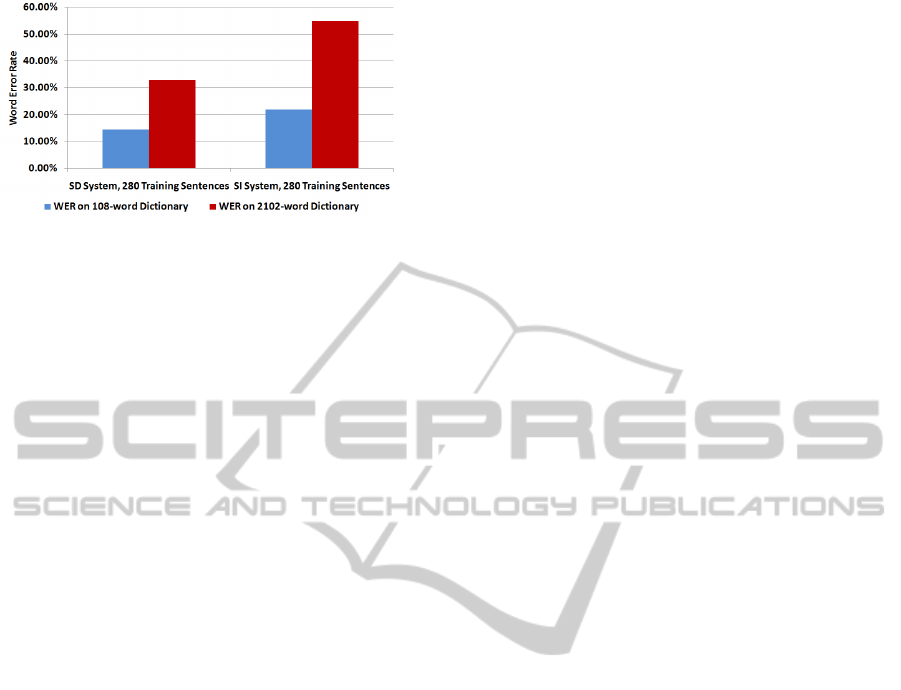
Figure 6: Average performance of systems on extended test-
ing vocabulary.
EMG recording sessions, between which the EMG
electrodes have been removed and reattached. We
demonstrated that session-independent EMG-based
speech recognition yields a suitable performance,
and that in particular, when testing is performed
on unseen sessions, the session-independent system
performs significantly better than a similarly large
session-dependent system, which shows that the
session-independent training approach indeed in-
creases the robustness of the system. We also showed
that adapting a session-independent system towards
a specific test session further improves the system
performance.
This technology allows us to create larger EMG-
based speech recognition systems than the ones pre-
viously investigated. We have shown that our current
best system can deal with vocabulary sizes ranging
up to 2.100 words, which brings EMG-based speech
recognition within a performance range which makes
spontaneous conversation possible.
Further steps in the field of EMG-based speech
processing may include a systematic study of the
discrepancies between different recording sessions,
which could not only improve the systems presented
in this paper, but also give further insight in what
causes these discrepancies. Second, transiting to true
speaker-independent systems is another major goal
for the future. In order to achieve it, however, fur-
ther studies on the behavior of the EMG signals of
the articulatory muscles are needed.
REFERENCES
Chan, A., Englehart, K., Hudgins, B., and Lovely, D.
(2001). Myoelectric Signals to Augment Speech
Recognition. Medical and Biological Engineering
and Computing, 39:500 – 506.
Denby, B., Schultz, T., Honda, K., Hueber, T., and Gilbert,
J. (2010). Silent Speech Interfaces. Speech Commu-
nication, 52.
Janke, M., Wand, M., and Schultz, T. (2010a). A Spec-
tral Mapping Method for EMG-based Recognition of
Silent Speech. In Proc. B-INTERFACE.
Janke, M., Wand, M., and Schultz, T. (2010b). Impact
of Lack of Acoustic Feedback in EMG-based Silent
Speech Recognition. In Proc. Interspeech.
Jorgensen, C., Lee, D., and Agabon, S. (2003). Sub Au-
ditory Speech Recognition Based on EMG/EPG Sig-
nals. In Proceedings of International Joint Conference
on Neural Networks (IJCNN), pages 3128 – 3133,
Portland, Oregon.
Jou, S.-C., Schultz, T., Walliczek, M., Kraft, F., and Waibel,
A. (2006). Towards Continuous Speech Recogni-
tion using Surface Electromyography. In Proc. Inter-
speech, pages 573 – 576, Pittsburgh, PA.
Jou, S.-C. S., Schultz, T., and Waibel, A. (2007). Contin-
uous Electromyographic Speech Recognition with a
Multi-Stream Decoding Architecture. In Proceedings
of the IEEE International Conference on Acoustics,
Speech, and Signal Processing (ICASSP), pages 401 –
404, Honolulu, Hawaii.
Leggetter, C. J. and Woodland, P. C. (1995). Maximum
Likelihood Linear Regression for Speaker Adaptation
of Continuous Density Hidden Markov Models. Com-
puter Speech and Language, 9:171–185.
Maier-Hein, L., Metze, F., Schultz, T., and Waibel, A.
(2005). Session Independent Non-Audible Speech
Recognition Using Surface Electromyography. In
IEEE Workshop on Automatic Speech Recognition
and Understanding, pages 331 – 336, San Juan,
Puerto Rico.
Schultz, T. and Wand, M. (2010). Modeling Coarticulation
in Large Vocabulary EMG-based Speech Recognition.
Speech Communication, 52:341 – 353.
Sch
¨
unke, M., Schulte, E., and Schumacher, U. (2006).
Prometheus - Lernatlas der Anatomie, volume [3]:
Kopf und Neuroanatomie. Thieme Verlag, Stuttgart,
New York.
Wand, M. and Schultz, T. (2009). Towards Speaker-
Adaptive Speech Recognition Based on Surface Elec-
tromyography. In Proc. Biosignals, pages 155 – 162,
Porto, Portugal.
BIOSIGNALS 2011 - International Conference on Bio-inspired Systems and Signal Processing
300