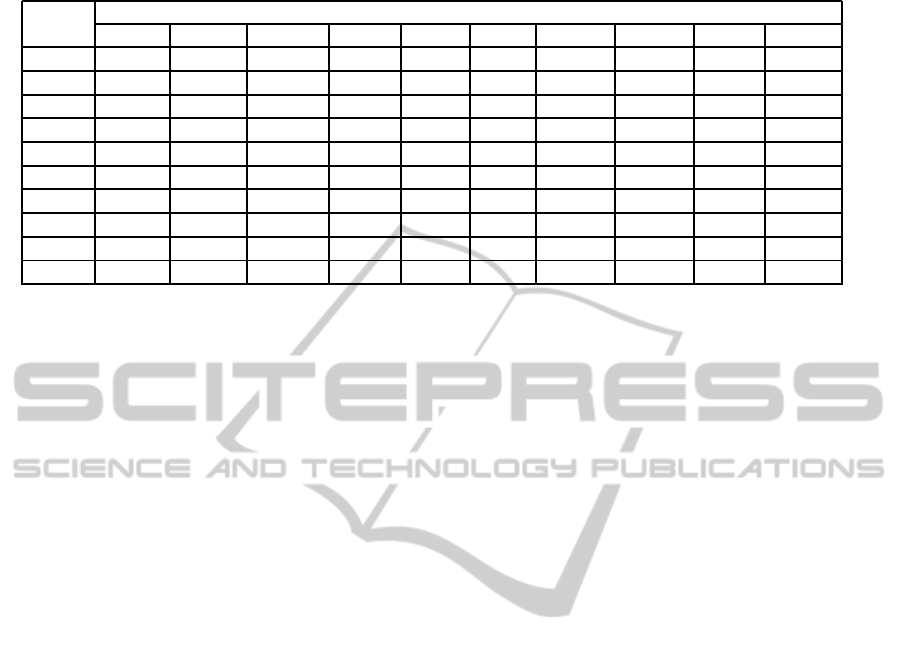
Table 6: The distance matrix between the images.
The distance between the images in node number
One Two Three Four Five Six Seven Eight Nine Ten
One 0 31 42 34 47 29 56 48 49 51
Two 31 0 43 35 48 30 57 49 50 52
Three 42 43 0 46 59 41 68 60 61 63
Four 34 35 46 0 51 33 60 52 53 55
Five 47 48 49 51 0 46 73 65 66 68
Six 29 30 41 33 46 0 55 47 48 50
Seven 56 57 68 60 73 55 0 74 75 77
Eight 48 49 60 52 65 47 74 0 67 69
Nine 49 50 61 53 66 48 75 67 0 70
Ten 51 52 63 55 68 50 77 69 70 0
REFERENCES
Abrahams, I. W. and Gregerson, D. S. (1983). Longitudinal
study of serum antibody responses to bovine retinal s-
antigen in endogenous granulomatous uveitis. British
Journal of Ophthalmology, 67:681–684.
Bhuiyan, A. (2009). Automated modeling of retinal vas-
cular network. PhD Thesis, The University of Mel-
bourne.
Bhuiyan, A., Nath, B., Chua, J., and Kotagiri, R. (2007a).
Blood vessel segmentation from color retinal images
using unsupervised classification. In the proceedings
of the IEEE International Conference of Image Pro-
cessing, pages 521–524.
Bhuiyan, A., Nath, B., Chua, J., and Ramamohanarao, K.
(2007b). Automatic detection of vascular bifurcations
and crossovers from color retinal fundus images. Pro-
ceedings of Third International IEEE Conference on
Signal-Image Technologies and Internet-Based Sys-
tem (SITIS), pages 711–718.
Bhuiyan, A., Nath, B., Chua, J., and Ramamohanarao, K.
(2008). Vessel cross-sectional diameter measurement
on color retinal image. Communications in Computer
and Information Science, 25:214–227.
Deng, K., Tian, J., Zheng, J., Zhang, X., Dai, X., and Xu,
M. (2010). Retinal fundus image registration via vas-
cular structure graphmatching. International Journal
of Biomedical Imaging, pages 1–13.
Gonzalez, R. C. and Woods, R. E. (2008). Digital Image
Processing. Pearson Prentice Hall, third edition.
Guo, X., Hsu, W., Lee, M. L., and Wong, T. Y. (2006). A
tree matching approach for the temporal registration
of retinal images. Proceedings of IEEE International
Conference on Tools with Artificial Intelligence, pages
632–642.
Harris, A. J. and Yen, D. C. (2002). Biometric authenti-
cation: assuring access to information. Information
Management and Computer Security, 10(1):12–19.
Hughes, A. D., Stanton, A. V., Jabbar, A. S., Chapman, N.,
Martinez-Perez, M. E., and Thom, S. A. (2008). Effect
of antihypertensive treatment on retinal microvascu-
lar changes in hypertension. Journal of Hypertension,
26:1703–1707.
Knuth, D. E. (1973). The art of computer programming.
Addison-Wesley Pub. Co., 2nd edition edition.
Lin, K.-S., Tsai, C.-L., and Sofka, M. (2009). Vascular tree
construction with anatomical realism for retinal im-
ages. Proceedings of Ninth IEEE International Con-
ference on Bioinformatics and Bioengineering, pages
313–318.
Marino, C., Penedo, M. G., Penas, M., Carreira, M. J., and
Gonzalez, F. (2006). Personal authentication using
digital retinal images. Pattern Analysis Application,
9:21–33.
Stanford, M. R., Graham, E., Kasp, E., Sanders, M. D., and
Dumonde, D. C. (1988). A longitudinal study of clin-
ical and immunological findings in 52 patients with
relapsing retinal vasculitis. British Journal of Oph-
thalmology, 72:442–447.
Taarnhoj, N. C. B. B., Munch, I. C., Sander, B., Kessel, L.,
Hougaard, J. L., and Kyvik, K. (2008). Straight ver-
sus tortuous retinal arteries in relation to blood pres-
sure and genetics. British Journal of Ophthalmology,
92:1055–1060.
Womack, M. (1994). The eyes have it. Sensor Review,
14(4):15–16.
Wong, T. Y., Kamineni, A., Klein, R., Sharrett, A. R.,
Klein, B. E., Siscovick, D. S., Cushman, M., and Dun-
can, B. B. (2006). Quantitative retinal venular caliber
and risk of cardiovascular disease in older persons.
Archives of Internal Medicine, 266:2388–2394.
Wong, T. Y., Klein, R., Klein, B. E. K., Meuer, S. M.,
and Hubbard, L. D. (2003). Retinal vessel diame-
ters and their associations with age and blood pres-
sure. Investigative Ophthalmology and Visual Science,
44(11):4644–4650.
AN AUTOMATED METHOD FOR RETINAL IMAGE MATCHING USING VASCULAR FEATURES
347