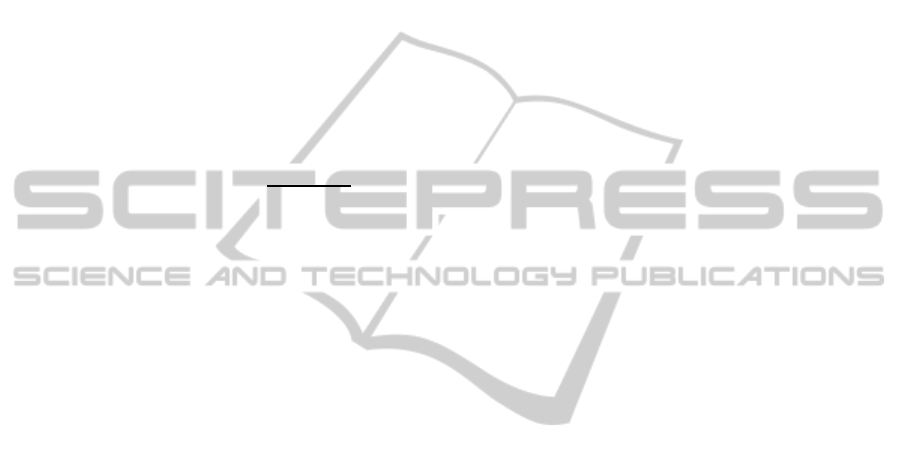
5.2 H2: MI is a Significant Term within
the Vioxx-related PML
There are a total of 4696 unique terms and 603 doc-
uments in our Vioxx-specific PML. The MI term oc-
curs a total of 82 times, ranking in the top 250 (5%)
most frequent terms in the corpus. Usually we might
consider these top ranking terms irrelevant because of
their high frequency; however, many common stop-
words have already been removedfrom the text by the
MetaMap UMLS mapping. Despite this, frequency is
a coarse measure of importance in text. In addition,
we construct a ranking of important terms within the
Vioxx-related PML. Our ranking is based on the term-
frequency inverse-document-frequency (tf-idf) met-
ric:
t f-id f (t, d) = t f(t, d) ∗ log
|D|
|D : t ∈ D|
where t f(t, d) is t’s normalized frequency in a doc-
ument d ∈ D, where D is the set of all corpus docu-
ments. Terms that occur frequently in a document, but
infrequently in the rest of the corpus, will get a high
tf-idf score, while common terms will get a low score.
Within a document d, tf-idf is intended to rank highly
those terms that “best describe that document”.
To obtain a set, S, of terms that are highly rele-
vant to the Vioxx-related corpus, we add the top 10%
of terms for each document by tf-idf ranking to S. For
example, if a document contained 50 terms, we would
add the top 5 tf-idf scored terms to S. Each element of
S is scored according to the number of documents for
which it was a top-10% tf-idf term. Although simple,
the technique is intuitive. While rare words will likely
score within the top 10% tf-idf ranked terms of some
document, only words truly descriptive of corpus seg-
ments should score within this range for several doc-
uments. Conversely, terms that occur so frequently as
to be meaningless should score within that range very
infrequently.
In the 3066 unique terms contained in S, MI is
ranked within the top 110 (3.3%) of highly relevant
terms in the corpus, and within the top 2.3% terms
in the corpus. In the same company are terms that
we would expect to rank highly, such as “arthri-
tis”, “pain”, and “NSAID”. There are also sev-
eral other suggestive terms. “Diethylstilbstrol” is
a non-steroidal drug that was withdrawn from the
market; “duodenal ulcer” relates to the problem that
Vioxx was supposed to solve (gastrointestinal com-
plications). Finally, a quick search for “vioxx” and
“deet” uncovers several articles comparing the danger
of the two drugs. However, the results also contained
irrelevant terms. “Text”, “document” and “stock”, for
example, are likely artifacts of HTML junk that the
MetaMap parser did not discard. Other terms, such
as “activity”, “wanted” and “include”, could likely be
filtered semantically.
6 DISCUSSION AND FUTURE
WORK
The results presented in Section 5.1 support our first
hypothesis: “Myocardial Infarction” is more signifi-
cant than expected in Vioxx-related articles. The MI
term occurs on average almost twice as often in the
Vioxx-related articles than in the control articles, as
shown in Table 1. Moreover, a non-parametric sta-
tistical test indicates with high confidence that the
frequency distribution of the MI term in the Vioxx-
related PML is significantly skewed to the right when
compared to that of the Naproxen and Ibuprofen-
related PML.
In light of the inter-drug PML significance of the
MI term, the results presented in Section 5.2 for-
tify both our first and second hypotheses. A sim-
ple method that, in essence, counted the number of
documents for which a word was highly descriptive,
ranked the MI term in the top 3.3% of the most rele-
vant words in the corpus. Cast in this light, our first
result, the fact that the difference in MI term distri-
bution between the corpora segments is statistically
significant, becomes even more relevant. Given these
results, we can claim with reasonable confidence that
the concept “Myocardial Infarction” was a distinctive
term in the Vioxx-related PML both within that litera-
ture itself, as well as across control PMLs, well before
Vioxx was withdrawn from the market. That is, not
only could MI could be tied to “Vioxx” as an impor-
tant, descriptive concept, but it could also be labeled
as an anomaly in that general class of PML. These
results are heartening for a proof-of-concept system.
We do note, however, that while 3.3% is an impres-
sive margin, 110 terms is still too many for a user to
browse through. Implementing an effective search in-
terface for potentially relevant links is one of the most
important components of future work.
Further improvements include expanding our
analyses to incorporate data sets containing overlap-
ping drug terms. Incorporating additional drugs into
the control corpus would provide more comparison
points. Finally, an important goal is to cultivate Web
content from alternative sources, such as Twitter feeds
and blog posts, into the corpus. Developing methods
for attaining, cleaning and analyzing these data will
prove challenging, but we believe that results will be
rewarding.
MINING THE WEB FOR MEDICAL HYPOTHESES - A Proof-of-Concept System
307