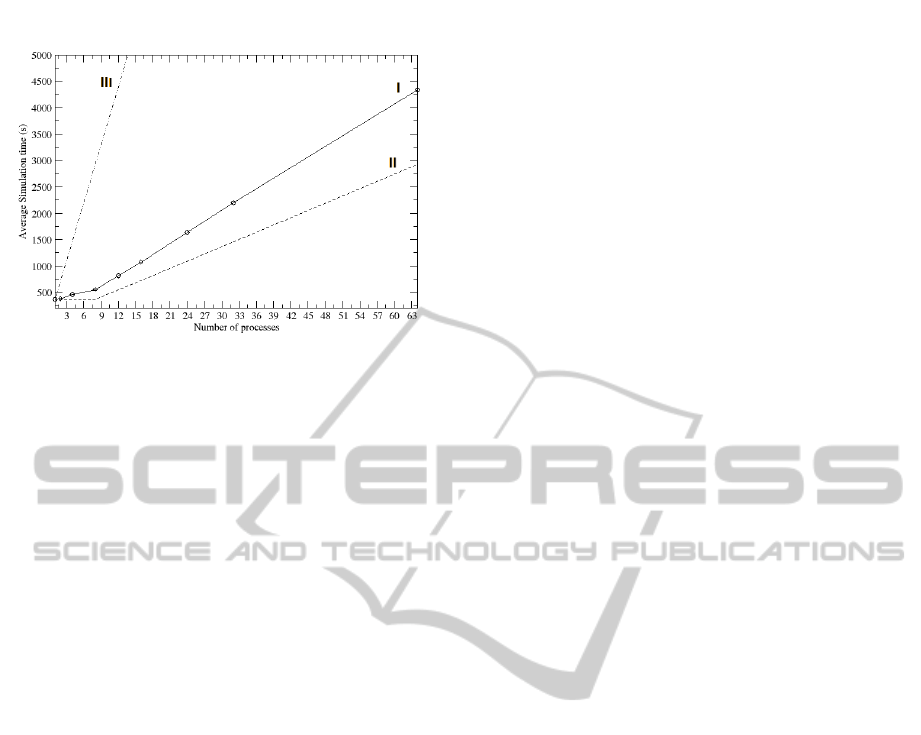
Figure 4: Trend of the simulation time at the increasing
of the running processes. Trace ’I’ and ’II’ are respec-
tively the real and the ideal trend for the simulation time
obtained by running an increasing number of parallel pro-
cesses, whereas trace ’III’ is that relative to an increasing
number of serial processes. The cycles on Trace ’I’ mark
the number of processes for which the test have been made.
regions, there is a considerable contention for access
to the L2 cache and the main memory. The latter
arises when the number of processes overcomes the
number of available CPUs. Indeed, it can be observed
that trace ’I’ is characterized by two different trends.
The first one, from 1 to 8 lambda, where only the
memory contention contributes. The second, from 8
to 64 lambda, where the context switch and process
migration overheads are also present, leading to an in-
creasing slope of the curve. The utilization is almost
100% before 8 lambda and decreases after 8 lambda,
where each process has to share the CPU with others.
It is worth nothing however that besides the overhead
discussed, the parallelization is unquestionably more
advantageous with respect to performing serial simu-
lations, whose simulation times are reported in Trace
’III’ of Figure 4. Overall, by putting together the
accuracy results with the computation costs, we can
conclude that a suitable trade-off between computa-
tion and accuracy can be set to 19 lambda.
4 CONCLUSIONS
The methodology presented in this paper has been de-
signed to face the various challenges of nucleic acid
simulations and binding free energy computations.
First, the positioning of the restraints has been deter-
mined to overcome the stability problems due to the
remarkable folding activity and the base pairs open-
ing of the complex. As a results, we were finally able
to perform MD simulations in stable conditions.
Second, the accuracy of the thermodynamic inte-
gration steps. On this concern, we studied the scala-
bility of the thermodinamic integration to determine a
reasonable trade-off between computation and accu-
racy. We shown that to achieve an error on the bind-
ing free energy computation lower than 4% we need
to perform 60 parallel simulations. On the other side,
a good trade-off between computation and accuracy
is already reached using 19 parallel simulations.
REFERENCES
Bartel, D. P. (2004). MicroRNAs: Genomics, Biogenesis,
Mechanism, and Function. Cell.
Cevec, M., Thibaudeau, C., and Plavec, J. (2008). Soluc-
tione structure of a let-7 miRNA:lin41 mRNA com-
plex from C.elegans. Nucleic Acids Research.
Cheatham, T. E. (2004). Simulation and modelling of nu-
cleic acid structure, dynamics and interactions. Cur-
rent Opinion in Structural Biology.
Cornell, W. D., Cieplak, P., Bayly, C. I., Gould, I. R., Merz,
K. M., Ferguson, D. M., Spellmeyer, D. C., Fox, T.,
Caldwell, J. W., and Kollman, P. A. (1995). A second
generation force field for the simulation of proteins,
nucleic acids, and organic molecules. J. Am. Chem.
Soc.
Lawrenz, M., Baron, R., and McCammon, J. A.
(2009). Independent-trajectories thermodynamic-
integration free-energy changes for biomolecular sys-
tem: Determinants of N5HN1 Avian Influenza Virus
Neuraminidase Inhibition by Peramivir. Journal of
Chemical Theory and Computation.
Pan, Y. and Mackerell, A. D. (2003). Altered structural
fluctuations in duplex RNA versus DNA: a confor-
mational switch involving base pair opening. Nucleic
Acids Research.
Schwarz, D. S. and Zamore, P. D. (2002). Why do miRNAs
live in the miRNP? Genes e Development.
Van Der Spoel, D., Lindahl, E., Hess, B., Groenhof, G.,
Mark, A. E., and Berendsen, H. J. C. (2005). GRO-
MACS: Fast, flexible, and free. Journal of computa-
tional chemistry.
Vella, M. C., Reinert, K., and Slack, F. J. (2004). Ar-
chitecture of a validate microRNA::target interaction.
Chemistry e Biology.
BINDING FREE ENERGY CALCULATION VIA MOLECULAR DYNAMICS SIMULATIONS FOR A miRNA:mRNA
INTERACTION
321