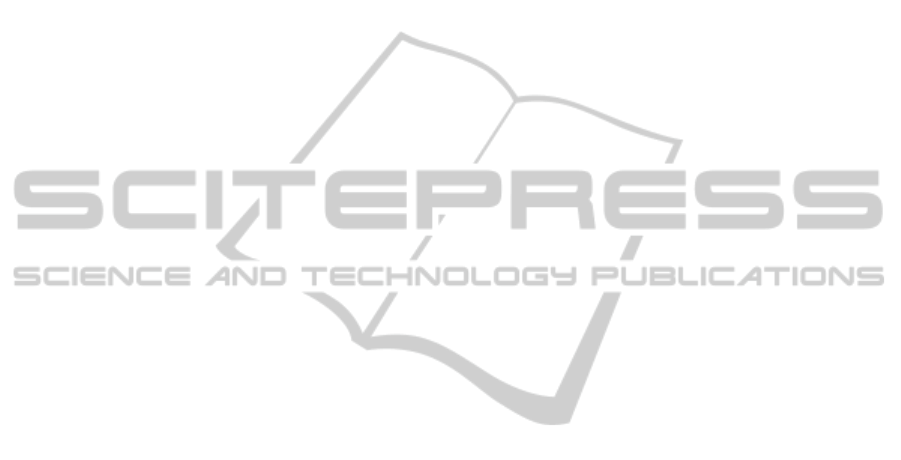
gression. As shown in Table 3, the principal com-
ponents with higher variance (i.e., PC7, PC8, PC2)
were dominant regressors; however the low-variance
principal components, such as PC93, PC71 and PC92,
were also important for estimation of HDS-R. Fi-
nally, we obtained the scatter plot shown in Fig. 4,
which suggests a positive linear relationship between
HDS-R and SPCIR. The results indicates a moder-
ately significant correlation (R = 0.73) between the
HDS-R score and the appropriate synthesis of sev-
eral selected prosodic features. Consequently, the ad-
justed coefficient of determination (
¯
R
2
= 0.57) sug-
gests that prosody-based speech sound analysis could
potentially be used to detect cognitive impairment in
elderly patients.
5 CONCLUSIONS AND FUTURE
WORK
Our study presented a novel approach to detect cog-
nitive impairment in elderly patients. This approach
uses prosody-based speech sound analysis and a mul-
tivariate statistical technique. As a clinical data exam-
ination, we collected 146 speech voice samples from
56 Japanese participants and extracted 98 prosodic
features from each of the samples. We then analyzed
the correlation between the HDS-R score and synthe-
sis of selected prosodic features by multiple linear re-
gression in combination with sophisticated feature se-
lection. We uncovered a moderately significant cor-
relation. Thus, this speech prosody-based approach
may be used to detect cognitive impairment in el-
derly patients. In future work, more expansive multi-
modality data collection will be performed using non-
invasive neurophysiological measurements such as
functional near-infrared spectroscopy (fNIRS). Much
more clinical trials will also be evaluated, and the
technique proposed here will be used as a screening
tool for dementia.
ACKNOWLEDGEMENTS
We are grateful to Dr. H. Endo and National Center
for Geriatrics and Gerontology for clinical assessment
and speech data collection. This work was supported
in part by SENTAN, Japan Science and Technology
Agency (JST), and part by Suzuken Memorial Foun-
dation.
REFERENCES
Akaike, H. (1974). A new look at the statistical model iden-
tification. IEEE Transactions on Automatic Control,
19(6):716–723.
Awata, S. (2009). Roll of the dementia medical center in
the community. In Japanese Journal of Geriatrics,
volume 46, pages 203–206. (in Japanese).
Buschke, H., Kuslansky, G., Katz, M., Stewart, W. F., Sli-
winski, M. J., Eckholdt, H. M., and Lipton, R. B.
(1999). Screening for dementia with the Memory Im-
pairment Screen. Neurology, 52(2):231–238.
Cho, J., Kato, S., and Itoh, H. (2009). Comparison of Sensi-
bilities of Japanese and Koreans in Recognizing Emo-
tions from Speech by using Bayesian Networks. In
IEEE International Conference on Systems, Man, and
Cybernetics, pages 2945–2950.
Cowie, R., Douglas-Cowie, E., Tsapatsoulis, N., Votsis,
G., Kollias, S., Fellenz, W., and Taylor, J. G. (2001).
Emotion recognition in human-computer interaction.
IEEE Signal Processing Magazine, 18(1):32–80.
Draper, N. and Smith, H. (1998). Applied Regression Anal-
ysis (3rd edition). John Wiley & Sons.
Folstein, M. F., Folstein, S. E., and McHugh, P. R. (1975).
“Mini-Mental State”: A practical method for grading
the cognitive state of patients for the clinician. J. Psy-
chiat. Res, 12(3):189–198.
Hoyte, K., Brownell, H., and Wingfield, A. (2009). Com-
ponents of Speech Prosody and their Use in Detection
of Syntactic Structure by Older Adults. Experimental
Aging Research, 35(1):129–151.
Imai, Y. and Hasegawa, K. (1994). The revised Hasegawa’s
Dementia Scale (HDS-R): evaluation of its usefulness
as a screening test for dementia. J. Hong Kong Coll.
Psychiatr., 4(SP2):20–24.
Massy, W. F. (1965). Principal Components Regression in
Exploratory Statistical Research. Journal of the Amer-
ican Statistical Association, 60(309):234–256.
Morris, J. C. (1993). The Clinical Dementia Rating
(CDR): Current version and scoring rules. Neurology,
43(11):2412–2414.
Scherer, K. R., Johnstone, T., and Klasmeyer, G. (2003).
Vocal expression of emotion. R. J. Davidson, H. Gold-
smith, K. R. Scherer eds., Handbook of the Affective
Sciences (pp. 433–456), Oxford University Press.
Sch¨olkopf, B., Smola, A., and M¨uller, K.-R. (1998). Non-
linear component analysis as a kernel eigenvalue prob-
lem. Neural Computation, 10(5):1299–1319.
Schwarz, G. E. (1978). Estimating the Dimension of a
Model. The Annals of Statistics, 6(2):461–464.
Taler, V., Baum, S. R., Chertkow, H., and Saumier, D.
(2008). Comprehension of grammatical and emo-
tional prosody is impaired in Alzheimer’s disease.
Neuropsychology, 22(2):188–195.
Taler, V. and Phillips, N. (2007). Language performance in
Alzheimer’s disease and mild cognitive impairment:
A comparative review. Journal of Clinical and Exper-
imental Neuropsychology, 30(5):501–556.
STATISTICAL ANALYSIS OF THE SIGNAL AND PROSODIC SIGN OF COGNITIVE IMPAIRMENT IN
ELDERLY-SPEECH - A Preliminary Study
327