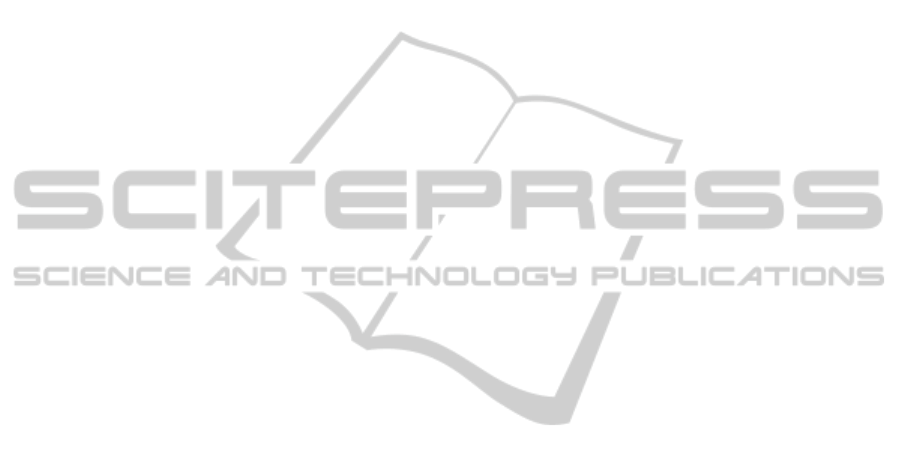
parasympathetic nervous activity in humans. Clin Sci
(Lond), 91(2):201–8.
Karlen, W., Mattiussi, C., and Floreano, D. (2009). Sleep
and wake classification with ecg and respiratory effort
signals. IEEE Transactions on Biomedical Circuits
and Systems, 3(2):71–78.
Keklund, G. and Akerstedt, T. (1997). Objective com-
ponents of individual differences in subjective sleep
quality. J Sleep Res., 6(4):217–220.
Khasawneh, A., Alvarez, S. A., Ruiz, C., Misra, S., and
Moonis, M. (2010). Discovery of sleep composition
types using expectation-maximization. In Proc. 23rd
IEEE International Symposium on Computer-Based
Medical Systems (CBMS 2010), Perth, Australia.
Kryger, M., Roth, T., and Dement, W. (2005). Principles
and Practice of Sleep Medicine. Elsevier Saunders,
Philadelphia, PA, USA, 4th edition.
Lewicke, A., Sazonov, E., Corwin, M., and Schuckers,
S. (2005). Reliable determination of sleep versus
wake from heart rate variability using neural net-
works. In Neural Networks, 2005. IJCNN ’05. Pro-
ceedings. 2005 IEEE International Joint Conference
on, volume 4.
Limoges, E., Mottron, L., Bolduc, C., Berthiaume, C., and
Godbout, R. (2005). Atypical sleep architecture and
the autism phenotype. Brain, 128(5):1049–1061.
Loomis, A., Harvey, E., and Hobart, G. (1937). Cerebral
states during sleep, as studied by human brain poten-
tials. Journal of Experimental Psychology, 21(2):127–
144.
M Malik et al (1996). Heart rate variability: standards of
measurement, physiological interpretation and clini-
cal use. task force of the european society of cardi-
ology and the north american society of pacing and
electrophysiology. Circulation, 93(5):1043–1065.
Manning, C. D., Raghavan, P., and Schutze, H. (2008).
Introduction to Information Retrieval. Cambridge
University Press. Web publication at informationre-
trieval.org.
Mietus, J., Peng, C.-K., I, I. H., Goldsmith, R., and Gold-
berger, A. (2002). The pNNx files: re-examining a
widely used heart rate variability measure. Heart,
88(4):378–380.
Moody, G. (1993). Spectral analysis of heart rate without
resampling. Computers in Cardiology, 20:715–718.
Moser, D., Anderer, P., Gruber, G., Parapatics, S., Loretz,
E., Boeck, M., Kloesch, G., Heller, E., Schmidt,
A., Danker-Hopfe, H., Saletu, B., Zeitlhofer, J., and
Dorffner, G. (2009). Sleep classification according to
AASM and Rechtschaffen & Kales: Effects on sleep
scoring parameters. Sleep, 32(2):139–149.
Neal, R. and Hinton, G. E. (1998). A view of the EM algo-
rithm that justifies incremental, sparse, and other vari-
ants. In Learning in Graphical Models, pages 355–
368. Kluwer Academic Publishers.
Propper, R., Christman, S., and Olejarz, S. (2007). Home-
recorded sleep architecture as a function of hand-
edness II: Consistent right- versus consistent left-
handers. J Nerv Ment Dis., 195(8):689–692.
Rao, M., Blackwell, T., Redline, S., Stefanick, M., Ancoli-
Israel, S., and Stone, K. (2009). Association between
sleep architecture and measures of body composition.
Sleep, 32(4):483–90.
Rechtschaffen, A. and Kales, A., editors (1968). A Manual
of Standardized Terminology, Techniques, and Scor-
ing System for Sleep Stages of Human Subjects. US
Department of Health, Education, and Welfare Public
Health Service – NIH/NIND.
Silber, M., Anconi-Israel, S., Bonnet, M., Chokroverty, S.,
Grigg-Damberger, M., Hirshkowitz, M., Kapen, S.,
Keenan, S., Kryger, M., Penzel, T., Pressman, M., and
Iber, C. (2007). The visual scoring of sleep in adults.
Journal of Clinical Sleep Medicine, 3(2):121–131.
Smith, S., Dingwall, K., Jorgensen, G., and Douglas, J.
(2006). Associations between the use of common
medications and sleep architecture in patients with un-
treated obstructive sleep apnea. Journal of Clinical
Sleep Medicine, 2(2):156–162.
Strehl, A. (2002). Relationship-based Clustering and Clus-
ter Ensembles for High-dimensional Data Mining.
PhD thesis, The University of Texas at Austin.
Sulekha, S., Thennarasu, K., Vedamurthachar, A., Raju,
T., and Kutty, B. (2006). Evaluation of sleep archi-
tecture in practitioners of Sudarshan Kriya yoga and
Vipassana meditation. Sleep and Biological Rhythms,
4(3):207–214.
Vanoli, E., Adamson, P. B., Ba-Lin, Pinna, G. D., Lazzara,
R., and Orr, W. C. (1995). Heart rate variability during
specific sleep stages: A comparison of healthy sub-
jects with patients after myocardial infarction. Circu-
lation, 91(7):1918–1922.
Zhang, L., Samet, J., Caffo, B., and Punjabi, N. (2006).
Cigarette smoking and nocturnal sleep architecture.
Am J Epidemiol., 164(6):529–537.
HEALTHINF 2011 - International Conference on Health Informatics
106