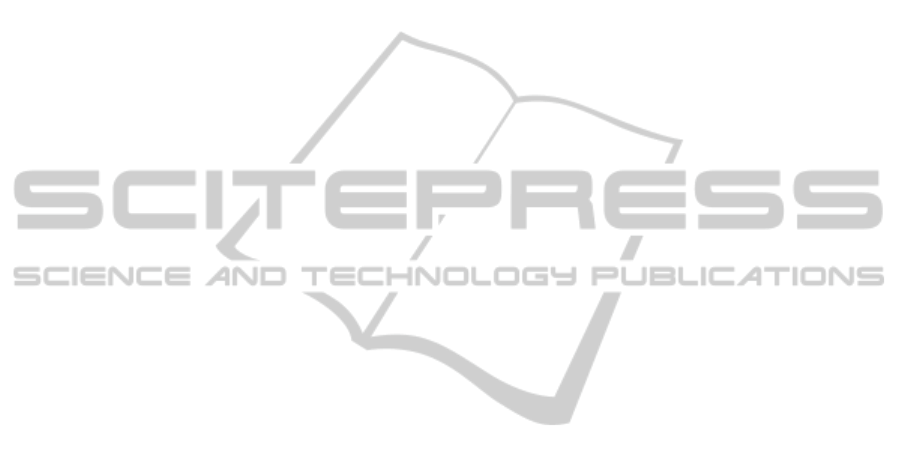
effect is a bit more challenging. Two approaches
were implemented:
Likelihood ratio test as discussed in
(Verbeke and Molenberghs, 2000, p. 65): the
test statistic is a difference in log-likelihood
between models with and without random
effect multiplied by two. Under the null
hypothesis (
=0) it is asymptotically
distributed as a mixture (weights ½ and ½)
of chi-squared distribution with 1 degree of
freedom and constant 0.
Permutation test: 1000 permutations of
observations within each experimental group
are performed to see how much
exceptionally high is the test statistic (the
observed residual variability explained by
LE). P-value is a fraction of randomly
generated test statistics greater than actually
observed test statistic.
All calculations were done in R 2.9.2, nlme
package was used for LE inference in mixed models.
4.3 Simulation Study
In the first scenario a random sample of 100 000
cases was generated for each considered value of
/(
+
) (from 0.00 to 0.75 by 0.05). For each
case two random vectors were generated such that
observation i of litter l(i) was computed as follows
=
()
+
,
(3)
where
()
and
were sampled from distributions
N(0,
) and N(0,
), respectively.
For each case T-test was performed and resulting
p-value recorded. The percentage of cases with p-
value below 5% was reported as Type-I-error.
In the second scenario for the same set of
considered values of
/(
+
) and a temporary
suggestion for possible minimal sample size n we
generated 10 000 samples of two vectors of length n
such that observation i of litter l(i) was computed as
follows
=
+
+ 2
,
(4)
where equals zero for the first vector and one for
the second vector;
()
and
were sampled from
distributions N(0,
) and N(0,
), respectively.
For each case we compared means of vectors by
ANOVA with a random litter effect and record the
p-value. If the percentage of cases with p-value
below 5% was lower than 80% then n was increased
by 1 and the whole procedure was repeated.
ACKNOWLEDGEMENTS
This work was supported in part by the Praemium
Academiae, Academy of Sciences of the Czech
Republic, and by grants IM6837805002 and
AV0Z50520514 from the Ministry of Education,
Youth and Sports of the Czech Republic.
REFERENCES
Broman, K. W., Sen, S., 2009. A Guide to QTL Mapping
with R/qtl. Berlin. Springer.
Gentleman, R., Carey, V., Huber, W., Irizarry, R., Dudoit,
S., 2005. Bioinformatics and Computational Biology
Solutions Using R and Bioconductor. Berlin. Springer.
Glass, G. V., Peckham, P. D., Sanders, J. R., 1972.
Consequences of Failure to Meet Assumptions
Underlying the Fixed Effects Analyses of Variance
and Covariance. Review of Educational Research, 42
(3) pp. 237–288.
Gregorová, S., Divina, P., Storchova, R., Trachtulec, Z.,
Fotopulosova, V., Svenson, K. L., Donahue, L. R.,
Paigen, B., Forejt, J., 2008. Mouse consomic strains:
exploiting genetic divergence between Mus m.
musculus and Mus m. domesticus subspecies. Genome
Research, 18 (3) pp. 509–515.
Grubb, S. C., Maddatu, T. P., et al., 2009. Mouse phenome
database. Nucleic Acids Res, 35 pp. D643–D649.
Haseman, J. K., Kupper L. L., 1979. Analysis of
dichotomous response data from certain toxicological
experiments. Biometrics, 35 (1) pp. 281–293.
Nadeau, J., Singer, J., Matin, A., Lander, E., 2000.
Analysing complex genetic traits with chromosome
substitution strains. Nat. Genet., 24 pp. 221–225.
Silver, L. M., 1995. Mouse Genetics: Concepts and
Applications. Oxford. Oxford University Press.
Tordoff, M. G., Bachmanov, A. A., Reed, D. R., 2000.
Forty mouse strain survey of voluntary calcium intake,
blood calcium, and bone mineral content. Physiol
Behav., 91 (5) pp. 632–643.
Verbeke, G., Molenberghs, G., 2000. Linear Mixed
Models for Longitudinal Data. Berlin. Springer.
LITTER EFFECT IN MOUSE PHENOTYPIC STUDIES
241