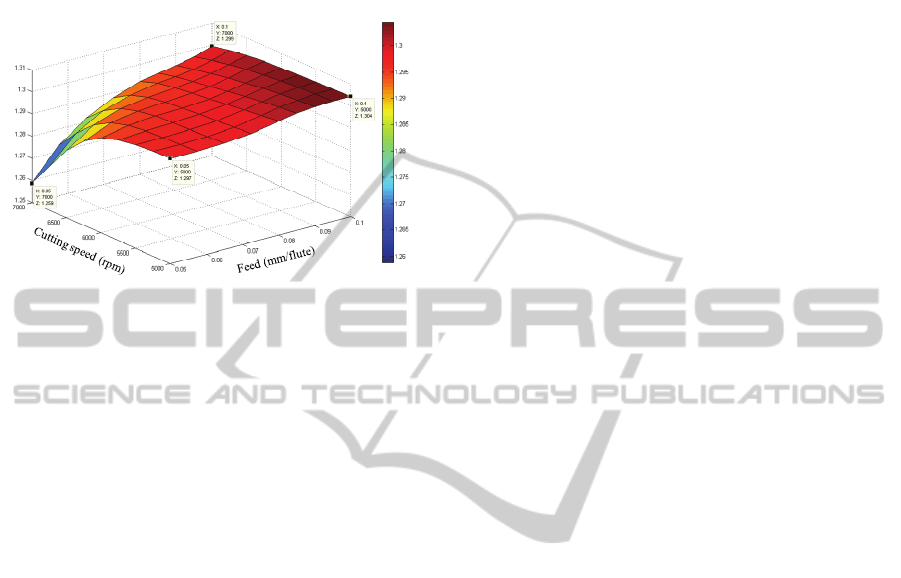
good correlation between output values and targets
during training (R=1), validation (R=0.89) and
testing procedure (R=0.93).
Ry (μm)
R
vs. Cuttin
speed & Feed
(Core diameter = 50 %, Flute angle = 38
o
, Rake angle = 22
o
, Relief angle 1
st
= 22
o
,
Relief angle 2
nd
= 30
o
, Cutting depth = 1,5 mm)
Figure 3: Response surface diagram of R
y
in relation to the
cutting speed and feed, while cutting depth is 1,5 mm.
The trained NN model can be used for the
optimization of the surface roughness parameters
during CNC end milling. This can be done by testing
the behaviour of the response variables (R
a
, R
y
and
R
z
) under different variations in the values of
geometry and cutting parameters. In order to ensure
accurate prediction of the surface roughness
parameters, the values concerning the eight input
parameters should be inside the range of values that
are defined during the experimental setup.
Figure 3 presents an example of a surface
response diagram for the roughness parameter R
y
while cutting speed and feed rate vary within their
range of values. In this diagram all the geometry
parameters were kept constant at their optimum
values. This figure shows that when the cutting
speed increases, as well as in the case of feed rate
reduction, the response variable (surface roughness,
R
y
) decreases.
5 CONCLUSIONS
A FFBP-NN model was built to estimate the surface
roughness indicator response according to the
geometry and cutting parameters of the process. The
performance of the network was found to be
efficient providing very good correlation between
outputs and targets during training (R=1), validation
(R=0.89) and testing procedure (R=0.93).
Furthermore, the response surface diagram in
Figure 3 shows that when the geometry parameters
take their optimum values, the increase of cutting
speed, as well as the decrease of feed rate, results in
deduction of the surface roughness, which is also in
accordance with the machining theory. Multi-
parameter investigation of the process according to
other quality indicators will be studied and analyzed
in future work.
ACKNOWLEDGEMENTS
In memory of George Petropoulos, Assistant
Professor in Machining Processes Technology,
Department of Mechanical & Industrial Engineering,
University of Thessaly, Volos, Greece.
REFERENCES
Chryssolouris, G., Pappas, M., Karabatsou, V., 2004.
Posture based discomfort modeling using neural
networks. Proceedings of IFAC MIM'04, Athens,
Greece, 19–23.
Engin, S., Altintas, Y., 2001. Mechanics and dynamics of
general milling cutters: Part I: helical end mills. Int. J.
Mach. Tools Manu. 41(15), 2195-2212.
Kechagias, J., Iakovakis, V., 2009. A neural network
solution for LOM process performance. Int. J.
Advanced Manufacturing Technology, 43(11–12),
1214–1222.
Kechagias J., Pappas, M., Karagiannis, S., Petropoulos,
G., Iakovakis, V., Maropoulos, S., 2010. An ANN
Approach on the Optimization of the Cutting
Parameters During CNC Plasma-Arc Cutting,
Proceedings of ASME ESDA 2010, Istanbul, Turkey.
Kechagias, J., Petropoulos, G., Iakovakis, V., Maropoulos,
S., 2009. An investigation of surface texture
parameters during turning of a reinforced polymer
composite using design of experiments and analysis.
Int. J. Experimental Design and Process Optimisation.
1(2/3), 164-177.
Markopoulos, A., et al., 2006. Artificial neural networks
modeling of surface finish in electro-discharge
machining of tool steels. Proceedings of ASME ESDA
2006, Torino, Italy.
Phadke, M. S., 1989. Quality Engineering using Robust
Design. Prentice-Hall, Englewood Cliffs, NJ.
Prechelt, L., 1998. Automatic early stopping using cross
validation: quantifying the criteria. Neural Networks,
11(4), 761–767.
Tzafestas, S. G., et al., 1996. On the overtraining
phenomenon of backpropagation NNs. Mathematics
and Computers in Simulation, 40, 507-521.
Yun, W. S., Cho, D. W., 2000. An improved method for
the determination of 3D cutting force coefficients and
runout parameters in end milling. Int. J. Adv. Manuf.
Technol., 16, 851–858.
ICAART 2011 - 3rd International Conference on Agents and Artificial Intelligence
598