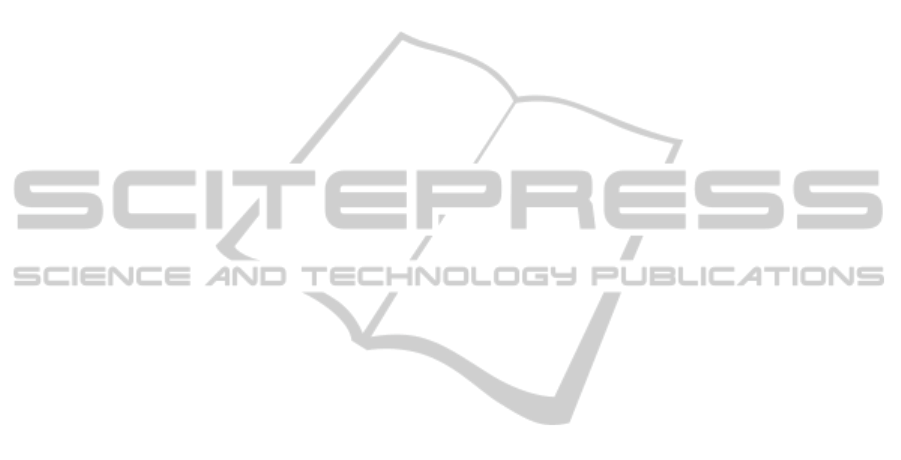
embedded in patterns of use, potentially able to
foster the growth of new active zones in artifact
space). The agents can use artifacts created by other
agents: this fact allows the occurrence of so-called
exaptation processes (Gould and Verba, 1982), very
often observed in socio-technological systems
(Villani et al. 2007) (Villani et al. 2009) (Villani et
al. 2010).
The reciprocal feedbacks between microstructure
(agents and artifacts) and macrostructure
(organizations) has long been explicitly recognized
as of fundamental importance for social sciences
(Hayek, 1948) (Olsen, 1975) (Schelling, 1978)
(Smith, 1937), but they are relatively new topics in
artificial agents research area. For long time
scientists have lacked the tools to quantitatively
model these feedbacks, nor could they deal with
their complexity. The most salient characteristic of
traditional quantitative models on these topics,
derived from economic or physical researches, is
their top-down construction: frameworks such as
fixed decision rules, common knowledge, mean field
and equilibrium assumptions occupied the greatest
part of the researches. Face-to-face interactions
among heterogeneous economic agents typically
play no role, with the only exception of the highly
stylized game tournaments (Fudenberg and Tirole,
1991) (Dutta 1999).
A major advance was the introduction of agent-
based models (Lane 1993a) (Lane 1993b) (Epstein
and Axtell, 1996) (Gilbert and Terna, 2000)
(C.Cioffi-Revilla 2002) (Ormerod et al. 2002)
(Axelrod and Tesfatsion, 2006). These models deal
with the topic of coordination and cooperation
among heterogeneous agents, often lacking a
complete knowledge of the whole system; the
models aim to bridge the gap between micro-level
interactions and emerging patterns at the macro-
level, avoiding the misleading “representative agent”
micro-macro link.
Agent-based models are very useful tools, but
many of them in the innovation context
underestimate the specific role of agents and, even
more, the attributions that agents make about artifact
identity, as noted above (Lane and Maxfield 1997)
(Lane et al. 2005). Agents and artifacts interact in
complex ways, giving rise to the so called socio-
technological systems.
In fact, one of the most intriguing observations
on these kinds of system is the growth of the
quantity and diversity of artifacts that agents use:
over time, not only the quantity and the diversity of
artifacts has grown, but also the number and kinds of
organizations has increased. These two phenomena
are in reciprocal relationship (van der Leeuw et al.
2009); both phenomena contribute in important
ways to the system’s information coordination and
processing capabilities. In particular, in the actual
world, a high rate of innovation seems to be a
peculiar and fundamental key to sustain the systems
itself.
But can the current explosion in number of
artifacts and organizational forms continue
indefinitely? How can agents lacking a global vision
of the whole system coordinate their actions, in
order to cooperate in building a coherent system?
Are there agents’ strategies that favor a sustainable
growth, and others that lead to system collapse? In
order to address this question, we need to understand
the dynamics of innovation processes.
In this paper we make use of an agent-based
model, where the relationships among agents are
mediated by the presence of artifacts. Agents
endowed with a suitable internal structure survey the
opportunities offered by their social and material
environment to create new (kinds of) artifacts, which
in turn change and shape the present pattern of
interactions heavily influencing the emergence of
new agents-artifacts (sub)systems. This kind of
approach has already provided some interesting
results highlighting the importance of relationships
among agents, which can influence the information
flows through the system (Lane et al. 2005) (Serra et
al. 2009) (Villani et al. 2007) (Villani et al. 2008). In
this paper, we describe four scenarios, which taken
together indicate that the conditions enabling a long
lasting sustainable growth are neither simple nor
widespread. The paper is organized as follows. The
second section provides a detailed introduction to
the basic innovation model. The third section
describes results obtained by exploring four different
innovation theoretic scenarios; the fourth section
presents some conclusions derived from simulations
based upon these scenarios.
2 THE MODEL
2.1 Agents and Artifacts
There are numerous approaches to studying
innovation dynamics, but few of them attempt to
construct models in which the reciprocal causality
between transformations in the space of artifacts and
organization in the space of agents plays an essential
role. Rather, most models assume that only artifacts
matter (for example, theories of technological
trajectories), whereas others are agent-centric, based
CONDITIONS FOR LONG LASTING SUSTAINABLE INNOVATION IN AN AGENT-BASED MODEL
411