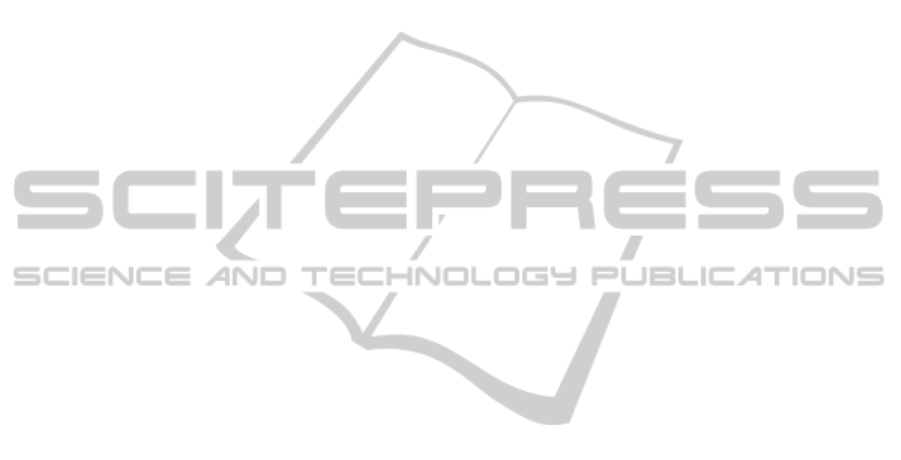
match in the second set, Orbb gets a better score
than any match from the first set.
Table 1 shows the average score and standard
deviation from the two sets of matches. Mynx's
score are not included as she scored 100 in every
match. The average score when Orbb uses opponent
modeling is twice that of when no modeling is used.
The standard deviation is also much higher, showing
that Orbb's scores are more variable when opponent
modeling is used.
Further tests were performed with more than two
competitors, however the results were excluded due
to space constraints. When more players compete in
a match the effect of opponent modeling was less
clear, but a small increase in performance was
regularly observed. There are many potential reasons
for only a small increase, such as more game time
needed to build models (more models need to be
built as there are more opponents), and less time
available to collect weapons and implement
strategies.
In addition to recording scores, we have
performed analysis on the generated models. This
analysis showed that a bot will fairly reliably
produce the same model for the same opponent, if
many games are played, each game starting with an
empty model. Analysis also showed that a bot will
produce different models for different opponents.
This is important as it shows the opponent models
do contain information derived from the particular
opponent.
5 CONCLUSIONS & FUTURE
WORK
These results show that opponent modeling, based
on techniques from student modeling, can provide
useful models of opponents in Quake 3. Now that
we have shown that useful information can be
obtained from such a model, more research can be
performed on how best to use such information. In
particular, the success value calculation could be
further refined. Future research will show us how to
best utilise model information, with the potential to
develop similar systems for other games.
The most practical benefit of this method of
opponent modeling seen in Quake 3 was in the
extreme cases. An opponent who was significantly
weaker than another player gained the most from
this enhancement. The models are useful for
highlighting very bad and very good decisions; if
this knowledge about the outcomes of decisions
against particular opponents is used to influence
each decision, the overall ability of the player is
improved. Although the models may not suggest the
best decision on every occasion, on average it can
improve the computer players’ performance, as
shown by the evaluation results.
This research has two aspects of significance.
One is the scale of the video games industry; this is
definitely big business, in terms of dollars and
influence. Additionally, the next generation of
educational games could benefit from utilising
similar opponent modeling techniques, to make the
game component of an educational game more
stimulating and challenging.
REFERENCES
Billings, D., Papp, D., Schaeffer, J., Szafron, D., 1998.
Opponent modeling in poker. Proceedings of the
fifteenth national/tenth conference on Artificial
Intelligence/Innovative applications of Artificial
Intelligence, p. 493-499.
Entertainment Software Association. 2010.
http://www.theesa.com/facts/salesandgenre.asp
Kobsa, A. 2001. Generic User Modeling Systems, User
Modeling and User-Adapted Interaction, p.49-63.
Mitrovic, A., Martin, B., 2007. Evaluating the Effect of
Open Student Models on Self-Assessment.
International Journal of Artificial Intelligence in
Education, Special issue on Open Learner Modeling,
p.121-144.
Mitrovic, A., Martin, B., Suraweera, P., 2007. Intelligent
tutors for all: Constraint-based modeling
methodology, systems and authoring. IEEE Intelligent
Systems, special issue on Intelligent Educational
Systems, p. 38-45.
Schadd, F., Bakkes, S., & Spronck, P., 2007. Opponent
modeling in real-time strategy games. In 8th
International Conference on Intelligent Games and
Simulation (GAME-ON 2007), M. Roccetti, Ed., p.
61-68.
Togelius, J., De Nardi, R., & Lucas, S. M., 2006. Making
racing fun through player modeling and track
evolution. Proceedings of the SAB Workshop on
Adaptive Approaches to Optimizing Player
Satisfaction.
Zanetti, S, Rhalibi, A., 2004. “Machine Learning
Techniques for First Person Shooter in Quake3”,
International Conference on Advances in Computer
Entertainment Technology.
Vygotsky, L., 1978. Mind in Society: The Development of
Higher Psychological Processes. Cambridge, MA:
Harvard University Press.
ICAART 2011 - 3rd International Conference on Agents and Artificial Intelligence
594