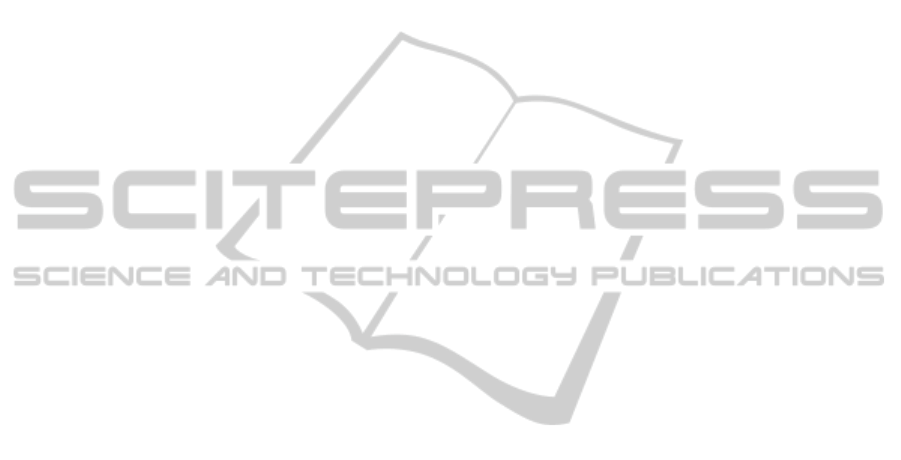
Furthermore, integrating Trusted information in the
document abstract set makes the results more accurate
also reducing the biological correlation score. BCL2
gene, for instance, is functionally specific of Apopto-
sis biological process. On the wave of this assertion,
this gene further decreases the correlation score in the
case of both Angiogenesis and Signal Transduction
when applying Trusted information. The expected be-
havior would be at most of unaltered result because
the Trusted information do not addict any further in-
formation concerning the correlation score between
BCL2 and the two biological processes. However,
integrating Trusted information increases the corre-
lation score of those terms related to the biological
processes and their correlation scores overcomes the
BCL2 one, that in turn becomes lower. Similar con-
sideration are also valid about the biological correla-
tion score between BCL-xL gene and ANGPT2 gene
and Signal Transduction biological process.
5 CONCLUSIONS
The proposed semantic analysis tool provides a
framework for measuring the biological correlation
score among biomedical terms. The score is the
results of a text mining analysis performed on the
abstracts of the most relevant scientific publications
with the support of the completeness of the UMLS
Metathesaurus. The knowledgeextracted by biomedi-
cal literature is further integrated with the information
coming from sources generally known as trustworthy.
In order to satisfy this requirement, public biological
pathways databases have been chosen and the embed-
ded information are first retrieved and then correlated
in the overall semantic analysis flow. Moreover, in
order to integrate the knowledge coming from an het-
erogeneous base of information, a new version of the
latent semantic analysis, based on the sprinkling tech-
nique, has been developed. The results remark the
efficiency of the proposed approach in enhancing the
accuracy of the biological correlation score computa-
tion by combining the knowledge extracted by scien-
tific document abstract set with the pathways infor-
mation.
As future step the complete automation of the tool
will allow to retrieve the document abstract set in an
automated and accurate manner. Moreover, the in-
tegration of information coming from other trusted
sources will be tailored and developed in order to bet-
ter improve the overall accuracy and robustness of the
proposed semantic analysis method.
REFERENCES
Abate, F., Ficarra, E., Acquaviva, A., and Macii, E. (2010).
An automated tool for scoring biomedical terms cor-
relation based on semantic analysis. In International
Conference on Complex, Intelligent and Software In-
tensive Systems.
Aronson, A. R. (2001). Effective mapping of biomedical
text to the umls. metathesaurus: The metamap pro-
gram. In AMIA Fall Symposium.
BioPAX (2007). Biological pathways exchange.
http://www.biopax.org.
Bodenreider, O. (2004). The unified medical language sys-
tem (umls): integrating biomedical terminology. Nu-
cleic Acids Research.
Cerami, E. G., Bader, G. D., Gross, B. E., and Sander, C.
(2006). cpath: open source software for collecting,
storing, and querying biological pathways. In Bioin-
formatics.
Chakraborti, S., Mukras, R., Lothian, R., Wiratunga, N.,
Watt, S., and Harper, D. (2006). Sprinkling: Super-
vised latent semantic indexing. Advances in Informa-
tion Retrieval.
Doms, A. and Schroeder, M. (2005). Gopubmed: explor-
ing pubmed with the gene ontology. Nucleic Acids
Research.
Gliozzo, A. M. and Strapparava, C. (2005.). Domain ker-
nels for text categorization. In Ninth Conference on
Computational Natural Language Learning.
Hermjakob, H. et al. (2004). The hupo psi’s molecular inter-
action format–a commu- nity standard for the repre-
sentation of protein interaction data. Natural Biotech-
nology.
Hill, D. P., Smith, B., McAndrews-Hill, M. S., and Blake,
J. A. (2008). Gene ontology annotations: what they
mean and where they come from. In Bioinformatics.
Kanehisa, M. and Goto, S. (1999). Kegg: Kyoto encyclo-
pedia of genes and genomes. Nucleic Acids Research.
MeSH (2005). Medical subject headings (mesh) fact sheet.
National Library of Medicine.
Pathway Commons (2007). Pathway commons.
http://www.pathwaycommons.org.
Plake, C., Royer, L., Winnenburg, R., Hakenberg, J., and
Schroeder, M. (2009). Gogene: gene annotation in
the fast lane. Nucleic Acids Research.
Romero, P., Wagg, J., Green, M. L., Kaiser, D., Krummen-
acker, M., and Karp, P. D. (2004). Computational pre-
diction of human metabolic pathways from the com-
plete human genome. Genome Biology.
Stark, C., Breitkreutz, B. J., Reguly, T., Boucher, L., Bre-
itkreutz, A., and Tyers, M. (2006). Biogrid: a general
repository for interaction datasets. Nucleic Acids Re-
search.
The Gene Ontology Consortium (2000). Gene ontology:
tool for the unification of biology. Nature Genetics.
Wang, J. Z., Du, Z., Payattakool, R., Yu, P. S., and Chen,
C. F. (2007). A new method to measure the semantic
similarity of go terms. In Bioinformatics.
BIOINFORMATICS 2011 - International Conference on Bioinformatics Models, Methods and Algorithms
74