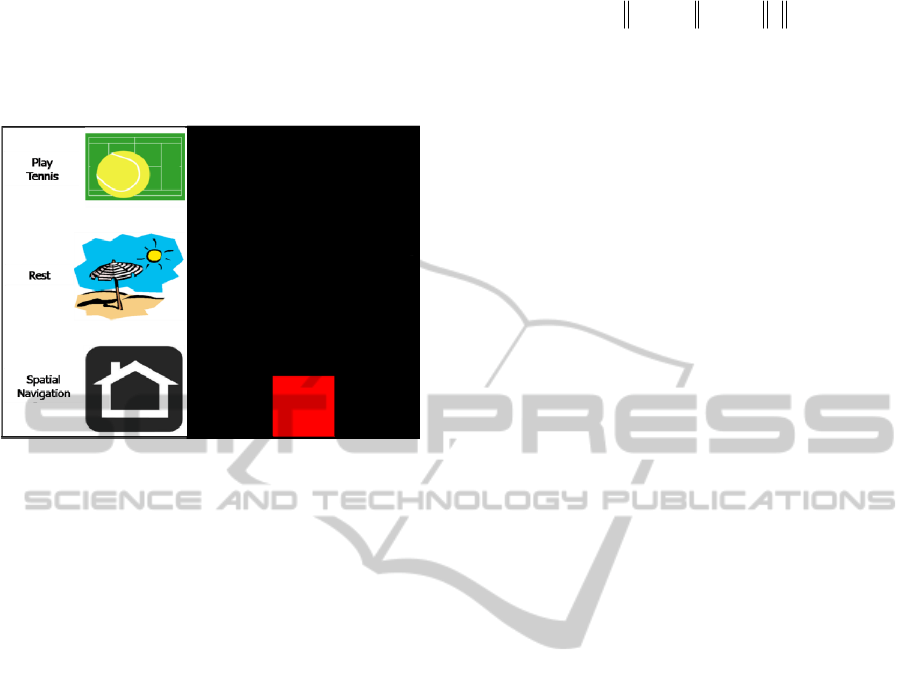
filtered (1-45 Hz) and eye movements were removed
from recordings utilizing Independent Component
Analysis (ICA). For the EEG analysis we considered
the interval [5:10] seconds in the middle of task
execution.
Figure 1: Stimulation window used for the experiment.
Subjects were asked to execute a particular task of mental
imagery according to the position of a red target on the
screen.
2.2 High Resolution EEG
High-resolution EEG technologies have been
developed to enhance the spatial information content
of EEG activity.
Accurate estimates of the cortical current density
could be obtained by using adequately detailed
geometrical reconstruction of the main
compartments lying between the cortical generator
sources and the EEG sensors. These estimates can be
obtained by solving a linear problem (Babiloni et al.,
2005), by means of a transfer matrix (lead field
matrix) that mimics the effects of the volume
conductor. In mathematical terms the relationship
between the modeled sources x, the lead field matrix
A, the EEG measurements b and the noise n can be
written as
Ax = b + n (1)
The solution of this linear system provides an
estimation of the dipole source configuration x that
generates the measured EEG potential distribution b.
The system includes also the measurement noise
n, assumed to be normally distributed. A is the lead
field or the forward transmission matrix, whose j-th
column describes the potential distribution generated
on the scalp electrodes by the j-th unitary dipole.
The current density solution vector ξ was
obtained as:
2
2
2
minarg
NM
A xbx
x
λξ
+−=
(2)
where M, N are the matrices associated to the
metrics of the data and of the source space,
respectively, λ is the regularization parameter and
|| x ||
M
represents the M norm of the vector x. The
solution of Eq. (2) is given by the inverse operator
G:
Gbξ
(3)
)
1
111
−
−−−
+
′′
= MAANANG
λ
(4)
An optimal regularization of this linear system was
obtained by the L-curve approach.
Using the relations described above, an estimate
of the signed magnitude of the dipolar moment for
each one of the 5,000 cortical dipoles was obtained
for each time point.
2.3 Spectral Cortical Activity
From the cortical waveforms, we estimated the
spectral activity, during the considered task time
interval, for each one of the 5 thousands dipoles of
the cortical model used.
T-test values obtained from comparisons
between Tennis-Rest and Navigation-Rest were then
mapped on the cortical model in different frequency
bands, defined according to Individual Alpha
Frequency (IAF) to take into account inter-
individual differences in localization of alpha band.
The IAF, defined as the individual frequency peak
within the alpha band, was determined from the Fast
Fourier Transform spectra over posterior leads
(parietal, parieto-occipital, and occipital).
Individually defined bands considered were:
Theta (IAF-6 / IAF-2), Alpha (IAF-2 / IAF+2), Beta
(IAF+2 / IAF+14) and Gamma (IAF+15 / IAF+30).
The uncorrected Student’s test and the appropriate
techniques of the False Discovery Rate (FDR) (Yoav
and Yekutieli, 2001) and the Bonferroni correction
for multiple comparisons were applied to the
evaluation of the power spectral maps estimated
from the data.
3 RESULTS
By following the procedure and the methods
illustrated above, we obtained statistical scalp and
cortical maps for each frequency band of interest
and experimental condition.
In the following figures, T-test values,
BIOSIGNALS 2011 - International Conference on Bio-inspired Systems and Signal Processing
286