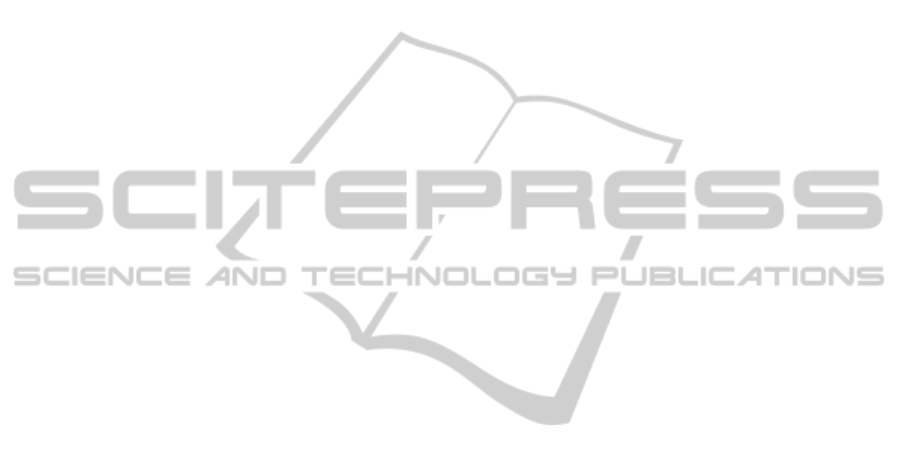
2 BAYESIAN NETWORKS
Bayesian networks (BN), also known as belief net-
works, belong to the probabilistic graphical models
family. These graphical structures are used for knowl-
edge representation of uncertain domains and when
they work with statistical techniques together, they
present several advantages for data analysis (Hecker-
man, 1996).
A formal definition of a BN is as follows. A
bayesian network model, or simply a bayesian net-
work, is a pair (D, P), where D is a directed acyclic
graph (DAG), P = {p(x
1
|π
1
), ..., p(x
n
|π
n
)} is a set of
n conditional probability distributions, one for each
variable, and Π
i
is the set of parents of node X
i
in D
(Castillo et al., 1997). The set P defines the associated
joint probability distribution as
p(x
1
, x
2
, ..., x
n
) =
n
∏
i=1
p(x
i
|π
i
) (1)
The construction of a bayesian network involves
the definition of its structure and the estimation of its
parameters. In the simplest case, the structure of a
bayesian network is specified by an expert and then
the corresponding parameters are learned from the
available data.
3 EXPERIMENTS
3.1 Experimental Design and Settings
In order to explore the performance of bayesian net-
works in the leukocytes classification problem, we de-
signed two models of this approach. That is, two ex-
periments for classifying all types (neutrophils, ba-
sophils, eosinophils, lymphocytes and monocytes) of
leukocytes were conducted. In the first experiment,
a bayesian network which includes some important
morphological features for leukocytes classification
was built. In the second experiment, we searched for
a simpler bayesian network with a better performance
than the one designed in the first experiment.
For the first experiment, the leukoA model was
developed. In the leukoA model, we proposed a
leukocyte classification node as the main one, and
with the purpose of expressing the real dependence
among features of leukocytes, we used a tree struc-
ture. In this model, we aimed to use some character-
istics that experts take into account for the classifica-
tion process. These features were incorporated into
the model as discrete latent variables. Furthermore,
for the bayesian network structure building we placed
some observable nodes (which are linked to the la-
tent variables) representing the description or mea-
surements of the corresponding features (see Figure
1). These measurements were obtained by applica-
tion of digital image processing techniques. The ob-
servable nodes are continuous variables that have a
normal distribution. The description of the incorpo-
rated knowledge into the leukoA model is presented
as follows.
The first characteristic considered into the leukoA
model was the shape of the nucleus. The nucleus
shape of lymphocytes is round, and the monocytes
shape have a great reniform or horseshoe-shaped nu-
cleus. The nucleus of neutrophils have from 2 to 5
lobules, it can present S, C or glass shapes. The nu-
cleus of eosinophils have 2 lobules and usually it is
glass shaped. The nucleus of basophils is bi- or tri-
lobed, but it is hard to see because of the number of
granules which hide it (Carr and Rodak, 2004; Greer
et al., 2009; Estridge et al., 1999). This knowledge
about the shape of nucleus was encoded into the nu-
cleus shape node. The estimation of this shape was
obtained by means of region descriptors, particularly,
we used the compactness, dispersion and the first Hu
moment (Nixon and Aguado, 2007). These descrip-
tors were included into the leukoA model as compact-
ness, dispersion and MH1 nodes.
Since nucleus size is more relevantthan cytoplasm
size for leukocytes identification, only the nucleus
size was considered for the leukoA model. For the
nucleus size measurement we took the number of pix-
els that belong to the corresponding region divided by
the total number of pixels of the cell (nucleus and cy-
toplasm pixels). This nucleus size information was
included into the nucleus size node, which was linked
with the nucleus shape node due to the relationship
between these two features.
The cytoplasm texture is an important characteris-
tic of leukocytes, it allows to group the cells by the
presence or absence of granules in their cytoplasm
(Greer et al., 2009). The granulocyte type cells are
neutrophils, basophils and eosinophils. The agranu-
locyte cells are lymphocytes and monocytes. In order
to get information about the cytoplasm texture, the en-
ergy descriptor (Nixon and Aguado, 2007) was used.
This knowledge about the cytoplasm texture and its
corresponding descriptor were captured with the cy-
toplasm texture and energyC nodes.
The texture of nucleus is another important char-
acteristic of leukocytes that is reported in medical
literature (Greer et al., 2009; Estridge et al., 1999).
For this reason, we included this knowledge into the
leukoA model in a similar way as the cytoplasm tex-
ture was.
ICAART 2011 - 3rd International Conference on Agents and Artificial Intelligence
682