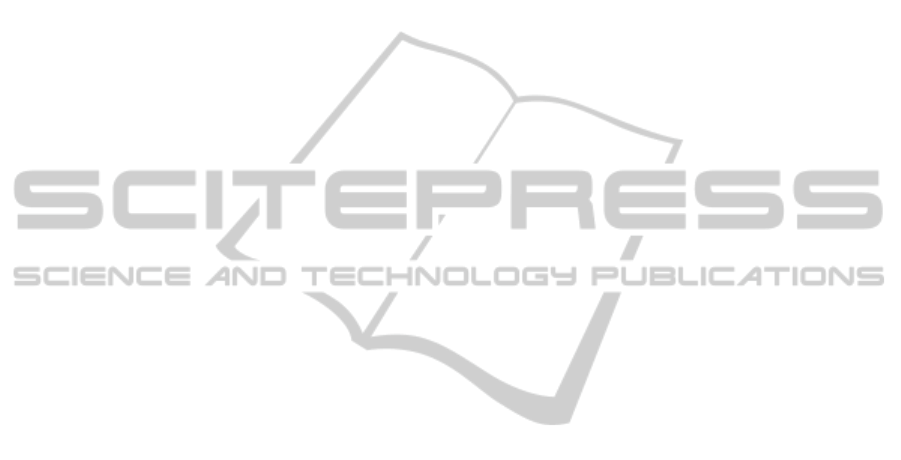
nition. Section 3 describes the different methods we
propose. Section 4 gives experimental results of our
methods. In the last section, we discuss the research
results and we propose some future research direc-
tions.
2 SHORT REVIEW OF FACE
RECOGNITION APPROACHES
Early face recognition approaches were based on
normalized error measures between significant face
points. One of the first method was designed by Bled-
soe (Bledsoe, 1966). Coordinates of important face
points were manually labelled and stored in the com-
puter. The feature vector was composed of the dis-
tances between these points. Vectors were classified
by the Nearest Neighbour rule. The main drawback
of such methods is the need of manually labelling of
important face points. On the other hand, variations
of the face pose, lighting conditions and other factors
can be handled due to this manual marking. Another
fully automatic method using similar measurements
was designed by Kanade (Kanade, 1977). In this case,
the labelling of important face points is automatic.
One of the first successful approaches is Prin-
cipal Component Analysis (PCA), so called Eigen-
faces (Turk and Pentland, 1991). Eigenfaces is a sta-
tistical method that takes into account the whole im-
age as a vector. Image vectors are put together and
create a matrix. Eigenvectors of this matrix are cal-
culated. Face images can then be expressed as a lin-
ear combination of these vectors. Each image is rep-
resented as a set of weights for corresponding vec-
tors. Eigenfaces perform very well when images are
well aligned and have approximately the same pose.
Changing lighting conditions, pose variations, scale
variations and other dissimilarities between images
decrease the recognition rate rapidly (Sirovich and
Kirby, 1987).
Another group of approaches use Neural Net-
works (NNs). Several NNs topologieswere proposed.
One of the best performing methods based on neu-
ral networks is presented in (Lawrence et al., 1997).
Image is first sampled into a set of vectors. Vectors
created from all labelled images are used as a train-
ing set for a Self Organizing Map (SOM). Image vec-
tors of the recognized face are used as an input of the
trained SOM. Output of the SOM is then used as an
input of the classification step, which is a convolu-
tional network. This network has a few layers and
ensures some amount of invariance to face pose and
scale.
A frequently discussed type of face recognition
algorithms is elastic bunch graph matching (Wiskott
et al., 1999; Bolme, 2003). This algorithm is based
on Gabor Wavelet filtering. Feature vectors are cre-
ated from Gabor filter responses as significant points
in the face image. Bunch graph is created and is
consequently matched against the presented images.
Another method which utilizes Gabor wavelets is the
method proposedby Kepenekci in (Kepenekci, 2001).
It uses wavelets in a different manner. Fiducial points
are not fixed. Their locations are assumed to be at the
maxima of Gabor filter responses. The main advan-
tage of Gabor wavelets is some amount of invariance
to lighting conditions.
3 METHODS DESCRIPTION
3.1 Average Eigenfaces
A classic Eigenfaces approach uses only one training
image. Our contribution is to adapt this method for
the case of more training examples being available.
We create one reference example from all training im-
age samples. In this preliminary study, we compute
from all training examples an average value of the in-
tensity at each pixel. These images are used for prin-
cipal component analysis.
3.2 SOM with a Gaussian Mixture
Model
Current face recognition methods are composed
of two steps: parametrization and classification.
Parametrization is used to reduce the size of the origi-
nal image with the minimal loss of discriminating in-
formation. The parametrized image is then used for
classification step instead of the original one.
We use self organizing maps in the parametriza-
tion step in order to reduce the size of the feature
vectors. The second step is a classification by the
Gaussian mixture model. The use of the SOM in the
parametrization is motivated by the work proposed
in (Lawrence et al., 1997). Authors use also SOMs
in the first step, while the classification model differs.
3.2.1 Parametrization with a SOM
Input images are represented as two dimensional ar-
rays of pixel intensities. We consider grayscale pic-
tures where each pixel is represented by a single in-
tensity value. Each image can be also seen as a sin-
gle dimensional vector of size w ∗ h, where w and h
are image width and height, respectively. For the di-
mension reduction a self organizing map is used. The
AUTOMATIC FACE RECOGNITION - Methods Improvement and Evaluation
605