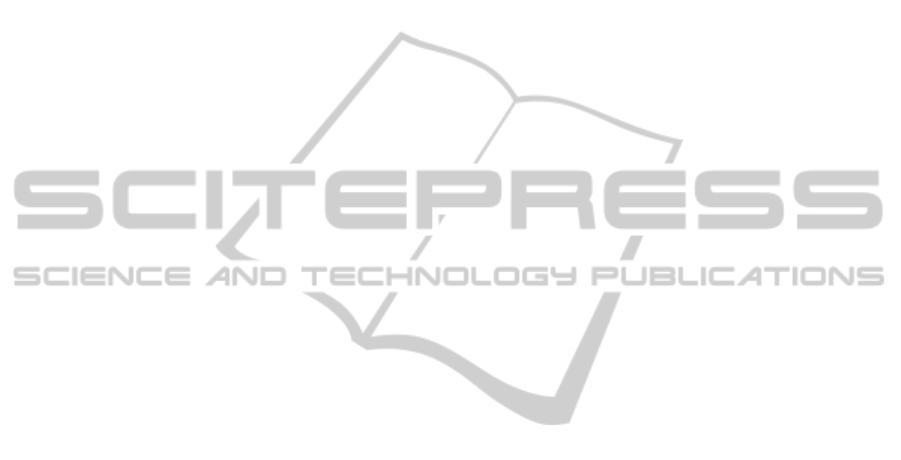
4 CONCLUSIONS
In this work we have presented a comparative study
of the use of variable selection in short-term wind
farm power prediction. The variable selection is done
with the Mutual Information that is estimated with the
method proposed by Kraskov. Two models are con-
sidered to study the effect of the variable selection.
One is a Multilayer Perceptron with different topolo-
gies and the other is the k-NN model for different val-
ues of the number of neighbors k.
Four different set of experiments were proposed
with different input and predicted variables. To assess
the quality of the results instead of the RMSE value,
the improvement of the RMSE over an improved per-
sistence model is used.
From the obtained results it can be concluded that
the k-NN model performs better than MLP model for
the different considered horizons and this is more em-
phasized as the number of neighbors increase. An in-
teresting conclusion is that the wind farm power pre-
diction is better done when power is used as predict-
ing variables instead of wind speed. Another fact that
the experiments has brought up and that it is in con-
sonance with the nature of the persistence model, it
is that as the horizon goes farther the MLP and k-NN
models yield better performance.
With respect to the introduction of a previous
stage of variable selection, in the experiments carried
out there is no evidence of a remarkable effect in the
results. In the MLP this effect is a bit noticeable when
predicting the wind farm power from previous mea-
sures of generated power and negligible in the k-NN
model.
ACKNOWLEDGEMENTS
This work has been partially supported by the Ca-
nary Islands government throught the project Sol-
SubC200801000137 and by the Spanish government
and FEDER through the project TIN2008-06068.
REFERENCES
Chow, T. W. S. and Huang, D. (2005). Estimating opti-
mal feature subsets using efficient estimation of high-
dimensional mutual information. IEEE Transactions
on Neural Networks, 16(1):213–224.
Costa, A., Crespo, A., Navarro, J., Lizcano, G., Madsen, H.,
and Feitosa, E. (2008). A review on the young history
of the wind power short-term prediction. Renewable
and Sustainable Energy Reviews, 12(6):1725–1744.
Crone, S. F. and Kourentzes, N. (2010). Feature selection
for time series predictiona combined filter and wrap-
per approach for neural networks. Neurocomputing,
73:1923–1936.
Focken, U., Lange, M., Monnich, K., Waldl, H., Beyer, H.,
and Luig, A. (2002). Short-term prediction of the
aggregated power output of wind farms - a statisti-
cal analysis of the reduction of the prediction error by
spatial smoothing. Journal of Wind Engineering and
Industrial Aerodynamics, 90:231–246.
Guill´en, A., Herrera, L., Rubio, G., Pomares, H., Lendasse,
A., and Rojas, I. (2010). New method for instance or
prototype selection using mutual information in time
seriesprediction. Neurocomputing, 73:2030–2038.
Ji, Y., Reyhani, N., and Lendasse, A. (2005). Direct
and recursive prediction of time series using mu-
tual information selection. In Computational Intelli-
gence and Bioinspired Systems (IWANN 2005), vol-
ume 3512/2005 of Lecture Notes in Computer Sci-
ence, pages 1010–1017.
Kraskov, A., Stgbauer, H., and Grassberger, P. (2004). Es-
timating mutual information. PHYSICAL REVIEW E,
69(6):066138.
Kusiak, A., Zheng, H., and Song, Z. (2009). Wind farm
power prediction: A data-mining approach. Wind En-
ergy, 12:275–293.
Landberg, L. (2001). Short-term prediction of local wind
conditions. Journal of Wind Engineering and Indus-
trial Aerodynamics, 89:235245.
Li, S., Wunsch, D. C., Ohair, E. A., and Giesselmann, M. G.
(2001). Using neural networks to estimate wind tur-
bine power generation. IEEE Transactions on Energy
Conversion, 16(3):276–282.
M´endez, J., Lorenzo, J., and Hern´andez, M. (2009). Ex-
periments and reference models in training neural net-
works for short-term wind power forecasting in elec-
tricity markets. In Bio-Inspired Systems: Computa-
tional and Ambient Intelligence, volume 5517/2009
of Lecture Notes in Computer Science, pages 1288–
1295.
MILCA (2010). Mutual information least-dependent com-
ponent analysis library. http://www.klab.caltech.edu/
∼kraskov/MILCA/. Last visited july, 2010.
Mohandes, M. A., Rehman, S., and Halawani, T. O. (1998).
A neural networks approach for wind speed predic-
tion. Renewable Energy, 13(3):345 – 354.
Nielsen, T. S., Joensen, A., Madsen, H., Landberg, L., and
Giebel, G. (1998). A new reference for wind power
forecasting. Wind Energy, 1(1):29–34.
S´anchez, I. (2006). Short-term prediction of wind en-
ergy production. International Journal of Forecasting,
22:43–56.
Yoon, H., Yang, K., and Shahabi, C. (2005). Feature sub-
set selection and feature ranking for multivariate time
series. IEEE Transactions on Knowledge and Data
Engineering, 17(9):1186–1198.
EXPERIMENTS IN SHORT-TERM WIND POWER PREDICTION USING VARIABLE SELECTION
375