K. (1992). Linear resolution for consequence find-
ing. Artificial Intelligence, 56:301–353.
Inoue, K. (2004). Induction as consequence finding. Ma-
chine Learning, 55:109–135.
Inoue, K., Sato, T., Ishihata, M., Kameya, Y., and
Nabeshima, H. (2009). Evaluating abductive hypothe-
ses using and EM algorithm on BDDs. In Proc. of
IJCAI-09, pages 820–815. AAAI Press.
Ishihata, M., Kameya, Y., Sato, T., and Minato, S. (2008).
Propositionalizing the EM algorith by BDDs. Tech-
nical report, TR08-0004, Dept. Comp. Sc., Tokyo In-
stute of Technology.
Ji, S., Krishnapuram, B., and Carin, L. (2006). Varia-
tional bayes for continuous hidden markov models
and its application to active learning. IEEE Transac-
tions on Pattern Analysis and Machine Intelligence,
28(4):522–532.
Juvan, P., Demsar, J., Shaulsky, G., and Zupan, B. (2005).
Genepath: from mutations to genetic networks and
back. Nucleic Acids Res., 33.
Kanehisa, M., Araki, M., Goto, S., Hattori, M., Hirakawa,
M., Itoh, M., Katayama, T., Kawashima, S., Okuda,
S., Tokimatsu, T., and Yamanishi, Y. (2008). KEGG
for linking genomes to life and the environment. Nu-
cleic Acids Res., 36:480–484.
Kanehisa, M. and Goto, S. (2000). Kyoto encyclopedia of
genes and genomes. Nucleic Acids Res., 28(1):27–30.
Keogh, E., Lin, J., and Fu, A. (2005). HOT SAX: efficiently
finding the most unusual time series subsequence. In
5th IEEE International Conference on Data Mining.
King, R., Garrett, S., and Coghill, G. (2005). On the
use of qualitative reasoning to simulate and iden-
tify metabolic pathways. Bioinformatics, 21(9):2017–
2026.
King, R., Whelan, K., Jones, F., Reiser, P., Bryant, C., Mug-
gleton, S., Kell, D., and Olivier, S. (2004). Functional
genomic hypothesis generation and experimentation
by a robot scientist. Nature, 427:247–252.
Kitano, H. (2002). Systems biology toward system-
level understanding of biological systems. Science,
295(5560):1662–1664.
Mooney, R. (1997). Integrating abduction and induction in
machine learning. In Working Notes of the IJCAI97
Workshop on Abduction and Induction in AI, pages
37–42.
Muggleton, S. (1995). Inverse entailment and progol. New
Generation Computing, 13(3/4):245–286.
Nabeshima, H., Iwanuma, K., and Inoue, K. (2003). SO-
LAR: A consequence finding system for advanced
reasoning. In Proc. of the 11th International Con-
ference TABLEAUX 2003, LNAI, volume 2786, pages
257–263.
Peters-Wendisch, P., Schiel, B., Wendisch, V., and et al.,
E. K. (2001). Pyruvate carboxylase is a major
bottleneck for glutamate and lysine production by
corynebacterium glutamicum. Molecular Microbiol.
Biotechnol., 3(2).
Rabiner, L. (1989). A tutorial on hidden markov models
and selected applications in speech recognition. Proc.
of the IEEE, 77(2):257–286.
Ray, O., Whelan, K., and King, R. (2009). A nonmonotonic
logical approach for modelling and revising metabolic
networks. Complex, Intelligent and Software Intensive
Systems, IEEE.
Schwarz, G. (1978). Estimating the dimension of a model.
Annals of Statistics, 6(2):461–464.
Tamaddoni-Nezhad, A., Chaleil, R., Kakas, A., and Mug-
gleton, S. (2006). Application of abductive ILP to
learning metabolic network inhibition from temporal
data. Machine Learning, 64:209–230.
Tiwari, A., Talcott, C., Knapp, M., Lincoln, P., and Lader-
oute, K. (2007). Analyzing pathways using SAT-
based approaches. In Proc of the 2nd Int. Conf. on
Algebraic Biology, pages 155–169.
BIOINFORMATICS 2011 - International Conference on Bioinformatics Models, Methods and Algorithms
54