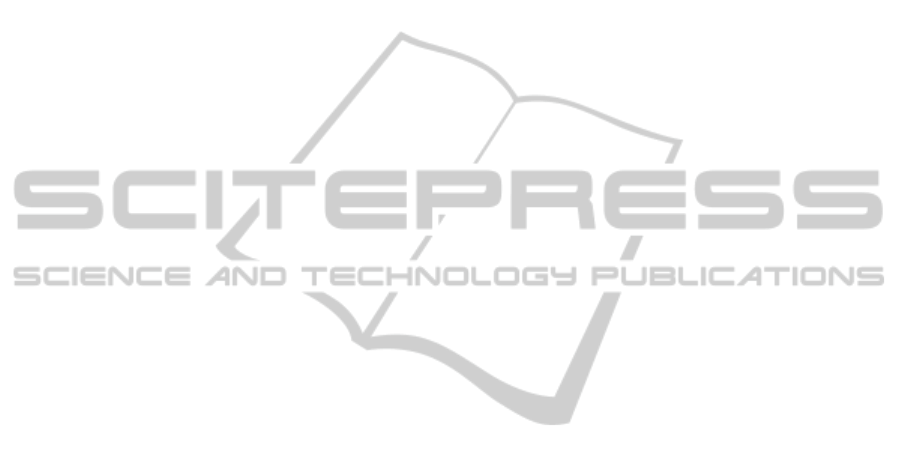
garding the precision and recall values.
4 CONCLUSIONS AND FUTURE
WORK
In this work, we were provided with 348 cases of
patients that went through mammography screening.
The objective of this work was twofold: i) find non
trivial relations among attributes by applying machine
learning techniques to these data, and; ii) learn mod-
els that could help medical doctors to quickly assess
mammograms. We used the WEKA machine learn-
ing tool and whenever applicable performed statisti-
cal tests of significance on the results.
The conclusions are threefold: (1) automatic clas-
sification of a mammography can reach equal or bet-
ter results than the ones annotated by specialists; (2)
mass density seems to be a good indicator of ma-
lignancy, as previous studies suggested; (3) machine
learning classifiers can predict mass density with a
quality as good as the specialist blind to biopsy.
As future work, we plan to extend this work
to larger data sets, and apply other machine learn-
ing techniques based on statistical relational learning,
since classifiers that fall in this category provide a
good explanation of the predicted outcomes as well
as can consider the relationship among mammograms
of the same patient. We would also like to investi-
gate how other attributes can affect malignancy or are
related to the other attributes.
ACKNOWLEDGEMENTS
This work has been partially supported by the
projects HORUS (PTDC/EIA-EIA/100897/2008)
and Digiscope (PTDC/EIA-CCO/100844/2008)
and by the Fundac¸˜ao para a Ciˆencia e Tecnologia
(FCT/Portugal). Pedro Ferreira has been supported
by an FCT BIC scholarship.
REFERENCES
Abbass, H. A. (2002). An evolutionary artificial neural net-
works approach for breast cancer diagnosis. Artificial
Intelligence in Medicine, 25:265.
Ayer, T., Alagoz, O., Chhatwal, J., Shavlik, J. W., Kahn, C.
E. J., and Burnside, E. S. (2010). Breast cancer risk es-
timation with artificial neural networks revisited: dis-
crimination and calibration. Cancer, 116(14):3310–
3321.
Cory, R. C. and Linden, S. S. (1993). The mammographic
density of breast cancer. AJR Am J Roentgenol,
160:418–419.
Davis, J., Burnside, E. S., Dutra, I. C., Page, D., and Costa,
V. S. (2005). Knowledge discovery from structured
mammography reports using inductive logic program-
ming. In American Medical Informatics Association
2005 Annual Symposium, pages 86–100.
Hall, M., Frank, E., Holmes, G., Pfahringer, B., Reutemann,
P., and Witten, I. H. (2009). The weka data mining
software: An update. SIGKDD Explorations, 11:263–
286.
Jackson, V. P., Dines, K. A., Bassett, L. W., Gold, R. H., and
Reynolds, H. E. (1991). Diagnostic importance of the
radiographic density of noncalcified breast masses:
analysis of 91 lesions. AJR Am J Roentgenol, 157:25–
28.
John, G. H. and Langley, P. (1995). Estimating continuous
distributions in bayesian classifiers. In Proceedings of
the Eleventh Conference on Uncertainty in Artificial
Intelligence, pages 338–345. Morgan Kaufmann, San
Mateo.
Nassif, H., Page, D., Ayvaci, M., Shavlik, J., and Burn-
side, E. S. (2010). Uncovering age-specific invasive
and dcis breast cancer rules using inductive logic pro-
gramming. In Proceedings of 2010 ACM International
Health Informatics Symposium (IHI 2010). ACM Dig-
ital Library.
Nassif, H., Woods, R., Burnside, E., Ayvaci, M., Shavlik,
J., and Page, D. (2009). Information extraction for
clinical data mining: A mammography case study. In
ICDMW ’09: Proceedings of the 2009 IEEE Interna-
tional Conference on Data Mining Workshops, pages
37–42, Washington, DC, USA. IEEE Computer Soci-
ety.
Platt, J. C. (1998). Sequential minimal optimization: A fast
algorithm for training support vector machines. Tech-
nical Report MSR-TR-98-14, Microsoft Research.
Sickles, E. A. (1991). Periodic mammographic follow-up of
probably benign lesions: results in 3,184 consecutive
cases. Radiology, 179:463–468.
Street, W. N., Mangasarian, O. L., and Wolberg, W. H.
(1995). An inductive learning approach to prognos-
tic prediction. In ICML, page 522.
Wolberg, W. H. and Mangasarian, O. L. (1990). Multisur-
face method of pattern separation for medical diagno-
sis applied to breast cytology. In Proceedings of the
National Academy of Sciences, 87, pages 9193–9196.
Woods, R. and Burnside, E. (2010). The mammographic
density of a mass is a significant predictor of breast
cancer. Radiology. to appear.
Woods, R., Oliphant, L., Shinki, K., Page, D., Shavlik, J.,
and Burnside, E. (2009). Validation of results from
knowledge discovery: Mass density as a predictor of
breast cancer. J Digit Imaging, pages 418–419.
Wu, Y., Giger, M. L., Doi, K., Vyborny, C. J., Schmidt,
R. A., and Metz, C. E. (1993). Artificial neural net-
works in mammography: application to decision mak-
ing in the diagnosis of breast cancer. Radiology,
187:81–87.
HEALTHINF 2011 - International Conference on Health Informatics
342