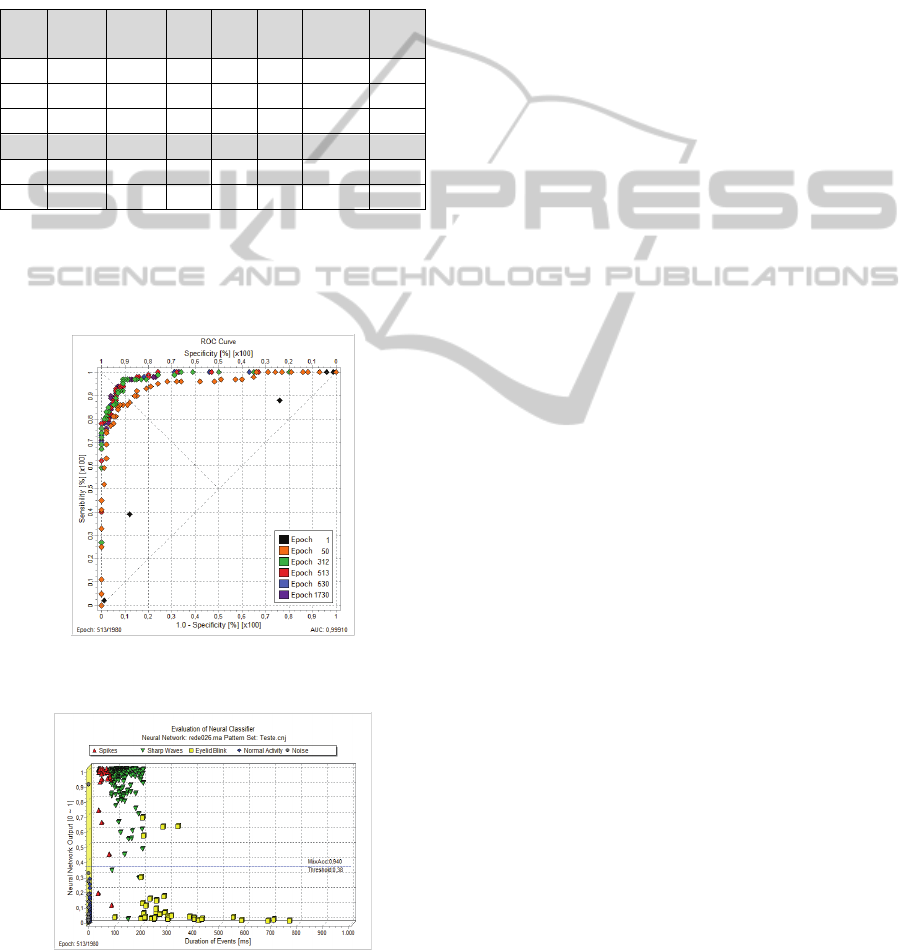
Table 2 shows the performance indexes obtained
with the neural classifier and the Figure 6 shows the
ROC curves of different epochs of training.
The epoch 513 had the highest value for
sensitivity and specificity, therefore, also showed the
highest rates of performance demonstrating that the
best results are obtained next to the epoch 530,
which was the occurrence point of early stopping.
Table 2: Obtained results between the epochs of training.
Epoch MSE
train
MSE
vald
Sens.
[%]
Spec.
[%]
Acc.
[%]
Threshold
AUC
Máx.
1 0,24992 0,24581 81,08 45,02 54,39 0,54 0,62150
50 0,08106 0,09933 91,76 82,61 88,07 0,56 0,99500
312 0,02028 0,05528 96,02 93,58 95,09 0,34 0,99790
513 0,00973 0,05180 97,14 94,55 96,14 0,38 0,99910
530 0,00918 0,05180 97,14 94,55 96,14 0,38 0,99850
1730 0,00123 0,05324 96,05 94,44 95,44 0,24 0,99630
After the evaluation of the neural classifier a
final test was performed by selecting the epoch 513,
which represents the highest AUC (AUC
Máx
=
0.99910).
Figure 6: ROC curves for some epochs of training. It can
be observed the epoch 513 had the higher AUC index.
Figure 7: Representation of the classification performed
with the test pattern set selecting the epoch 513 (Highest
AUC Index).
It was used a set of test with 285 events. Figure 7
shows the classification made by the neural
classifier, based in the epoch 513 of training
reaching values of sensitivity of 97.14%, specificity
of 94.55% and accuracy of 96.14%
5 CONCLUSIONS
In the obtained results using the Wavelet Transform
was observed that only the amplitude of decomposed
signals cannot separate Epileptiform and Non-
Epileptiform events reaching values of sensitivity of
96.43%, specificity of 88.03% and accuracy of
92.98%. Further studies are being made with the
Wavelet Multiresolution Analysis to signals with
512 Hz of sample rate.
The neural classifier evaluation was performed
using the performance indexes (AUC index and
accuracy index). These indexes could be an efficient
way to verify the performance of the classifier. The
best results of the classifier training were at the
epochs that the indexes obtained are located near to
the epoch indicated by the early stopping. The
experiments with the neural classifier using signals
without processing reached better results than
signals processed by the Wavelet Transform:
sensitivity of 97.14%, specificity of 94.55% and
accuracy of 96.14%.
It can be concluded that the high sample rate of
the EEG signals influence directly in the recognition
process. With a high sample rate more pattern details
are passed to the Neural Network inputs, improving
distinction between the events, which allowed
achieve better results without the need to pre-process
the EEG signals. However, the high sample rate
means more details about the signal, and fast
variations present in the signal that characterized
high frequency are highlighted too. This implied in
an increase of false positives due to the fact that the
Wavelet Transform confuse fast variations with
spikes. This fact explains the difference between the
rates of sensitivity and specificity among the use or
not of the Wavelet Transform as pre-processing the
inputs of neural classifier.
REFERENCES
Wilson, S. B., Emerson R., 2002. Spike Detection: a
review and comparison of algorithms. Clinical
Neurophysiology. Vol. 113, pp. 1873-1881.
Niedermeyer, E., Silva, F. L., 2004.
Electroencephalography: Basic Principles, Clinical
BIOSIGNALS 2011 - International Conference on Bio-inspired Systems and Signal Processing
508