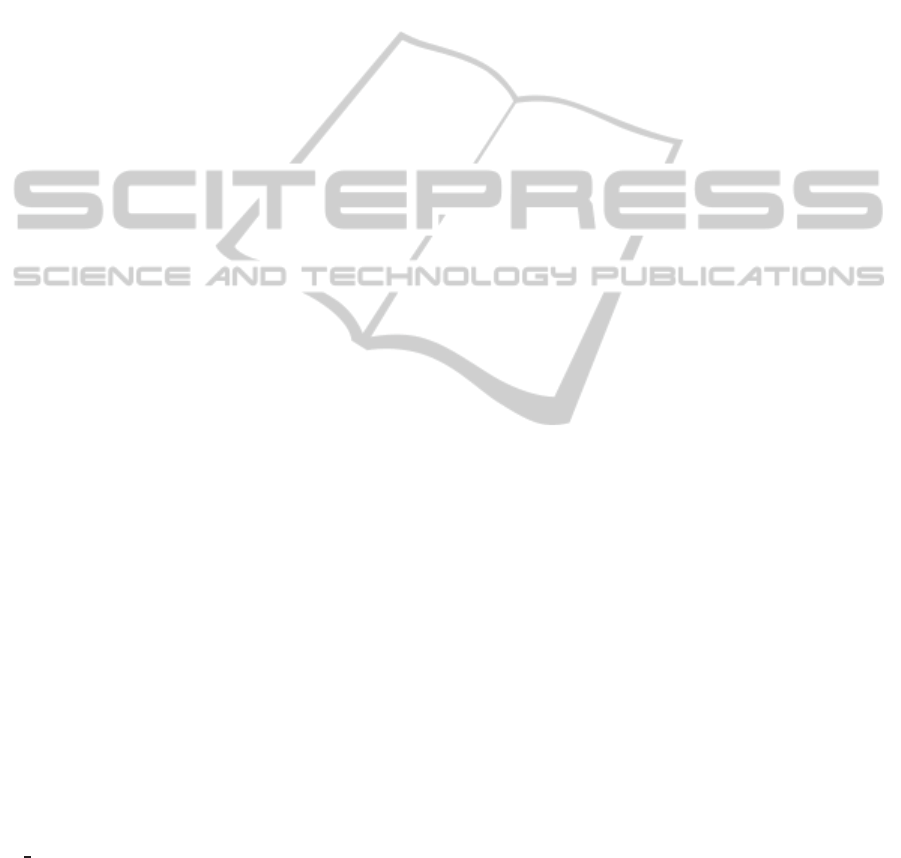
(where) pathway for keypoints. This idea is strength-
ened by the fact that area MT also plays a role in
the motion-aftereffect illusion (Kohn and Movshon,
2003), which is tightly related to motion adaptation
and prediction. Therefore, motion prediction might
play a very important role in the dorsal pathway, not
only where objects are now but also where they are
expected next. Such predictions tied to objects may
lead to much more efficient processing, for exam-
ple in robot vision, because most image regions can
be skipped. Nevertheless, robot vision also requires
some sort of “arousal” system for spotting new or un-
expected moving objects.
Having a model for matching keypoints in con-
secutive time frames for optical flow, the same prin-
ciple can be applied to stereo (disparity), matching
left and right frames. Since information of one of the
two frames is already available for optical flow, the re-
quired additional CPU time will be limited, especially
if only the distance of moving objects is necessary, for
example to detect objects which may be on collision
course, with and without egomotion. In general, how-
ever, disparity can be used for obtaining a 3D sketch
of an entire scene, plus the 3D structure of individual
objects in the scene which may complement the (2D)
line/edge scale space for object recognition. More-
over, optical flow and disparity can be combined to
obtain more robust object segregations.
Keypoints can complement the line/edge coding
in attributing depth, not only to vertical lines and
edges but also line and edge junctions. This results
in a sort of 3D “wireframe” representation as used in
modelling solid objects in computer graphics. The
fact that projections from left and right eyes are very
close in the cortical hypercolumns and that many sim-
ple and complex cells are also disparity tuned sug-
gests that our visual system processes 3D objects in
the same way, probably simplifying 3D object recog-
nition.
ACKNOWLEDGEMENTS
Portuguese Foundation for Science and Technol-
ogy (FCT) through the pluri-annual funding of
the Inst. for Systems and Robotics (ISR/IST), the
POS Conhecimento Program with FEDER funds, and
FCT project SmartVision (PTDC/EIA/73633/2006).
REFERENCES
Bar, M. (2004). Visual objects in context. Nature Rev.:
Neuroscience, 5:619–629.
du Buf, J. (1993). Responses of simple cells: events, inter-
ferences, and ambiguities. Biol. Cybern., 68:321–333.
Duffy, C. and Wurtz, R. (1991). Sensitivity of mst neu-
rons to optic flow stimuli. I. A continuum of response
selectivity to large-field stimuli. J. Neurophysiol.,
65(6):1329–1345.
Hubel, D. (1995). Eye, Brain and Vision. Scientific Ameri-
can Library.
Kohn, A. and Movshon, J. (2003). Neuronal adaptation to
visual motion in area mt of the macaque. Neuron,
39:681–691.
Morrone, M., Tosetti, M., Montanaro, D., Fiorentini, A.,
Cioni, G., and Burr, D. (2000). A cortical area that
responds specifically to optic flow, revealed by fMRI.
Nature Neuroscience, 3(7):1322 – 1328.
Oliva, A. and Torralba, A. (2006). Building the gist of a
scene: the role of global image features in recognition.
Progress in Brain Res.: Visual Perception, 155:23–26.
Orban, G., Lagae, L., Verri, A., Raiguel, S., Xiao, D., Maes,
H., and Torre, V. (1992). First-order analysis of optical
flow in monkey brain. PNAS, 89(7):2595–2599.
Rodrigues, J. and du Buf, J. (2006). Multi-scale keypoints
in V1 and beyond: object segregation, scale selection,
saliency maps and face detection. BioSystems, 2:75–
90.
Rodrigues, J. and du Buf, J. (2009a). A cortical frame-
work for invariant object categorization and recogni-
tion. Cognitive Processing, 10(3):243–261.
Rodrigues, J. and du Buf, J. (2009b). Multi-scale lines and
edges in v1 and beyond: brightness, object categoriza-
tion and recognition, and consciousness. BioSystems,
95:206–226.
Smith, A., Wall, M., Williams, A., and Singh, K. (2006).
Sensitivity to optic flow in human cortical areas
mt and mst. European Journal of Neuroscience,
23(2):561–569.
Wall, M. and Smith, A. (2008). The representation of ego-
motion in the human brain. Current Biology, 18:191–
194.
Warren, P. and Rushton, S. (2009). Optic flow process-
ing for the assessment of object movement during ego
movement. Current Biology, 19(19):1555–1560.
William, K. and Charles, J. (2008). Cortical neuronal re-
sponses to optic flow are shaped by visual strategies
for steering. Cerebral Cortex, 18(4):727–739.
Wurtz, R. (1998). Optic flow: A brain region devoted to
optic flow analysis? Current Biology, 8(16):R554–
R556.
OPTICAL FLOW BY MULTI-SCALE ANNOTATED KEYPOINTS - A Biological Approach
315