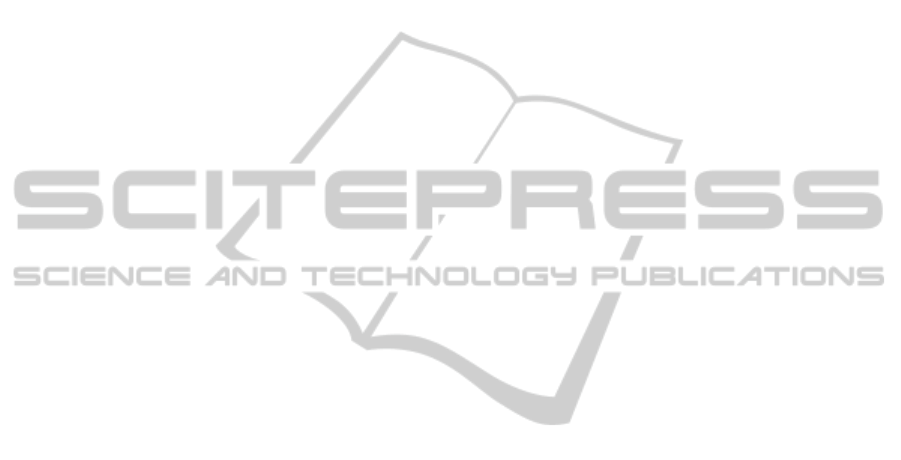
4.6 Summarry
Rule classification trees enable the user to reduce in-
terdependent, superfluous and strongly outlying data.
Moreover, rule trees obtained by us have the ”white-
box” character, i.e., the knowledge representation is
overt, readable and useful for diagnosticians and clin-
icians. Rule knowledge-based systems are small and
do not exceed fifteen classification (decision) rules.
The greatest number (twelve) of rules was generated
for the Eichmann’s system. All our analyses demon-
strate that in an index-rule approach the selected in-
dexes, Eichman’s (calculated in [T]), extended Di-
amond’s, Leary’s (without two basic personality di-
mensions) are used not only for supporting diagnosis
in the so-called interpretation quiet (mostly the range
40 - 60 [T]) or only for delivering additional inter-
pretation (descriptive) hypotheses. Indexes selected
by us and additionally transformed into the index-rule
form give the high accuracy (above 80%). Therefore,
they can not be overlooked. This is their new clini-
cal and psychometric application. The systems men-
tioned earlier are implemented in Copernicus and they
support quantitative and clinical differential diagnosis
based on an index-rule approach.
5 CONCLUSIONS AND FURTHER
WORK
In the paper we have shown an index-rule-based ap-
proach to differential diagnosis of patients with men-
tal disorders. In our experiments we have used the
Copernicus system and the WEKA system. The
Copernicus system is a tool created by our team.
Among others, it enables the user to calculate a wide
variety of indexes used in diagnosis of psychopatholo-
gies. Some of them can also be visualized by means
of appropriate diagrams. WEKA is a collection of
machine learning algorithms for data mining tasks.
Our experiments deliver important information for
diagnosticians and clinicians about individual scales
(their validities and utilities) used in the MMPI test-
ing of patients. In the future work, among others, we
are going to incorporate rule generation algorithms
and visualization modules into the Copernicus sys-
tem. Our main goal is to deliver to diagnosticians and
clinicians an integrated tool supporting the compre-
hensive diagnosis of patients. The Copernicus system
is flexible and it is dedicated for supporting differen-
tial diagnosis of profiles of patients examined using
multiphasic personality inventories.
REFERENCES
Choynowski, M. (1964). Multiphasic Personality Inventory
(in Polish). Psychometry Laboratory, Polish Academy
of Sciences, Warsaw.
Cios, K., Pedrycz, W., Swiniarski, R., and Kurgan, L.
(2007). Data Mining. A Knowledge Discovery Ap-
proach. Springer.
Dahlstrom, W., Welsh, G., and Dahlstrom, L. (1986). An
MMPI Handbook, vol. 1-2. University of Minnesota
Press, Minneapolis.
Duch, W., Kucharski, T., Gomuła, J., and Adamczak, R.
(1999). Machine learning methods in analysis of psy-
chometric data. Application to Multiphasic Personal-
ity Inventory MMPI-WISKAD (in Polish). Toru
´
n.
Gan, G., Ma, C., and Wu, J. (2007). Data Clustering. The-
ory, Algorithms, and Applications. SIAM, Philadel-
phia, ASA Alexandria, VA.
Gomuła, J., Paja, W., Pancerz, K., and Szkoła (2010a).
A preliminary attempt to rules generation for mental
disorders. In Pardela, T. and Wilamowski, B., edi-
tors, Proc. of the HSI’2010, pages 763–765, Rzesz
´
ow,
Poland.
Gomuła, J., Pancerz, K., and Szkoła, J. (2010b). Analysis
of MMPI profiles of patients with mental disorders -
the first unveil af a new computer tool. In Grzech,
A.,
´
Swia¸tek, P., and Brzostowski, K., editors, Appli-
cations of Systems Science, pages 297–306. Academic
Publishing House EXIT, Warsaw, Poland.
Gomuła, J., Pancerz, K., and Szkoła, J. (2010c). Classifi-
cation of MMPI profiles of patients with mental disor-
ders - experiments with attribute reduction and exten-
sion. In Yu, J. et al., editors, Rough Sets and Knowl-
edge Technology, volume 6401 of LNAI, pages 411–
418. Springer-Verlag, Berlin Heidelberg.
Kucharski, T. and Gomuła, J. (1998a). Introduction to the
MMPI-2 questionnaire (in Polish). Toru
´
n.
Kucharski, T. and Gomuła, J. (1998b). Introduction to the
MMPI-WISKAD questionnaire (in Polish). Toru
´
n.
Lachar, D. (1974). The MMPI: Clinical assessment and au-
tomated interpretations. Western Psychological Ser-
vices, Fate Angeles.
Leary, T. (1957). Interpersonal diagnosis of personality:
a functional theory and methodology for personality
evaluation. The Ronald Press Company, New York.
Pancheri, P., Biondi, M., Portuesi, G., and P.L., M. (1992).
Psicodiagnostica ed evoluzione nosografica: la ver-
sione dsm iii del sistema mmpi-apap. Rivista di
Psichiatria, 1:7–22.
Płu
˙
zek, Z. (1971). Value of the WISKAD-MMPI test for
nosological differential diagnosis (in Polish). The
Catholic University of Lublin.
Quinlan, J. (1993). C4.5. Programs for machine learning.
Morgan Kaufmann Publishers.
Witten, I. H. and Frank, E. (2005). Data Mining: Practi-
cal Machine Learning Tools and Techniques. Morgan
Kaufmann.
HEALTHINF 2011 - International Conference on Health Informatics
328